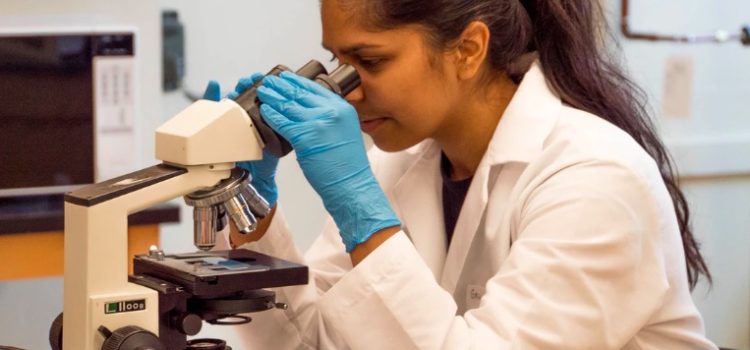
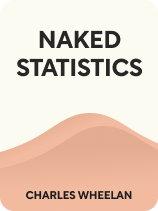
This article is an excerpt from the Shortform book guide to "Naked Statistics" by Charles Wheelan. Shortform has the world's best summaries and analyses of books you should be reading.
Like this article? Sign up for a free trial here .
What is program evaluation research? What types of program evaluations are there?
Program evaluation refers to any situation where we’re interested in measuring the outcome of an event, which we refer to as a “treatment.” “Treatments” encompass academic interventions, social programs, political policies, fitness regimens, business tactics, clinical trials, and so on.
Keep reading to learn about program evaluation research design and major challenges.
Program Evaluation Study Design
One of the most fundamental challenges in program evaluation research is establishing treatment and control groups. If we want to know how well a program worked or the impact that an experience had, then we need to be able to compare the outcomes of individuals who participated in the program (the treatment group) to the outcomes of those who did not (the control group).
Many factors can make establishing treatment and control groups challenging for a particular research project. We’re often interested in collecting data on “treatments” which we expect will make people’s lives better. Therefore, withholding treatment from a “control group” for the sake of experimentation may not be appropriate. In contrast, we’re also often interested in studying the effects of a “treatment” that we expect may be harmful; smoking, for instance. To purposefully expose a control group to a harmful intervention for data collection purposes would be considered unethical.
Using Animals as Treatment and Control Groups One way medical researchers navigate the challenges of establishing treatment and control groups is by using animals as research subjects. Much of the research on drugs and medical treatments is done on animals, particularly rodents. Since rodents are biologically similar to people, but with much shorter lifespans, researchers are able to study the effect of an intervention or exposure over an individual’s entire lifetime, or even over the course of several generations. In addition, federal law currently mandates that treatments be tested on animals before they are tested on humans in order to ensure safety for human treatment groups in clinical trials. However, animal rights advocates and others opposed to animal testing use statistics to argue that the benefits of testing on animals are outweighed by the costs. According to the National Institutes of Health, up to 95% of the attempts to bring a new drug to market fail; but before they do they can cost over $1 billion, take over a decade, and cause death and suffering for countless animals. Even in instances where drugs prove successful in animals, they often fail to work the same way in humans. For example, PETA notes that there have been 85 HIV-prevention drugs that have worked for primates but not for people. In light of results like these, the British Medical Journal notes that there may not be enough evidence to support our reliance on animal testing; especially since, as Wheelan notes, many (if not most) studies with negative findings go unreported. This is not to say that experiments with negative findings are a failure, since negative findings can be just as informative as positive ones. However, it does raise the issue of whether the time and resources spent on animal studies that never make their way to human trials could be better spent on other research methods that don’t cause animals pain. |
Data Collection Strategies
Particularly in the social sciences, clear-cut treatment and control groups are not always readily apparent or available for a given research question, and researchers often have to be flexible and creative. Wheelan highlights the following strategies researchers use to establish treatment and control groups when a highly controlled research environment is unavailable:
Natural Experiments: Wheelan explains that researchers often look for natural experiments that establish treatment and control groups for them. For example, say researchers were interested in studying the link between being struck by lightning and negative long-term health consequences. Clearly, researchers won’t establish a “treatment” group by purposefully exposing people to a lightning strike. Instead, they’ll likely look for individuals who have been struck by lightning in the past and compare their health to comparable individuals who haven’t been struck by lightning.
The Coronavirus Pandemic as an Opportunity for Data Collection The coronavirus pandemic has created opportunities for natural experiments around the world. Scientists are using the disruption in people’s daily lives to collect data on the effects of remote learning, the reduction in personal mobility on the atmosphere, the impacts of the fishing industry on shark populations, and many other topics. Maternal and fetal medicine is one area where researchers and medical professionals are eager to collect data during the pandemic. In wealthy nations, the medical community has noted a large drop in premature births during the pandemic, whereas in poorer nations premature birth rates have increased. Research into this trend is ongoing, but one possible explanation is that mothers in wealthy countries experienced cleaner air and less exposure to infections while in lockdown, while mothers in poorer countries experienced more exposure to indoor air pollution as well as more stress due to economic strain. |
Differences in Differences: In a similar fashion to natural experiments, Wheelan explains that researchers often look for a retroactive control group to measure the effects of a treatment. This situation arises when researchers are interested in the outcome of a treatment, but everyone in their current population experienced that treatment, leaving them without a control group. To address this problem, researchers often look for another population that experienced different circumstances over the same time period and use outcomes from that population as a control.
A hypothetical example of this scenario would be a governor interested in the effect of a mask mandate in her county on disease transmission during the coronavirus pandemic. Since all of the residents in her county were part of the mask “treatment” group, she might look for the most comparable county that did not instate a mask mandate and compare transmission rates between the two.
Keeping Groups Constant in Differences-in-Differences As Wheelan explains, the differences-in-differences research model affords researchers an opportunity to collect data in instances where a controlled experiment wouldn’t be possible. As with natural experiments, many (if not all) of the research subjects in a differences-in-differences model may be unaware that their data is being used for research. In our above mask-mandate example, for instance, unless the study was published and you read it, you would have no idea that your coronavirus test results had become part of a research study. The flexibility of the differences-in-differences model also presents a challenge for research validity because for the data to be reliable, the two groups must remain constant throughout the study period. If people don’t even know that they’re part of a research study, however, they won’t be monitoring their behavior to maintain the study’s validity. Continuing with the mask mandate example, say one of the towns that data was being collected on is a seasonal town. Halfway through the study period, there is a huge influx of seasonal residents. The composition of the town is now different than it was at the start of the experiment, so the results of the study lose reliability. |
Discontinuity Analysis: Sometimes, researchers can establish treatment and control groups with people who “barely made the cut” and vice versa for a treatment.
For example, say a very dedicated parent and statistician was interested in the impact of attending a prestigious soccer camp on children’s future success in the sport. That parent could use a group of children whose skills barely qualified them for the camp as the treatment group and a group of children who just barely did not qualify for the camp as the control group.
Discontinuity analysis could also be appropriate in measuring outcomes for children whose families barely qualified for programs like SNAP and Medicaid and those whose families barely didn’t qualify.
Benefits of Discontinuity Analysis While Wheelan presents discontinuity analysis as a compromise when other research methods aren’t available, there are benefits of this type of study: – Since the study is done on the very population and at the very threshold of existing policies and programs, the results of the study will be directly applicable to the real-world scenario researchers are interested in. – Since the programs analyzed with discontinuity analysis are already running, discontinuity analysis can be a much more affordable research scenario than designing and implementing a study from scratch. |
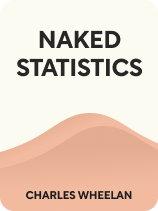
———End of Preview———
Like what you just read? Read the rest of the world's best book summary and analysis of Charles Wheelan's "Naked Statistics" at Shortform .
Here's what you'll find in our full Naked Statistics summary :
- An explanation and breakdown of statistics into digestible terms
- How statistics can inform collective decision-making
- Why learning statistics is an exercise in self-empowerment