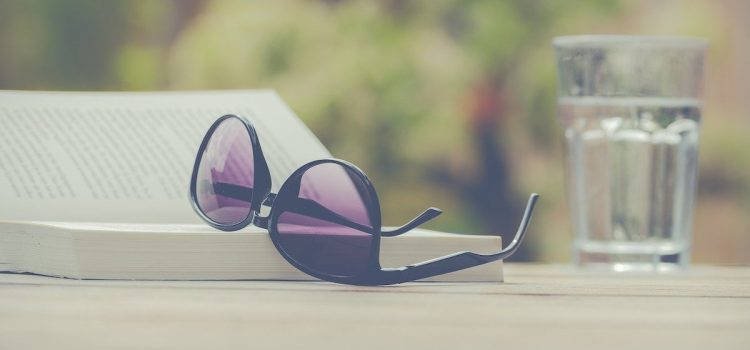
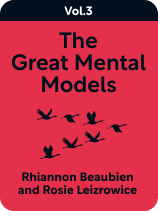
This article is an excerpt from the Shortform book guide to "The Great Mental Models Volume 3" by Rhiannon Beaubien and Rosie Leizrowice. Shortform has the world's best summaries and analyses of books you should be reading.
Like this article? Sign up for a free trial here .
Would you like to sharpen your thinking and decision-making? What practical lessons can you learn from models such as critical mass, algorithms, and randomness?
The Great Mental Models Volume 3: Systems and Mathematics is the third in a series of books designed to improve your thinking by giving you a set of models that you can use to better understand the world. The premise of the series is that the world operates according to specific rules and patterns (“models”) that occur again and again in many different contexts. Volume 3 draws its models from systems science and mathematics—fields rich in concepts that help explain human behavior and give us a more objective and accurate perspective on life.
Read more to learn about these models and how you can apply them to your life in a practical way.
The Great Mental Models Volume 3: Systems and Mathematics
The Great Mental Models book series builds on a list of mental models first published on Farnham Street, a website and blog dedicated to helping people learn from the best ideas available. The authors suggest that, by internalizing these models, you can build your understanding and improve your decision-making since you’ll have a starting point every time you encounter a new situation.
The Great Mental Models Volume 3: Systems and Mathematics is written by Rhiannon Beaubien and Rosie Leizrowice. Beaubien is the managing editor of Farnham Street and the lead writer for the entire Mental Models book series, and Leizrowice is a former content strategist at Farnham Street. The book is aimed at readers who want to improve their thinking and decision-making and who are interested in learning from a variety of fields.
In the book, the models are divided into systems and mathematics, and each chapter focuses on one model. The models can be organized around five general themes:
- The basic components of human behavior
- Group dynamics
- How systems grow and why they lose efficiency
- How and why to think long term
- How to think statistically for a more accurate perspective on life
Part 1: Understanding Behavior
A lot of the ideas in The Great Mental Models Volume 3 are concerned with understanding behavior. In fact, when the authors talk about systems, they are most interested in how systems explain behavior. In this section, we’ll look at how systems shape our behaviors and explore some factors to keep in mind if your goal is behavior change.
Feedback Loops
According to Beaubien and Leizrowice, many human behaviors are based on feedback loops—effects that occur whenever a system’s output affects the system’s future behaviors. The authors point out that there are two types of feedback loops: balancing and reinforcing:
- A balancing loop occurs when the output causes a change in behavior.
- A reinforcing loop occurs when the output encourages repeat behavior.
The authors argue that, in addition to shaping individual behaviors, balancing and reinforcing feedback loops interact to create many of our social institutions, which in turn form a feedback loop with society at large. They give the example of the justice system, in which criminal judgments and penalties provide clear feedback on certain types of behaviors.
Behavior Change
If you understand feedback loops, you’ll have a better chance of changing your behaviors if that’s your goal. Until you see the connection between a behavior and its long-term feedback, you can’t act on the feedback. Because of the potential gap between behavior and feedback, the authors say that, if you want to change a behavior, you should anticipate future consequences by analyzing any feedback loops that might be in play.
Algorithms
Not all behaviors are driven by feedback loops. The authors point out that some behaviors in a system can be described as algorithms. An algorithm is a set of simple steps (like a recipe) that turns input into output in a consistent, predictable way each time. Algorithms are valuable because they’re consistent and repeatable, and they replace the need to make decisions about the same things over and over.
Like feedback loops, algorithms explain more than just individual behavior—Beaubien and Leizrowice point out that social structures like laws and constitutions also function as algorithms by providing clear if-then relationships between behaviors and consequences. In the criminal justice system, for example, there are a set of rules specifying what punishments might be applied if you steal.
Part 2: Group Dynamics
We’ve seen how feedback loops and algorithms help explain both individual and group behavior. But one of the interesting things about systems is that groups tend to develop behaviors and qualities that aren’t present in any of the individuals that make up the group. In this section, we’ll look at several models of group dynamics that help us understand how groups create value based on their size, how social change works, and how turnover can both help and hurt a system.
Network Effects
One way that groups create meaning or value that isn’t present in the individual is through what’s called network effects. The authors explain that network effects happen when something increases in value or utility the more people have it or use it. For example, a social networking app is valuable only if it has a reasonably large user base.
Critical Mass
Network effects show that you need a certain number of people to make certain products or services worthwhile. A broader version of this principle is the concept of critical mass—the idea that you need a certain number of people to think alike in order to effect social change. The authors explain that, more generally, a system is said to be critical when it’s right at its tipping point and about to change from one state to another. Critical mass is important because it helps you focus your efforts where they’re most needed.
Churn
So far, we’ve been looking at the power of getting more people together and on the same page. But when you’re looking at group dynamics, you also have to think about the rate at which people leave the group. Beaubien and Leizrowice explain that managing churn—the inevitable attrition that happens over time—is key to keeping a group functioning at its best.
In a social system, churn is governed by the rate at which new members join a group and the rate at which existing members leave. The authors argue that, in any social context, churn is necessary for innovation.
Part 3: Growth and Efficiency
Now that we’ve seen the kinds of behaviors that emerge as systems grow, let’s look at some models for understanding how systems grow in the first place as well as how and why they break down. The fundamental idea in this section is that systems are nonlinear, which means there’s not always a 1:1 correlation between changes in input and changes in output.
Bottlenecks
The authors point out that one of the difficulties in growing a system is that growth inevitably creates bottlenecks, which are the slowest parts of the system. A bottleneck can be:
- A physical limitation or choke point
- An inefficiency in your methods
- A resource shortage
In each of these cases, the bottleneck serves as a limiting factor—it either slows the system down or it limits how much output the system can create.
Diminishing Returns
When you run into a bottleneck, you’ll discover that adding more input doesn’t always increase output—a phenomenon known as diminishing returns. Beaubien and Leizrowice say that the model of diminishing returns describes any situation in which input increases output only up to a point: Past that point, more input has less and less effect.
Part 4: Long-Term Thinking
As we’ve seen, if you’re looking to maintain or grow a system, it pays to plan ahead. To help you do so, this section focuses on several models that encourage effective long-term thinking. The central theme of this section is that being open-minded and broadening your knowledge can lead to big gains down the road. These gains might be material or they might come in the form of enhanced creativity or greater preparedness for the unknown.
Compounding
One major reason to think long term is that doing so lets you capitalize on the effects of compounding. The authors explain that investments of money, knowledge, and effort compound over time, leading to exponential (rather than linear) gains.
Surface Area
Even when it’s happening, sometimes compounding isn’t obvious because there’s no way to know what gains await you down the line. For this reason, the authors recommend that you increase your surface area by exposing yourself to as many new ideas and experiences as possible.
So how do you increase your surface area? The authors recommend being curious and open to new things. They also recommend cultivating your relationships.
Part 5: Looking at the Big Picture
As you work through any of the models we’ve discussed so far, you’ll stand the best chance of success if you have an accurate view of the world. Unfortunately, because each of us has a limited perspective, sometimes it’s hard to see the big picture—and easy to misinterpret some situations as a result. This final section introduces several models drawn from mathematics that can help us see the big picture more clearly. The basic theme of this section is that, by thinking statistically, you’ll have a clearer context for the things you encounter.
Distributions
Beaubien and Leizrowice argue that, in order to have accurate contexts for information, you need to understand probability distributions. In statistics, a distribution describes how likely different results are in a given data set. A major takeaway from this discussion of distributions is that you always need to look for the greater context for information, especially when you’re dealing with figures and statistics. You also need to remember that our intuitive notions about things like averages can sometimes lead us astray.
Randomness
Whereas distributions can help you understand the patterns behind facts or events, the model of randomness can help prevent thinking errors by reminding you that sometimes there isn’t a pattern to be found. Beaubien and Leizrowice argue that most of what happens does so by chance.
The idea that the universe is mostly random might seem scary at first, but Beaubien and Leizrowice point out that recognizing randomness has numerous benefits, starting with better decision-making. Understanding randomness also helps you avoid making faulty predictions.
Another lesson we can learn from randomness is that the past doesn’t always predict the future. According to Beaubien and Leizrowice, the principle of regression to the mean suggests that unusual results are most likely to be followed by ordinary results rather than by additional unusual results.
One final reason Beaubien and Leizrowice say we should embrace randomness is that randomness is at the heart of creativity.
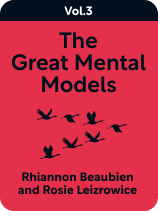
———End of Preview———
Like what you just read? Read the rest of the world's best book summary and analysis of Rhiannon Beaubien and Rosie Leizrowice's "The Great Mental Models Volume 3" at Shortform .
Here's what you'll find in our full The Great Mental Models Volume 3 summary :
- How to understand anything in the world by learning a finite set of rules and patterns
- A look at how humans are driven by algorithms
- Why you shouldn't be fooled by randomness