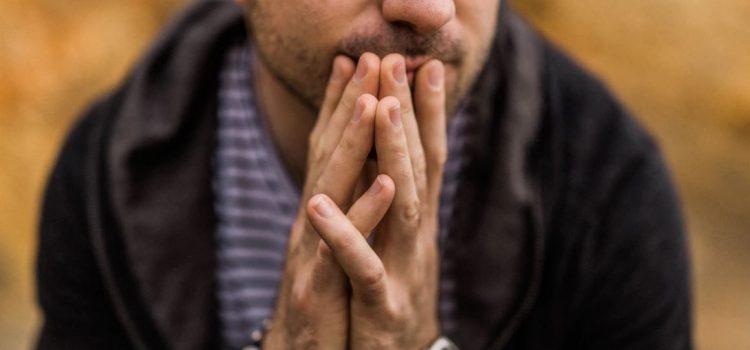
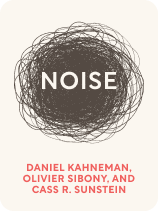
This article is an excerpt from the Shortform book guide to "Noise" by Daniel Kahneman, Olivier Sibony, and Cass Sunstein. Shortform has the world's best summaries and analyses of books you should be reading.
Like this article? Sign up for a free trial here .
Do you want to know how to improve decision-making? Are mechanical judgment tools better than human judgment?
In the book Noise, the authors explain that one way to improve human judgments is actually to eliminate humans from the judgment process. Human judgments are full of noise and bias, which can be removed and replaced with statistical models or computer algorithms.
Here’s how to improve decision-making by removing noise.
How to Reduce or Eliminate Noise
Want to know how to improve decision-making? The authors of Noise offer a few solutions, including mechanical judgment tools (models and algorithms) that can replace or augment human judgment as well as a set of suggestions for reducing noise in human decision-making.
Detecting and Measuring Noise
Typically, the first step in reducing noise is figuring out how much noise there is in the first place. This step is necessary because administrators tend to believe that their organizations make judgments consistently, and until they can see the problem firsthand, they may be resistant to change.
To determine how much noise is present in a company, organization, or system, the authors outline a noise audit process they use when consulting with businesses. The book includes an appendix with detailed guidelines for conducting a noise audit. The general gist is that an organization would give a set of sample cases to all of its members whose job it is to make judgments about such cases. For example, an insurance company would give a set of sample claims to all of its adjusters. The judgers being audited complete their judgments independently, and then the results are compared to see how much variability there is throughout the organization.
Mechanical Judgments
Once noise has been detected, there are several options for reducing it. One option is to remove human judgment from the equation altogether. To do so, decision-making can be handled via statistical models or computer algorithms.
Though they touch on several mechanical judgment methods (which we’ll explain briefly below), the authors are more interested in reducing noise in human judgment rather than replacing human judgment with mechanical judgment. This is in part because, as the authors point out, mechanical predictions currently can’t do anything humans can’t do—they just do it with better predictive accuracy. The authors argue that this improved accuracy mostly results from the elimination of noise, and so we might see the efficacy of mechanical judgments more as a demonstration of the benefits of noise reduction rather than as a blanket solution to the problem of noise.
(Shortform Note: Although the authors come down in favor of improving rather than replacing human judgments, they perhaps don’t make this point as clearly as they could, given the way some reviewers focus on the dangers of algorithms as a major criticism of the book’s recommendations. Indeed, the authors spend a lot of time explaining models and algorithms and defending them from potential criticism, which perhaps creates a misleading impression of how central they are to Noise’s proposed course of action. To keep the focus on ways to improve human judgment, we’ve kept the following discussion of models and algorithms brief and to the point.)
Statistical Models
One way to make predictions is by using a statistical model. A statistical model is a formula that uses weighted variables to calculate the probability of an outcome. For example, you could build a statistical model that predicts the likelihood of a student graduating college by assigning weights to factors like high school GPA, SAT scores, number of extracurricular activities, whether the student’s parents graduated college, and so on.
Studies have shown that simple statistical models that apply weighted averages of relevant variables consistently outperform human predictive judgments. In fact, the authors provide studies suggesting that any statistical model, whether carefully crafted or cobbled together at random, can predict outcomes better than humans can.
The authors argue that this superior performance is simply because statistical models (and by extension, algorithms) eliminate noise. Even the crudest or most arbitrary model has the advantage of being consistent in every single case. And while human judgers can weigh subtle subjective factors that a model can’t take into account, the authors suggest that this subjectivity tends to add more noise than predictive clarity. As we saw earlier, we’re not very good at recognizing which factors are relevant to our predictions.
Computer Algorithms
Another more recent and more complex form of mechanical judgment is the computer algorithm. The authors explain that computer algorithms build on the basic idea of statistical modeling, but they also come with additional benefits that improve their accuracy. Because they take into account massive data sets and can be programmed to learn from their own analysis, algorithms can detect patterns that humans cannot. These patterns can form new rules that improve the accuracy of the judgments.
The authors acknowledge that algorithms are not perfect—and that if they are trained using data that reflects human bias, they will reproduce that bias. For example, if an algorithm built to predict criminal recidivism is built from a data set that reflects racial biases in the justice system, the algorithm will perpetuate those racial biases. (Shortform note: For example, after years of development, Amazon discovered that its recruitment algorithm systematically favored men over women. Likewise, Facebook’s advertising algorithms have come under fire for helping to spread everything from fake news to hate speech.)
Combining Mechanical and Human Judgment Because the authors are most interested in finding ways to improve human judgment, they don’t give much attention to the option of combining human and mechanical judgment. This hybrid approach has real-life precedents and may sometimes be the best way to tackle a problem. For example, after the success of Michael Lewis’s Moneyball, some baseball teams began favoring rigorous statistical analysis over traditional scouting when deciding which players to acquire. At the time, there wasn’t a great statistical way to measure players’ fielding skills, so some teams neglected defense in favor of more easily measured offensive skills. In practice, these teams gave up so many runs that they offset the benefits of their new statistical approach. In more recent years, most teams have adopted statistical modeling techniques, but the most successful teams have combined these models with old-fashioned human scouting. This hybrid approach works because scouts can account for things that models can’t, such as the mental factors needed to succeed in professional baseball. Baseball provides a counterargument to Noise’s cautions against human subjectivity. But the key here is that teams have learned how to combine human and mechanical judgments in ways that maximize the strengths and minimize the weaknesses of each. |
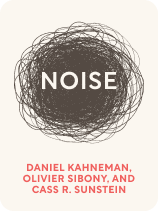
———End of Preview———
Like what you just read? Read the rest of the world's best book summary and analysis of Daniel Kahneman, Olivier Sibony, and Cass Sunstein's "Noise" at Shortform .
Here's what you'll find in our full Noise summary :
- A deep dive into the unexpected and unwanted variance in human judgments
- How to reduce or eliminate noise from your decision-making
- How to practice good decision hygiene