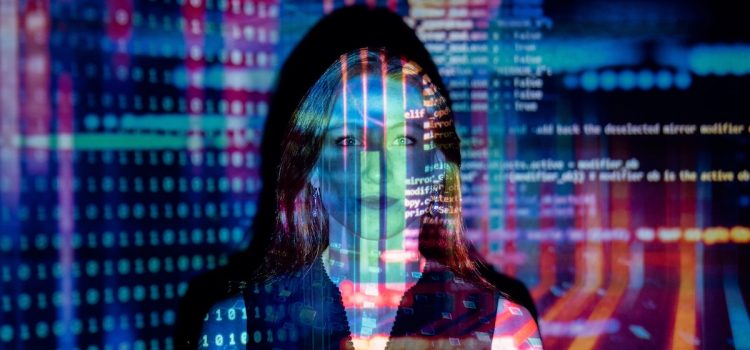
What’s big data? How helpful is training data? What can make it problematic?
Professors Carl T. Bergstrom and Jevin D. West are so concerned about misinformation that they wrote a book about it. In Calling Bullshit, they argue that big data can foster bullshit because it can incorporate poor training data and find illusory connections by chance.
Continue reading to learn about a problem with big data that should have everyone’s attention.
The Bullshit Problem With Big Data
Big data refers to a technological discipline that deals with exceptionally large and complex data sets using advanced analytics. Bergstrom and West explain how big data generates computer programs. They relate that researchers input an enormous amount of labeled training data into an initial learning algorithm.
For instance, if they were using big data to create a program that could accurately guess people’s ages from pictures, they would feed the learning algorithm pictures of people that included their age. Then, by establishing connections between these training data, the learning algorithm generates a new program for predicting people’s ages. If all goes well, this program will be able to correctly assess new test data—in this case, unfamiliar pictures of people whose ages it attempts to predict. But, a major problem with big data can arise when training data is flawed.
(Shortform note: ChatGPT, a chatbot launched by OpenAI in November 2022, is itself a byproduct of big-data-fueled machine learning, as it processed an immense amount of training text to create coherent sequences of words in response to test data (inquiries from users). The widespread success of ChatGPT—and other related large language models—suggest that although Bergstrom and West may be correct that big data can propagate bullshit, it can also create revolutionary forms of artificial intelligence whose impact is felt worldwide.)
Bergstrom and West argue that flawed training data can lead to bullshit programs. For example, imagine that we used big data to develop a program that allegedly can predict someone’s socioeconomic status based on their facial structures, using profile pictures from Facebook as our training data. One reason why this training data could be flawed is that people from higher socioeconomic backgrounds typically own better cameras and thus have higher-resolution profile pictures. Thus, our program might not be directly identifying socioeconomic status but rather camera resolution. In turn, when exposed to training data not sourced from Facebook, the big data program would likely fail to distinguish between socioeconomic status.
(Shortform note: These bullshit programs can perpetuate discrimination in the real world, as illustrated by Amazon’s applicant-evaluation tool that consistently discriminated against women in 2018. As training data, Amazon had exposed AI to resumés from overwhelmingly male candidates in the past, leading its AI program to display prejudice towards male applicants in the test data—that is, when reviewing current applicants’ resumés. For instance, it punished resumés that even included the term “women,” as in “women’s health group,” and it learned to discredit applicants from certain all-women’s universities.)
In addition, Bergstrom and West point out that, when given enough training data, these big data programs will often find chance connections that don’t apply to test data. For instance, imagine that we created a big data program that aimed to predict the presidential election based on the frequency of certain keywords in Facebook posts. Given enough Facebook posts, chance connections between certain terms may appear to predict election outcomes. For example, it’s possible that posts including “Tom Brady” have historically predicted Republican victories, just because the Patriots have happened to win on the verge of Republican presidential elections.
(Shortform note: One way to identify chance connections versus genuine causal connections is to seek out confounding variables—a third factor that explains the chance connection between two variables. For example, the number of master’s degrees issued and box office revenues have been tightly correlated since the early 1900s, but this correlation is likely due to a third factor—population growth—that is driving increases in both master’s degrees and box office revenues.)