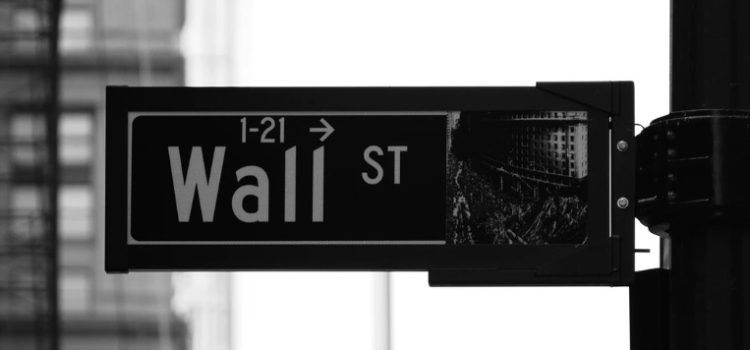
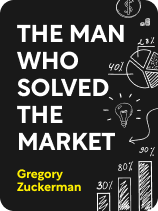
This article is an excerpt from the Shortform book guide to "The Man Who Solved the Market" by Gregory Zuckerman. Shortform has the world's best summaries and analyses of books you should be reading.
Like this article? Sign up for a free trial here.
When was the Renaissance hedge fund launched? How did Jim Simons attract mathematicians and investors to the fund?
After careers in codebreaking and academia, Jim Simons turned to a life of finance in 1978. He launched Monometrics in the same year, which was later renamed Renaissance.
Learn more about Simons’s journey to the successful Renaissance hedge fund.
Launching Renaissance
At 30 years old with a wife and children, writes Gregory Zuckerman in The Man Who Solved the Market, Simons still saw himself fundamentally as a mathematician and academic who thrived in the company of other similarly minded individuals. We’ll explore how Simons transitioned from an intellectually minded academic to a wildly successful and wealthy captain of finance. Specifically, we’ll look at his time as a mathematics chair on Long Island in the 1970s; his founding of the Renaissance hedge fund in 1978; and the extraordinary, market-beating growth of Renaissance in the 1980s.
Mathematics Chair at Stony Brook
In 1968, Simons took a job as the mathematics chair at the State University of New York at Stony Brook, on Long Island, where he stayed until 1978. There, his connections and keen eye for talent helped him build a first-rate mathematics department, transforming Stony Brook from a little-known public university into a mathematics powerhouse that could hold its own with Ivy League universities. While at Stony Brook, Simons continued his academic work, publishing groundbreaking mathematics papers that cemented his reputation as a leading pure mathematician of his time.
Importantly, writes Zuckerman, Simons also recruited many scholars he’d known at Berkeley to Stony Brook, as well as former IDA colleagues. Many of these hires would in turn become colleagues and investors at Simon’s future hedge fund, Renaissance.
(Shortform note: Simons left a lasting impact on Stony Brook’s reputation and academic standing. According to US News and World Report, in 2023, Stony Brook ranked #30 in the United States for graduate mathematics, ahead of Ivy League universities like Dartmouth. And, although he hasn’t worked for the university in an official capacity for decades, Simons still maintains strong ties with the university: In June 2023, Simons and his wife Marilyn gave $500 million through their Simons Foundation to Stony Brook’s endowment, support scholarships, professorships, research, and clinical care.)
Seeking a New Challenge
In 1978, Simons shocked his friends when he announced his intention to leave academia and start his own fund. Despite his success and popularity at Stony Brook, Simons never quite lost the itch for investing he’d developed as a younger man—whether it had been experimenting with futures contracts in the early 1960s or building mathematical trading models with his IDA colleagues. The rush, the thrill, the attraction of using his quantitative abilities and elite standing within the mathematics world to make money seemed like a way to put pure mathematics into action.
“Mathiness” and the (Mis)use of Numbers Some in the economics field have decried what they see as the appropriation of advanced mathematics for profit, as Simons did in his career, or to advance ideological agendas. In 2015, economist Paul Romer coined the term “mathiness” to describe what he saw as the misuse of mathematics in economics research. He decried the employment of complicated mathematics to mislead audiences and obscure authors’ true agendas—specifically how statistics and economic models are used in politics. For example, a politician might campaign on a platform of lowering tax rates while claiming, seemingly paradoxically, that the lower rates will increase government revenue. She may justify this position by pointing to tables and graphs, which lend the appearance of mathematical rigor. This is then used to show that the lower rates will boost productivity across the economy and thus enable the government to collect more overall revenue, even at the lower rate. But the tables and graphs obscure the fact that the economic growth and revenue projections they purport to show are purely speculative and based on the most optimistic reading of the data. |
Finding Signals in the Noise
Simons launched his fund in 1978. It sported an atypical look for what would become one of the most successful hedge funds of all time—far from the glittering towers of Wall Street and the glamor of Manhattan, Simons started his operation out of a strip mall on Long Island. And the shabbily dressed, scruffy, academic Simons hardly looked the part of a finance power player.
The fund, initially named Monometrics (the name was changed to Renaissance in 1982), built on the investment model Simons had begun experimenting with back in his IDA days. It focused on buying or selling currencies at the right time based on the model’s predictions of when they were most likely to rise or fall in value—predictions derived from quantitative analysis and statistical modeling of historical price data.
It was, as Zuckerman writes, the logical extension of Simons’s work as a mathematician and codebreaker: finding patterns, structure, signals, and meaning in the seemingly random data. Based on the condition or phase of the market at a given time, the model could create a probability distribution mapping out the likelihood of different sets of subsequent outcomes. From this, the model could assess which assets or commodities were likely to increase in value over a certain period of time and which were likely to decrease in value.
Alfred Winslow Jones and the Invention of the Hedge Fund Although Simons and his team were certainly early hedge fund pioneers—and among its most successful practitioners—this form of investing had existed for decades. In 1949, Australian investor Alfred Winslow Jones started what many scholars call the world’s first hedge fund. Like Simons, Jones came from a non-financial background (in fact, he’d even been a spy like Simons), yet he was able to perceive insights that eluded traditional investors. Jones argued for a systems-based approach to investing that foreshadowed the hedge fund strategies that investors like Simons, George Soros, and Ray Dalio honed decades later. Jones’s strategy was to create a market-neutral portfolio—in other words, one that would be “hedged” against the volatility of the market. He achieved this by buying securities his model predicted would outperform the market, and short-selling (effectively betting against) securities he believed would sell below market value. Like Simons later, his strategy was predicated upon buying and selling the right mix of assets at the right times, rather than allowing his portfolio to simply rise or fall with the fortunes of the market. And Jones’s “hedged” strategy paid off handsomely: A 1966 article in Fortune showed that Jones’s fund had outperformed the top-performing mutual fund by 44% over the preceding five years. |
Early Success
Zuckerman writes that, within a few years, the mathematical modeling strategy paid off for Simons: Although still flying largely under the radar, the fund attracted clients and investors, growing by tens of millions of dollars. Simons used rigorous mathematical modeling and the nascent power of computing to test and refine his models as they absorbed new data—the building blocks of what, decades later, would be called machine learning. And in this effort, he tapped the minds of his mathematician colleagues—people like Lenny Baum, who built the original fund algorithm that directed the fund to buy or sell certain assets based on observed price movements; and James Ax, who helped build the fund’s computer trading system that enabled the firm to execute those timely trades.
The Rise of Computers The timing of the rise of Simons’s fund in the 1970s and 1980s coincided with the extraordinary, exponential growth of computer processing power during this time. The principle behind this growth is known as Moore’s law, which states that the speed and capabilities of computer chips double every two years due to chipmakers’ increasing ability to fit more transistors on semiconductor chips. Since its promulgation in 1965, Moore’s law has largely held true. Simons and his team successfully rode the computer processing wave as they were getting Renaissance off the ground, with computing power increasing a trillion-fold from 1956 to 2015. And it wasn’t just the finance industry that computing power was revolutionizing. One study of labor and residential data from 1970 to 2000 shows a significant shift in the skills required for new jobs across all industries after 1980—with this shift beginning at the same time that the personal computer became ubiquitous. Computer-related jobs became the most in-demand and computer-related industries saw the fastest labor growth. By 2000, all the fastest-growing jobs were related to computers. |
Increasing the Trade Volume
By the mid-1980s, Simons and Renaissance were getting closer to a truly automated trading system due to cheaper and more powerful computers and access to new microdata. These enabled the fund to capitalize on intraday trading fluctuations, broken down by hours and minutes, which optimized the algorithms and made them more robust. And these intraday fluctuations pointed to the need for the fund to massively increase its volume of daily trading to capitalize on these short-term movements—to make the model agile and responsive enough to buy and sell assets within minutes or seconds.
Zuckerman writes that Simons also believed more frequent trading would reduce risk: With more trades, there would be less risk associated with each individual trade. The negative effects of a “bad” trade would be minimized, since the model didn’t need to “win” every trade to make a profit—it just needed to win the majority of them.
The key to the strategy’s success was learning to trust the model’s predictions based on the computer’s analytics of historical data. It wasn’t necessary to know anything substantive about the underlying bonds, commodities, or currencies being traded or why their prices fluctuated the way they did: What mattered was the integrity of the data and the reliability of the patterns identified by the algorithm. If the algorithm could detect a non-random pattern and make bets that paid off more often than not, the fund would invest even if it followed no apparent economic logic.
(Shortform note: Simons’s focus on short-term price movements presaged the rise of what would become known as high-frequency trading (HFT). In Flash Boys, Michael Lewis describes HFT as a type of electronic trading platform that uses automated computer algorithms to quickly buy and sell large quantities of stocks. These HFT algorithms gather market data and use this information to buy and sell stocks, completing this process in microseconds. This was a very different strategy from the time-honored method used by traditional mutual funds, which typically buy a large, diversified bundle of stocks and hold on to them for the long term. The speed advantage of the HFT algorithms enables them to accrue billions of dollars in tiny profits from trades that traditional investors and traders can’t capitalize on.)
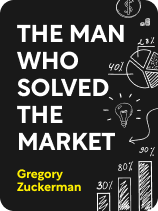
———End of Preview———
Like what you just read? Read the rest of the world's best book summary and analysis of Gregory Zuckerman's "The Man Who Solved the Market" at Shortform.
Here's what you'll find in our full The Man Who Solved the Market summary:
- The story of Jim Simons, one of the most successful hedge fund managers ever
- How Simons' experience as a codebreaker helped him succeed
- How Simons set the stage for today's large-scale, automated trading