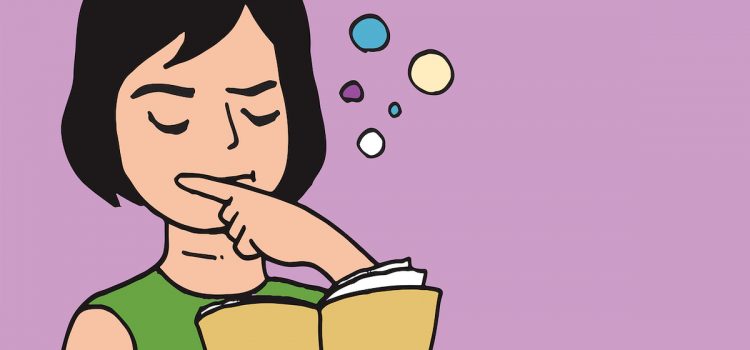
Do you know bullshit when you see it? What do you do about it? Should you call it out?
With the proliferation of misinformation online, in the news, and even in academia, the modern world is replete with nonsense and flat-out lies. In their book Calling Bullshit, Carl T. Bergstrom and Jevin D. West contend that anyone can learn how to detect and refute bullshit in its many forms.
Continue reading for an overview of this book that’s more important than ever.
Overview of Calling Bullshit
Bergstrom and West define bullshit as the use of misleading evidence to persuade an audience. But, they argue in their 2020 book Calling Bullshit that we aren’t defenseless against this tactic.
Bergstrom and West’s complementary backgrounds are evident throughout Calling Bullshit. Bergstrom’s expertise in evolutionary biology lends him insight into the ways that bullshit can sneak into formal scientific models. In a similar vein, West’s experience as the co-founder of the University of Washington’s DataLab—a hub for data science and analytics research—informs his discussion of how data can be misappropriated to create bullshit. Bergstrom and West have long co-taught a class at the University of Washington called “Calling Bullshit,” and this book codifies the lessons from their class.
We’ll begin with an introduction to bullshit, examining Bergstrom and West’s definition of bullshit and explaining why bullshit pervades the news and the internet. Next, we’ll discuss the varieties of bullshit most prevalent in contemporary society—namely, bullshit based on data. We’ll analyze how data can be miscollected and misinterpreted, creating bullshit that infects science and big data. Finally, we’ll examine Bergstrom and West’s concrete strategies for identifying and calling bullshit.
An Introduction to Bullshit
Bergstrom and West note that to recognize specific forms of bullshit, you have to understand what bullshit is more generally. To that end, we’ll begin by discussing their general thesis about the nature of bullshit and outlining why bullshit is so pervasive online and in the news.
What Is Bullshit?
Bergstrom and West define bullshit as the use of misleading evidence, without regard for the truth, to sway an audience by confusing or overwhelming them.
This definition, they point out, has two key aspects. First, the bullshitter appeals to evidence that obscures the truth, rather than illuminating it. For example, this might involve using bombastic language that makes it difficult for an audience to follow the argument. Alternatively, this could involve using statistics or data taken out of context.
Second, Bergstrom and West say, the bullshitter bears no commitment to the truth. This lack of commitment manifests in two ways. Most obviously, it can manifest when someone actively seeks to undermine the truth, such as by intentionally making false claims to advance a political agenda. The lack of commitment can also manifest when someone is simply indifferent to the truth, such as a comedian who embellishes her stories for the sake of entertaining a crowd. For such entertainers, the truth is simply irrelevant.
Crucially, Bergstrom and West add that black boxes can immunize bullshit from criticism. Black boxes, they explain, are highly complex sources of evidence that laypeople are not equipped to evaluate. For instance, a climate change activist might appeal to projections from the Dynamic Integrated Climate Change Model to argue that the threat of climate change is more serious than most assume. Because this multivariable climate model lies beyond the expertise of most laypeople, it constitutes a black box that is effectively beyond reproach.
Bullshit Online and in the News
Although bullshit can exist in any era, Bergstrom and West contend that two components of contemporary society—the internet and the mainstream media—are particularly potent vehicles for spreading bullshit. They argue that because the internet and the media are incentivized to earn revenue through clicks and views, they often propagate bullshit to meet this end since bullshit often sounds far more interesting than the unalloyed truth.
First, Bergstrom and West point out that the internet created an entirely new marketplace of ideas that inundated us with information. But, unlike previous information sources that earned money via subscriptions—such as paper magazines and newspapers—website owners mostly earn money via advertising revenue. Consequently, online stories seek to generate clicks to earn this revenue.
Further, the authors note that according to one 2017 study, the internet headlines that generate the most clicks are emotive, rather than factual. In practice, this means that facts are not enough to compete in the online marketplace because online authors care more about clicks (and therefore money) than truth. For this reason, the internet is a fertile ground for bullshit since bullshit allows you to write emotionally charged headlines with no regard for the truth.
Additionally, Bergstrom and West contend that similar incentives have influenced the media since the 1987 repeal of the Fairness Doctrine. The Fairness Doctrine, they explain, required news outlets to include competing viewpoints when discussing contentious ideas. Bergstrom and West write that, since its repeal, mainstream media sources have become increasingly partisan, promoting bullshit to more easily push a partisan agenda. Moreover, the authors observe that social media algorithms have amplified this partisanship, as they only expose users to sources that reinforce their existing views. Bergstrom and West contend that because these algorithms aim to generate views, they likewise promote bullshit at the expense of truth.
Brandolini’s Principle
The perverse incentives that infect the internet and the media aren’t the only reason bullshit is so widespread. Bergstrom and West maintain that another reason is codified in Brandolini’s principle: It takes exponentially more energy to refute bullshit than to produce it. Thus, bullshit online and in the news accumulates faster than we can eradicate it.
In addition to its intuitive plausibility, Bergstrom and West note that Brandolini’s principle is supported by several studies examining the spread of rumors on social media platforms. For example, one study found that even after being “fact-checked” by agencies like Snopes, false rumors continued to spread more rapidly on Facebook than true rumors.
Varieties of Contemporary Bullshit
Historically, bullshit was often transmitted via rhetorical devices like hyperbole, ad hominem attacks, and false analogies. However, Bergstrom and West point out that the proliferation of data-based arguments in contemporary society has given rise to new variants of bullshit that rely heavily on data analytics. We’ll outline three such types of bullshit—bullshit arising from improper data collection, bullshit arising from improper data interpretation, and bullshit arising in science and big data in particular.
How Improper Data Collection Creates Bullshit
According to Bergstrom and West, bullshit often arises when data-based arguments rely on data that was itself flawed. Specifically, they argue that selection bias can lead to bullshit because it justifies faulty conclusions based on unrepresentative samples.
Selection bias, Bergstrom and West explain, occurs whenever the population sampled for a research study doesn’t represent the broader population that you’re interested in. For example, if you wanted to know how the US population would vote in the 2024 election, you could commit selection bias by only polling (say) senior citizens. And although selection bias takes many forms, Bergstrom and West focus on two in particular: the observation selection effect and data censoring.
Selection Bias #1: The Observation Selection Effect
Bergstrom and West maintain that selection bias can creep into data via the observation selection effect, which occurs when our data collection process is correlated with the variable that we’re collecting data on. For example, if we could only conduct presidential polls via smartphones, the correlation between smartphone ownership and presidential preference would undermine the integrity of these polls—smartphone owners may be wealthier, on average, and therefore more likely to vote for a candidate who cuts taxes for the rich.
The observation selection effect, Bergstrom and West argue, can yield misleading conclusions that bullshitters can prop up. Returning to the previous example, bullshitters could use poll data conducted exclusively by smartphone to spread a false narrative that one candidate was guaranteed to win, when in fact their chances might be much lower than the poll suggests.
Selection Bias #2: Data Censoring
Bergstrom and West explain that in addition to the observation selection effect, data censoring can be another source of selection bias. They relate that data censoring occurs whenever an initially random sample becomes non-random at the completion of a study because a non-random subset of that initial sample was ineligible for inclusion in the study’s results.
For instance, imagine that we conducted a study in 2023 assessing the life expectancy of individuals born in the 1900s versus those born in the 2000s. Because individuals who are still alive can’t figure into our results, the 2000s sample would appear to have a drastically lower life expectancy because it could only include those who had died by 2023 (and thus were at most 23 years old). Although data censoring is rare, Bergstrom and West caution that its misleading results can be propagated by bullshitters.
How Misinterpreting Data Creates Bullshit
Even when safe from selection bias, data can nonetheless be manipulated to promote bullshit. According to Bergstrom and West, bullshitters often misinterpret data to draw unjustified conclusions, either by making invalid inferences from valid data or by visually misrepresenting valid data in graphic form.
Shoddy Inferences From Sound Data
Bergstrom and West write that bullshitters employ various strategies to infer unsound conclusions from sound data. We’ll examine three such strategies: inferring causation from correlation, discussing numbers out of context, and presenting misleading percentages.
Strategy #1: Causation From Correlation
Humans naturally seek out causal narratives, write Bergstrom and West. For this reason, we often judge that one phenomenon causes another, even when our evidence only suggests the two phenomena are correlated. For example, it’s well known that increasing ice cream sales are positively correlated with increasing drowning rates. However, it’d be foolish to conclude that ice cream is causing the uptick in drownings—according to a more likely explanation, ice cream sales increase in the summer, and drownings also increase in the summer because more people swim when the weather is warmer.
Consequently, bullshit often arises when people make causal conclusions based on mere correlation. This bullshit is especially pervasive in mainstream news outlets, with one analysis finding that almost half of all news articles that report on research studies identifying correlations between two variables framed these studies as having established a causal link.
Strategy #2: Numbers Without Context
In a similar vein, Bergstrom and West contend that taking numbers out of context can lead to bullshit. For example, if a politician proudly announces that his economic plan has increased the average citizen’s income by $10,000, the economic plan could seem beneficial for the average citizen. However, if this plan only increased the income of the wealthiest 1% of citizens, thus inflating the average, then it may be less universally beneficial than it appears. Thus, by presenting the average income increase without the context of how this increase was distributed across the population, the politician can promote a false view of his economic plan.
Strategy #3: Misleading Percentages
Further, Bergstrom and West explain that using percentages can obscure true values and promote bullshit. For instance, a tobacco company might point out that in 2022, of 330 million Americans, only 0.2% died of cancer. While technically correct, this percentage obscures the fact that cancer accounted for almost 20% of deaths among Americans in that same year, as it caused 600,000 deaths out of 3.2 million. Percentages can therefore be a powerful vehicle for disguising bullshit.
Misleading Data Visualizations
According to Bergstrom and West, bullshitters can further manipulate valid data by using strategies to create misleading representations. Although Bergstrom and West list an array of strategies for misleading viewers, we’ll focus on three particularly illuminating ones: prioritizing form over function, shoehorning data in suboptimal visualizations, and using disproportionate visualizations.
Misleading Strategy #1: Prioritizing Form Over Function
Bergstrom and West argue that prioritizing form over function can create bullshit that obscures the data that a visualization attempts to convey. For instance, imagine that you wanted to represent the proportion of Americans associated with a specific religious affiliation in graphical form. If, instead of representing this information via a simple bar chart, you chose to represent it via a shaded map of the United States, that shaded map would be neglecting function for the sake of form. After all, although the shaded map of the United States might look cooler, it would also be more difficult to interpret.
Misleading Strategy #2: Shoehorning Data Into Inappropriate Visualizations
While an excessive focus on form can be innocuous, Bergstrom and West contend that shoehorning data into inappropriate forms is an unequivocal attempt to create bullshit. The clearest example of this is the proliferation of visual representations that parallel the periodic table; for instance, there are periodic tables of marketing techniques, cryptocurrencies, and typefaces. But, while the original periodic table was carefully designed to convey the chemical groupings of different elements, these mock periodic tables are not. Rather, they attempt to convey an appearance of rigor which their underlying data lack.
Misleading Strategy #3: Using Disproportionate Visualizations
Finally, the authors argue that certain data visualizations spread bullshit by violating statistician Edward Tufte’s requirement of proportionate visualizations. In his 1983 book, The Visual Display of Quantitative Information, Tufte argues that the size of regions that represent data should be proportionate to the values they represent. For instance, if you’re creating a bar chart that illustrates the number of Nobel Prize winners by country, then the bar representing Germany’s 111 Nobel laureates should be just over five times as large as the bar representing Austria’s 22 Nobel laureates.
Bergstrom and West point out that, although the principle of proportionate visualization seems commonsensical, violations of it are common—any time a bar chart’s vertical axis doesn’t start at zero, it will violate this principle. Such charts can convey bullshit because they misrepresent the scale of the difference between two values, providing a ripe ground for false inferences. For example, imagine we created a bar chart measuring Nobel laureates by country that was evenly spaced but started at 20 rather than zero. Then, Germany’s bar would be 91 units tall (111 minus 20) while Austria’s would be 2 units tall (22 minus 2), creating a misleading picture of the difference between the two countries.
How Experts Give Rise to Bullshit
While there are various ways to mistakenly collect and interpret data, it’s tempting to think that official forms of data analysis are immune from bullshit. But, according to Bergstrom and West, this couldn’t be further from the truth. They argue that bullshit is widespread both in science and in so-called “big data.”
Scientific Bullshit
According to Bergstrom and West, even the institution of science isn’t immune from bullshit. On the contrary, they argue that science’s focus on statistical significance gives rise to bullshit for two reasons: We can easily misinterpret what statistical significance means, and we’re only exposed to statistically significant findings because of publication bias.
For context, they explain that a statistically significant finding is one with a certain p-value—a statistical measure of how likely it is that a study’s result happened by pure chance.
For example, imagine you wanted to see if there was a relationship between smoking cigarettes daily and getting lung cancer. You could perform a statistical analysis comparing the rates of people with lung cancer who did, and did not, smoke cigarettes daily. If you found a positive correlation between smoking and cancer and the resulting p-value of that correlation was less than 0.05, scientists would normally consider that a statistically significant result—one that is unlikely to occur from chance alone. If your analysis yielded a p-value of 0.01, that would mean there would be a 1% chance of it occurring if there weren’t a correlation between smoking and cancer.
However, Bergstrom and West point out that many people misinterpret the p-value, taking it to be the likelihood that there’s no correlation between the variables tested, which leads to bullshit when they overstate the results of statistical analyses. For example, just by sheer chance, it’s possible that if we flipped two coins 100 times, they would land on the same side 60 times, yielding a p-value of around 0.03 (in other words, there’s about a 3% chance of getting this result by pure luck). But we would be mistaken to conclude that the likelihood the two coins are connected is 0.97 because we know that barring any funny business, two simultaneous coin flips are independent events. So, we would instead be justified in concluding that the low p-value was a statistical anomaly.
Further, Bergstrom and West contend that publication bias can promote bullshit by creating a distorted view of scientific studies. Publication bias refers to scientific journals’s tendency to only publish statistically significant results since such results are considered more interesting than non-significant results. In practice, this means published scientific studies often report statistically significant results even when these results don’t necessarily indicate a meaningful connection.
For example, even though there isn’t a connection between astrological signs and political views, if 100 studies attempted to test this relationship, we should expect about five to have a p-value below 0.05. Because these five studies would likely get published while the other 95 wouldn’t, scientific journals would inadvertently promote the bullshit view that there’s a connection between astrology and politics because of publication bias.
Big Data Bullshit
In a similar vein, Bergstrom and West argue that big data—a technological discipline that deals with exceptionally large and complex data sets using advanced analytics—can foster bullshit because it can incorporate poor training data and find illusory connections by chance.
For context, Bergstrom and West explain how big data generates computer programs. They relate that researchers input an enormous amount of labeled training data into an initial learning algorithm. For instance, if they were using big data to create a program that could accurately guess people’s ages from pictures, they would feed the learning algorithm pictures of people that included their age. Then, by establishing connections between these training data, the learning algorithm generates a new program for predicting people’s ages. If all goes well, this program will be able to correctly assess new test data—in this case, unfamiliar pictures of people whose ages it attempts to predict.
However, Bergstrom and West argue that flawed training data can lead to bullshit programs. For example, imagine that we used big data to develop a program that allegedly can predict someone’s socioeconomic status based on their facial structures, using profile pictures from Facebook as our training data. One reason why this training data could be flawed is that people from higher socioeconomic backgrounds typically own better cameras, and thus have higher-resolution profile pictures. Thus, our program might not be directly identifying socioeconomic status, but rather camera resolution. In turn, when exposed to training data not sourced from Facebook, the big data program would likely fail to distinguish between socioeconomic status.
In addition, Bergstrom and West point out that, when given enough training data, these big data programs will often find chance connections that don’t apply to test data. For instance, imagine that we created a big data program that aimed to predict the presidential election based on the frequency of certain keywords in Facebook posts. Given enough Facebook posts, chance connections between certain terms may appear to predict election outcomes. For example, it’s possible that posts including “Tom Brady” have historically predicted Republican victories, just because the Patriots have happened to win on the verge of Republican presidential elections.
How to Deal With Bullshit
Although the modern world is rife with bullshit, we aren’t defenseless against it. On the contrary, Bergstrom and West argue that several strategies can help us recognize and refute bullshit. We’ll examine these strategies in depth, first focusing on ways to identify bullshit before concluding with ways to call bullshit.
How to Identify Bullshit
We’ll focus on three of Bergstrom and West’s key strategies for identifying bullshit: Evaluate information sources, scrutinize claims that are “too good to be true,” and be wary of confirmation bias.
Strategy #1: Evaluate Information Sources
Bergstrom and West explain that we should assess information sources by asking who the information is coming from and what their possible motivations are. After all, many information sources have ulterior motives, meaning they’re more likely to use bullshit to support their aims. For example, in light of conclusive evidence linking smoking to lung cancer in the 1950s, the tobacco industry conducted a marketing campaign that sought to undermine this scientific consensus (and thus retain their massive profits). This campaign was rife with bullshit, but by asking what the tobacco industry’s underlying motivations were, you could have easily detected this bullshit.
Strategy #2: Scrutinize Implausible Claims
Next, Bergstrom and West recommend being careful when you encounter claims that seem too good to be true. In other words, if a claim seems absolutely implausible, there is a good chance that it’s bullshit. For instance, in so-called “Nigerian prince scams,” scammers email potential targets claiming to be international royalty in need of financial assistance, promising to quickly return your loans with sizable interest. These scams are too good to be true—Nigerian royalty certainly wouldn’t solicit loans via anonymous emails. Thus, by treating such claims with a healthy amount of skepticism, you’ll often be able to detect bullshit.
Strategy #3: Remain Cognizant of Confirmation Bias
Finally, Bergstrom and West caution us that confirmation bias—the tendency to hold claims that conform to our preexisting views to lower evidentiary standards—can blind us to bullshit. For example, imagine that allegations broke that a politician you disliked had committed fraud or had an affair. If you already distrusted that politician, you might be more likely to accept these allegations at face value—even if they ultimately proved to be bullshit.
How to Call Bullshit
Finally, Bergstrom and West acknowledge that identifying bullshit alone isn’t enough to mitigate its spread. To that end, we’ll discuss three of their techniques for calling bullshit so that others don’t fall for it: Construct a reductio ad absurdum, provide counterexamples, and use clarifying analogies.
Technique #1: Construct a Reductio ad Absurdum
Bergstrom and West explain that a reductio ad absurdum (“reduction to absurdity”) can be a powerful tool for exposing bullshit. Constructing a reductio ad absurdum involves showing that a claim has an obviously false consequence, and thus logically cannot be true. For example, imagine that you read an op-ed arguing that parents should have absolutely no restrictions on how they choose to raise their children. In this case, you could construct a reductio ad absurdum pointing out that this op-ed’s view implies that parents should have the right to abuse or neglect their children. Because this implication is ridiculous, the original view must be false.
Technique #2: Provide Counterexamples
A similar technique for calling bullshit involves providing counterexamples to bullshit claims. To provide a counterexample, point out a situation in which a bullshit theory or claim makes a false prediction. For instance, if someone made the sweeping claim that you must get a college education to make a good living, you could provide a counterexample by pointing to the many millionaire entrepreneurs who don’t have degrees. Counterexamples thus provide a simple way to refute bullshit generalizations.
Technique #3: Use Clarifying Analogies
Finally, Bergstrom and West argue that analogies can be a useful tool for shedding light on bullshit that hides in seemingly plausible claims. To provide an analogy that exposes bullshit in an argument, create an argument that parallels the bullshit argument but is clearly invalid. For example, imagine that several parents defended the decision to spank children on the grounds that they had spanked their children without any adverse effects. To underscore why this argument is bullshit, you could offer an analogous defense of drunk driving that notes that many people who drive drunk don’t get into car crashes. This analogy makes it clear that just because a practice sometimes has no adverse effects, that doesn’t mean it’s a good practice.
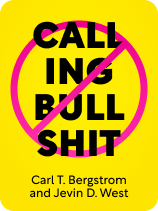
———End of Preview———
Like what you just read? Read the rest of the world's best book summary and analysis of Carl T. Bergstrom and Jevin D. West's "Calling Bullshit" at Shortform.
Here's what you'll find in our full Calling Bullshit summary:
- That misinformation online, in news, and in academia is spreading
- How to detect and refute bullshit in its many forms
- How data can be miscollected and misinterpreted in science