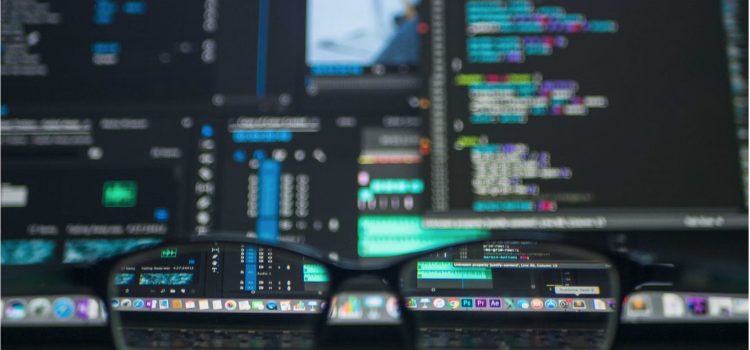
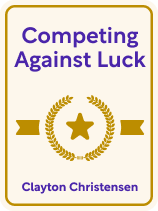
This article is an excerpt from the Shortform book guide to "Competing Against Luck" by Clayton Christensen. Shortform has the world's best summaries and analyses of books you should be reading.
Like this article? Sign up for a free trial here.
What are the disadvantages of data analytics in product development? How can hard data fail to support business innovation?
In his book Competing Against Luck, Clayton Christensen challenges the conventional thinking around business innovation and highlights the disadvantages of relying solely on data analytics to guide decision-making. Christensen argues that this approach often leads to disappointment.
Keep reading to learn the two key disadvantages of data analytics in business, according to Christensen.
Innovation & the Disadvantages of Data Analytics
In his book Competing Against Luck, Clayton Christensen argues that the way most companies think about business innovation is misguided. Christensen explains that this has to do with the disadvantages of data analytics, arguing that many business leaders assume that tracking the right metrics and gathering enough hard data will reveal what profitable products to design or how to make their products better. For instance, a clothing company may track what smartphone apps their customers use to try and learn what their target demographic wants. If the company sees that their customers spend 75% of their time on social media apps, they could interpret this as demand for social engagement and they might respond by adding chatrooms to their shopping app.
These companies spend an exorbitant amount of money on comprehensive data analytics to aid research and development, but often, their innovations fail to make their products much better for the consumer. Their investment is wasted, and any successful innovations they do produce are due to lucky guesses.
(Shortform note: Contrary to Christensen’s claim about the disadvantages of data analytics, some surveys reveal that data analytics may be key to effective product innovation. One survey of 1,500 companies found that 90% of the companies showing improved customer results were rated either “good” or “great” at utilizing data analytics. Additionally, the ubiquitous nature of data analytics may be a testament to its effectiveness. In 2022, companies around the globe spent a total of over $271 billion on data analytics, and this field is only projected to grow. By 2029, companies are projected to spend over $655 billion on data analytics.)
According to Christensen, there are two main disadvantages of data analytics, which means that hard data fail to reliably guide the creation of profitable innovations.
Disadvantage #1: The Illusion of Objectivity
The first disadvantage that Christensen discusses is how data analytics fails to support innovation because it creates the illusion of objectivity. Working with data feels like a hard, objective science, and any ideas for innovation that have numbers to back them up feel much more trustworthy. However, Christensen notes that data aren’t as objective as they seem. The ways that we measure metrics and collect data points often depend on numerous subjective decisions.
For example, imagine that a video streaming service like Hulu is trying to decide whether to pick up a new reality TV show. If they surveyed their existing subscribers to predict how many would watch it, mild differences in this survey process could result in different data sets. If the survey is attached to an enticing video teaser, for instance, it could inflate the numbers—a survey with the teaser may report that 75% of subscribers want to watch the show, while a survey with just a text description may report that only 45% of viewers want to watch it. Even though both surveys would offer seemingly objective numbers, they would both be subjective because they’re the result of arbitrary choices in the data collection process.
Disadvantage #2: The Illusion of Success
Another disadvantage of data analytics is that it creates the illusion of success, argues Christensen. He explains that people tend to see what they want to see when interpreting data. For this reason, teams often interpret inconclusive data as evidence that they’ve successfully created the next big thing, and they consequently invest heavily in a launch doomed to fail.
For example, imagine Baskin-Robbins tries to innovate by offering ice cream wrapped in a burrito. They gather a significant amount of customer feedback that, in their eyes, indicates that this would be a success: The market for casual Tex-Mex food is growing, a huge percentage of their customers are interested in fusion cuisine, and so on. Unfortunately, although these data seem objective and positive, they’re likely masking the failure of a bad idea. These data don’t prove that people want a fusion of Tex-Mex and ice cream, specifically—you’d get the same data even if no one wants to eat a soggy ice cream burrito.
How Superforecasters Overcome the Illusions in Data In Superforecasting, Philip Tetlock and Dan Gardner describe research they conducted on “superforecasters” who consistently made better predictions about the future than most other experts. They identify the specific techniques that allow superforecasters to make their predictions—techniques that arguably allow them to overcome the disadvantages of data analytics and see through the illusions of data that Christensen describes in Competing Against Luck. First, superforecasters overcome the illusion of objectivity by intentionally using rough estimates to make their predictions. By choosing not to rely on precise math to make their predictions, it’s possible that they avoid giving too much weight to data sets potentially compromised by subjective data generation processes. Instead of seeing their predictions as mathematical facts, they more accurately see them as educated guesses. Second, superforecasters are aware of the human tendency to see what you want to see in data—the illusion of success. To compensate for this cognitive bias, superforecasters form groups and practice active open-mindedness, deliberately seeking out counterarguments. If one expert analyzes a data set in a biased way, others can counterbalance them with a more objective view. Tetlock and Gardner find that the best predictions in the world come from teams whose members can comfortably disagree with one another and integrate everyone’s perspectives into logical predictions. |
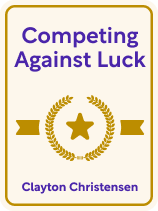
———End of Preview———
Like what you just read? Read the rest of the world's best book summary and analysis of Clayton Christensen's "Competing Against Luck" at Shortform.
Here's what you'll find in our full Competing Against Luck summary:
- How to analyze consumer demand to guide product innovation
- How you can design and sell a product that can't possibly fail
- Tips for increasing your company's chances of success