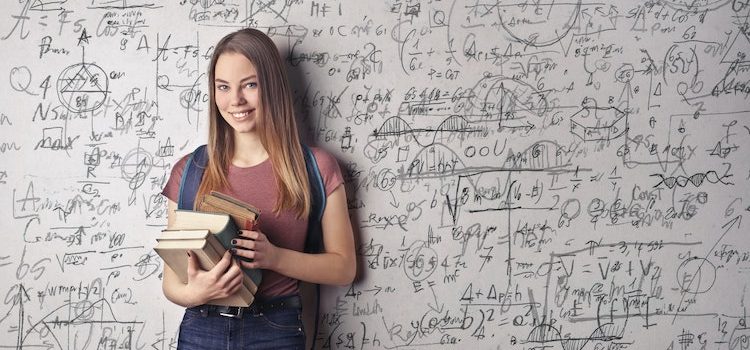
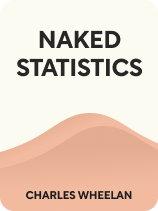
This article is an excerpt from the Shortform book guide to "Naked Statistics" by Charles Wheelan. Shortform has the world's best summaries and analyses of books you should be reading.
Like this article? Sign up for a free trial here .
What is inferential analysis? What can inferential statistics tell us about data?
In simple terms, inferential statistics are a kind of combination of data and probability. Just as probability is never a guarantee of an outcome, there are no definitive answers in inferential statistics. Rather, inferential statistics help us use what we do know to make math-based best guesses about what we want to know.
Keep reading for the ultimate guide to inferential statistics.
Understanding Inferential Statistics
What is inferential analysis in statistics? Inferential analysis enable us to extrapolate beyond the data we collect and make inferences about how the world works. However, inferential statistics can’t actually prove anything by themselves because they are based exclusively on numerical data that can’t capture the complexity of the real world. In other words, inferential statistics give us a compelling reason to believe that two variables are related but don’t supply a mechanism for that relationship.
For example, say you’re researching the effectiveness of a stream clean-up program. Your regression analysis suggests a strong positive relationship between clean-up efforts and stream biodiversity, from which you infer (hence the terminology) that the program is working. However, you would still need to know why and how it is working. For example, perhaps your efforts to curb thermal pollution from a wastewater treatment plant upstream have led to a higher level of dissolved oxygen in the water, which has allowed more species to survive. Taken together, your understanding of the mechanism (heat from the wastewater treatment plant heats the water, and warm water holds less dissolved oxygen than cooler water) and your statistical analyses make a strong causal case for the clean-up program’s impact on water quality.
Next, we’ll look at some of the most basic terms and concepts of inferential statistics.
Hypothesis Testing
Inferential statistics test hypotheses, which are educated guesses about how the world works. Based on our statistical analyses, we can either accept these hypotheses as true or reject them as false with varying degrees of certainty.
There are common conventions around testing a hypothesis with inferential statistics. We’ll give a general overview of some of these conventions and apply them to an example in the following sections.
The Null and Alternative Hypotheses
When we use inferential statistics to answer a question, we begin with a null hypothesis. A null hypothesis assumes a relationship between two variables that we’ll accept or reject. Wheelan explains that the convention is to begin with a null hypothesis that we hope or expect to reject. For example, a vitamin company might hope to reject the null hypothesis that absorption of their new vitamin is no better than absorption of their previous formula.
Rejecting a null hypothesis in inferential statistics means “accepting” an alternative hypothesis. The alternative hypothesis is the logical inverse of the null hypothesis. If the vitamin company’s null hypothesis is that absorption of their new vitamin is no better than absorption of their previous formula, their alternative hypothesis is that absorption of their new vitamin is better than absorption of their previous formula.
Communicating Confidence in Statistics
Since there are no definitive answers in inferential statistics, we can never accept or reject a null hypothesis with complete certainty. Instead, we accept or reject a null hypothesis with a specified degree of confidence, known as the confidence level.
The person running the statistical test sets the confidence level. Wheelan explains that commonly used confidence levels are .05, .01, and .1, but researchers can set their confidence level wherever they choose.
Confidence levels represent the uncertainty that remains when we reject the null hypothesis. So at a .05 confidence level, we can be 95% confident in rejecting the null hypothesis (.05 as a percent is 5%, and 100-5 gives us 95%). At a .01 confidence level, we can be 99% confident in rejecting the null hypothesis.
In statistics, when we reject a null hypothesis, we accept the alternative hypothesis as (probably) true. For example, if the vitamin company rejects their null hypothesis at a .05 confidence level, it means they are 95% certain that the alternative hypothesis—that their new formula has better absorption than their previous formula—is true.
Type l and Type ll Errors
Wheelan explains that another way to think about confidence levels is as the “burden of proof” that you’re putting on your statistical analyses. While a high burden of proof might seem intuitively desirable, there are trade-offs to consider when selecting confidence levels for statistical analyses. To understand these trade-offs, we need to understand Type I and Type II errors.
Type I errors are false positives, which means we reject a null hypothesis that is actually true (and accept an alternative hypothesis that is actually false). For example, if the absorption of our vitamin company’s new formula wasn’t any better than the previous formula, but their study led them to conclude that it was, they would be making a Type l error.
Type lI errors are false negatives, which means we accept a null hypothesis that is actually false (and reject an alternative hypothesis that is actually true). For example, if the absorption of our vitamin company’s new formula was better than the previous formula, but their study led them to conclude that it wasn’t, they would be making a Type ll error.
Setting your burden of proof (confidence level) high, say, .001, makes it statistically more difficult to reject the null hypothesis because, at that level, you have to be 99.9% certain the alternative hypothesis is true before you can reject the null. This makes you more likely to make a Type ll error by accepting the null hypothesis as true when it’s not.
In contrast, setting your confidence level lower, say, .1, reduces the burden of proof necessary to reject the null hypothesis because you only need to be 90% certain the alternative hypothesis is true before you can reject the null. This makes you more likely to make a Type l error by rejecting a null hypothesis that is actually true (and accepting an alternative hypothesis that is actually false).
Since you can never fully eliminate the possibility of making Type l and Type ll errors, the circumstances around your research and statistical analysis will determine which one you are more willing to accept.
For example, as a medical researcher working on a new vaccine, you might have a very low tolerance for rejecting a true null hypothesis (Type l error). Since people’s health is at stake, you want to be very sure that your vaccine works. Therefore, you might opt for a high confidence level, say, .001, meaning that you are 99.9% confident when you reject the null hypothesis that your vaccine is not effective against a certain malady. In this case, by opting for such a high level of confidence, you increase your chances of making a Type ll error and concluding that your vaccine is not effective when, in fact, it is.
In contrast, you might be more inclined to accept a Type l error as a social sciences researcher looking to implement a recreational therapy program at a local senior center. You might even set your confidence level at .25, meaning that you would be 75% confident in rejecting the null hypothesis that your recreation program does not produce clinically significant results. In this case, your rationale might be that even if the results of the program are not clinically measurable, the community as a whole will still enjoy it, and there is little risk of doing harm.
Statistical Significance
The results of statistical analyses are often reported as being “statistically significant” or “not statistically significant.” When results are statistically significant, it means that you can be reasonably certain that your observed results are due to the variable you are measuring and not to random chance.
Statistical significance is often reported with a statistic called the p-value. The p-value pinpoints how likely your results are given a true null hypothesis. In other words, it tells you the likelihood of getting your results if the variable you’re measuring really has no effect and chance alone influenced the data.
A small p-value adds confidence to rejecting the null hypothesis. When the p-value is less than your established confidence level, you can report your results as statistically significant.
For example, say our vitamin company chooses a .05 confidence level for their statistical analysis, meaning that they want to be 95% confident when they reject the null hypothesis and move forward with the new formula. Their statistical analysis shows a p-value of .01. This means that there is a 1% chance that their results are due to random chance, and the null hypothesis is true (in other words, there is a 99% chance that these results are not just due to chance). Since this p-value falls within their 95% confidence level for rejecting the null hypothesis, they report their results as “statistically significant.”
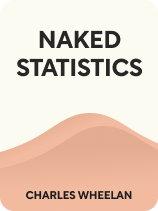
———End of Preview———
Like what you just read? Read the rest of the world's best book summary and analysis of Charles Wheelan's "Naked Statistics" at Shortform .
Here's what you'll find in our full Naked Statistics summary :
- An explanation and breakdown of statistics into digestible terms
- How statistics can inform collective decision-making
- Why learning statistics is an exercise in self-empowerment