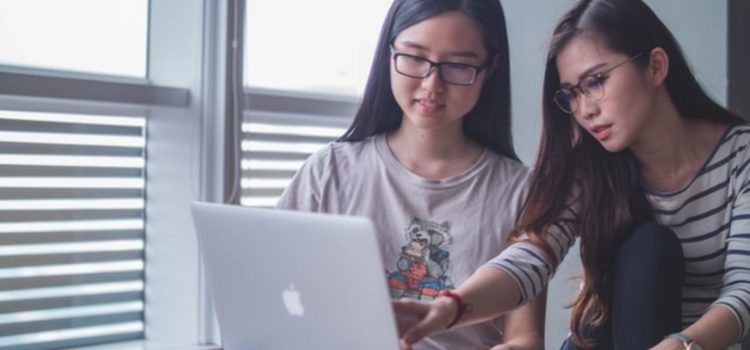
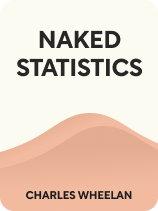
This article is an excerpt from the Shortform book guide to "Naked Statistics" by Charles Wheelan. Shortform has the world's best summaries and analyses of books you should be reading.
Like this article? Sign up for a free trial here .
What is regression analysis in statistics? What can a regression test tell us about the relationship between two variables?
Regression analysis is an inferential statistic that can help us infer relationships between variables that we wouldn’t otherwise be able to study. Regression analysis quantifies the direction, magnitude, and significance of an independent variable’s relationship to a dependent variable.
Here’s a look at what inferential analysis does and the statistics involved.
Regression Analysis Basics
What is regression analysis in statistics? Regression analysis illuminates the relationship between an independent variable and a dependent variable. According to Wheelan, we can think of the independent variable as the “event” we’re interested in and the dependent variable as the associated outcome. In the chemical exposure and cancer example, your independent variable is chemical exposure, and your dependent variable is cancer rates.
(Wheelan notes that no matter how close the relationship between independent and dependent variables is, regression analysis can only illuminate relationships. It can’t determine causation. Therefore, we use terminology like “association” between an independent and dependent variable rather than saying that the independent variable “causes” a change in the dependent variable.)
As with other inferential statistics, regression analysis begins with a null hypothesis that you’ll either accept or reject at a specified confidence level. The null hypothesis in this example is that “Exposure to chemical X is not associated with an increased risk of cancer.” Say you set your confidence at .05, meaning you want to be at least 95% sure when accepting or rejecting the null hypothesis. Next, you collect data from a large, random sample of people who were exposed to the chemical and compare their cancer rates to the cancer rates of the general population.
To learn about the process and statistics involved, we’ll carry this example through each step of regression analysis.
Confounding Variables There is a third category of variable, confounding variables, that researchers would often prefer not to have in their experiments. Confounding variables obscure or confound (hence the terminology) the relationship between the independent and dependent variables and, therefore, the validity of regression analyses. Since confounding variables can impact the dependent variable, the independent variable, and the relationship between the independent and dependent variables, it can be difficult to distinguish exactly which relationship the regression analysis is analyzing. For example, say you were interested in studying the relationship between excessive sugar consumption and subsequent meltdowns in children. A confounding variable might be the fact that oversized sweet treats are often a part of special occasions, which also naturally drain children’s energy and can lead to post-fun meltdowns. Therefore, without further analysis, you can’t be sure whether the sugar or the excitement precipitated the meltdown. |
The Regression Coefficient and Line of Best Fit
As Wheelan explains, when we plot our independent and dependent variables in a scatter plot, we can often infer their relationship at a glance. (Note: The independent variable is plotted on the horizontal axis, and the dependent variable is plotted on the vertical axis.) Below is a scatter plot for a hypothetical dataset comparing cancer rates and exposure to chemical X. Without doing any statistics, we can see that as chemical exposure increases, cancer rates also increase.
Regression analysis quantifies this relationship by finding a line of best fit for a scatter plot of our data.
The line of best fit doesn’t actually go through many (if not most) of our data points, but instead is a line that minimizes the total distance between itself and all of the data points, hence the term “best fit.”
The slope of the line of best fit is represented by the regression coefficient. The regression coefficient tells us the direction of the relationship (positive or negative), and by how much a change in the independent variable predicts a change in the dependent variable.
For example, say your regression coefficient for cancer rates and chemical exposure was +2. The positive sign tells you that an increase in exposure is associated with an increase in cancer, and the number two tells you that for every unit that chemical exposure increases, the risk of cancer increases by two units.
Once we know the regression equation, we can use it to calculate specific values. For example, you could calculate the cancer risk at one, five, or 10 “units” of chemical exposure.
Regression Analysis for Smoking and Lung Cancer The above example linking cancer risk and chemical X is of course hypothetical. However, researchers have enough data on smoking and cancer rates to make the sort of predictions outlined above. For example, a study in the National Library of Medicine used regression analysis to calculate that for every additional 1% that American adults smoke (collectively), lung cancer rates rise by 164 cases per 1,000 citizens. It’s important to remember that the regression equation represents a line of “best” fit, meaning that it’s set up to minimize the distance between the line and all collective data points. Therefore, when using a regression equation to measure an outcome like lung cancer, we can’t expect the regression equation to give us a perfect prediction for our individual risk. Our personal risk of developing cancer could be higher or lower than a regression equation predicts, sometimes by a great deal. For example, plenty of heavy smokers never get lung cancer, and plenty of non-smokers do. Just as descriptive statistics can’t encompass the nuance of the original dataset, a regression equation based on population-level data can’t encompass the complexity of an individual (although we will discuss how to get closer predictions with multivariate regression analysis below). |
The R2 Statistic
Regression analysis goes a step further than quantifying the association between independent and dependent variables. Thanks to a statistic called the R2 statistic, regression analysis can tell us how much of the change in our dependent variable is explained by changes in our independent variable. In our chemical exposure example, for instance, R2 can tell you how much of a person’s overall cancer risk is determined by their exposure to chemical X, and how much is due to other factors such as smoking, diet, exercise, genetics, and so on.
R2 is reported as a value between zero and one and interpreted as a percent. A value of zero means that our regression equation can’t predict our dependent variable at all, and a value of 1 means that it can predict 100% of the variation in our dependent variable.
In the cancer risk example, if your R2 for chemical exposure was .08, then 8% of a person’s overall cancer risk would be explained by their exposure to the chemical, and 92% would be due to other factors.
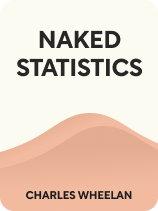
———End of Preview———
Like what you just read? Read the rest of the world's best book summary and analysis of Charles Wheelan's "Naked Statistics" at Shortform .
Here's what you'll find in our full Naked Statistics summary :
- An explanation and breakdown of statistics into digestible terms
- How statistics can inform collective decision-making
- Why learning statistics is an exercise in self-empowerment