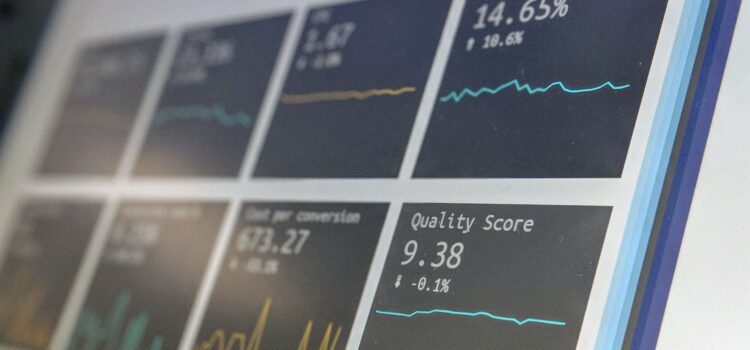
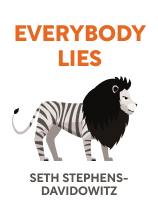
This article is an excerpt from the Shortform book guide to "Everybody Lies" by Seth Stephens-Davidowitz. Shortform has the world's best summaries and analyses of books you should be reading.
Like this article? Sign up for a free trial here .
What are the disadvantages of big data? At what point does data get in the way?
In Everybody Lies, Seth Stephens-Davidowitz warns that good data science isn’t just a matter of amassing a giant data set. When working with data, he says it’s important to keep data’s shortcomings in mind and not lose sight of the bigger picture.
Read more so you can be aware of big data’s disadvantages.
Disadvantage #1: False Correlations
Stephens-Davidowitz says that when a dataset is too detailed, it can lead to predictive errors. The problem, he says, is one of the disadvantages of big data: the curse of dimensionality. It’s a phenomenon whereby the more details a dataset contains, the more likely it is to suggest false positives when you look for predictive correlations.
Stephens-Davidowitz gives the example of flipping coins to try to predict the stock market. Say you flip a coin every day, record whether it was heads or tails, and then record whether the stock market went up or down that day. Stephens-Davidowitz says that if you perform this test using 1,000 coins for two years, it’s likely that by pure chance, at least one coin’s results will appear to correlate with market performance. Obviously this correlation is false. But Stephens-Davidowitz says this problem happens any time you test a lot of variables against a small number of outcomes—such as when trying to predict the stock market or link gene variations to disease likelihood.
(Shortform note: In addition to the risk of drawing conclusions based on random noise as Stephens-Davidowitz describes, the curse of dimensionality can make it hard to draw any meaningful conclusions at all. That happens when you classify data into so many parameters that all data points appear equidistant from each other and there are fewer “clusters” of data to draw your attention—in other words, you can no longer see useful similarities between items.)
Disadvantage #2: Data for Data’s Sake
Stephens-Davidowitz points out that it’s easy to fall in love with data for its own sake. When that happens, we’re likely to lose sight of what the data was supposed to be doing for us in the first place. He gives the example of standardized testing in education, which aims to make teaching and learning measurable by generating data on student outcomes. But in many cases, schools end up focusing on improving their test scores (which are tied to schools’ reputation and funding) by any means necessary—means that include limiting the curriculum in order to focus on test prep and, in extreme cases, cheating on the tests.
Stephens-Davidowitz says that studies suggest the best way to use data to measure teacher quality is to combine test scores with other factors like student evaluations and classroom observation. He says that many fields are finding that this combination of big data and traditional, small-scale information works better than focusing on big data alone.
Big Data vs. Small Data
Similarly, in Small Data, author and branding consultant Martin Lindstrom argues that big data on its own is misleading and that it should be coupled with what he calls “small data”—in-person observation of people’s desires and motivations.
Lindstrom gives the example of LEGO, which tried to address struggling sales by turning to big data research. That research convinced the company that millennials would be easily bored by toy building blocks, so the company simplified its sets in an attempt to offer instant gratification. This approach failed. But when market research interviews with actual children revealed that kids like mastering hobbies, LEGO found more success than ever before by making more complicated sets—an approach that directly contradicted what big data had told them.
Lindstrom’s definition of “small data” is not the only one. Other researchers use the term to describe small-scale measurements of specific attributes—such as wind direction sensors on wind turbines or smart bottle labels that track a medicine’s remaining shelf life. This kind of small data can work on its own (for example, by telling the turbine to adjust its blades to maximize electricity output) or integrate with big data techniques (for example, to track when, where, and why medicines expire on shelves).
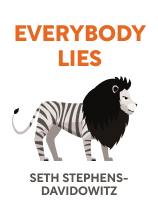
———End of Preview———
Like what you just read? Read the rest of the world's best book summary and analysis of Seth Stephens-Davidowitz's "Everybody Lies" at Shortform .
Here's what you'll find in our full Everybody Lies summary :
- How people confess their darkest secrets to Google search
- How this "big data" can be used in lieu of voluntary surveys
- The unethical uses and limitations of big data