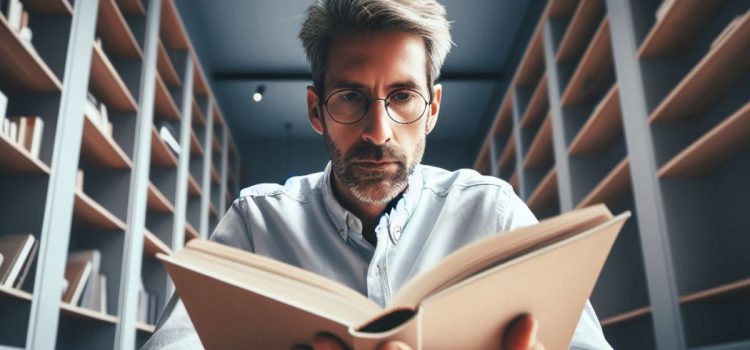
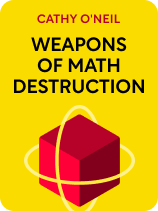
This article is an excerpt from the Shortform book guide to "Weapons of Math Destruction" by Cathy O'Neil. Shortform has the world's best summaries and analyses of books you should be reading.
Like this article? Sign up for a free trial here.
What’s Weapons of Math Destruction about? How is math used to discriminate against people in poverty?
In Weapons of Math Destruction, Cathy O’Neil explores the difference between benign mathematical models and dangerous ones. She also describes the negative effects that dangerous mathematical models produce.
Read below for a brief Weapons of Math Destruction book overview.
Weapons of Math Destruction by Cathy O’Neil
In her Weapons of Math Destruction book, Cathy O’Neil details the insidious ways organizations use mathematical models to determine everything from interest rates on mortgages to prison sentences. A mathematical model is a simulation of a real-world event or situation, which is used to analyze and predict outcomes—companies and organizations use these models to set prices, target audiences, and make an increasingly large number of decisions.
According to O’Neil, when people use these mathematical models without disclosing their methods or incorporating feedback, the models can produce dangerous results. Specifically, O’Neil argues that dangerous mathematical models treat poor people worse than rich people, reinforce bias by lumping people into groups, and produce judgments that become self-fulfilling prophecies. Weapons of Math Destruction is O’Neil’s attempt to bring these issues to light.
O’Neil is a data scientist, professor, and journalist who has written extensively on issues in data science. Weapons of Math Destruction, published in 2016, is a New York Times best seller and winner of the 2019 Euler Book Prize.
Benign Models and Dangerous Models
O’Neil notes that not all mathematical models are dangerous. When implemented responsibly, mathematical models can be powerful tools that enable organizations to make effective decisions that wouldn’t otherwise be possible. Below we’ll define mathematical models and briefly discuss their limitations before moving on to describe the traits of dangerous mathematical models.
What Are Mathematical Models?
According to O’Neil, a mathematical model is a mathematical simulation of a real-world event. Organizations use mathematical models to make predictions and decisions more efficiently than they would otherwise be able to when handling large amounts of data.
For example, suppose you recently adopted 100 cats, which you allow to freely roam your 100-acre property. To figure out where and when to leave food for the cats, you equip 20 of them with GPS collars to track their movements throughout the day. Based on the data from the collars, you estimate where the best places to leave food are—you’ve used data to create a simple mathematical model that simulates the movement of your cats without having to track all 100 cats.
O’Neil argues that mathematical models are inherently reductive. Real-world events and people are immensely complex, and it’s impossible to input every factor that influences them into an equation. Lacking all the information necessary to simulate an event flawlessly, mathematical models inevitably produce a few mistakes. Organizations that use mathematical models are aware of these limitations, but they nonetheless use mathematical models because they’re willing to accept the trade-off between accuracy and efficiency.
To return to our example, your mathematical model of cat movements will occasionally return inaccurate results. As any cat owner can tell you, cats do what they want, when they want, coming and going in ways that aren’t entirely predictable. However, you decide that it’s still worth it to feed the cats based on the information your model spits out, as it’s much more efficient than tracking down and feeding each cat each day—and as long as all the cats are getting enough food, there’s no harm done.
O’Neil cautions that in addition to their limited accuracy, mathematical models also have ethical limitations. Mathematical models reflect the biases and incorrect assumptions of the people who make them. Additionally, unlike humans, mathematical models are unable to factor in moral and ethical concepts like equality.
For example, a cat feeding model designed by someone who incorrectly assumed that cats spend most of their lives in trees would probably place food sources up high. Based on this model, you’d end up distributing food unfairly, placing it where only the most nimble and adventurous cats would have access to it, while their terrestrial siblings would be left to fend for themselves.
The Characteristics of Dangerous Mathematical Models
Now that we understand what mathematical models are, let’s consider the three characteristics that make them dangerous: They’re opaque, they don’t incorporate feedback, and they operate on a large scale.
Dangerous Models Are Opaque
According to O’Neil, dangerous mathematical models don’t make their methods known to the public. Often, the inner workings of these models are considered valuable proprietary information—and the companies that own these models closely guard this information. O’Neil argues that this lack of transparency makes it impossible for outsiders to critique a particular model as outsiders aren’t permitted to know how the model works.
Lack of transparency also makes it impossible for individuals to protest the way models judge them. For example, if a bank uses a mathematical model to decide to deny you a loan and refuses to explain how the model reached that decision, you’re left with no recourse except to look elsewhere.
By contrast, good mathematical models make their methods transparent, says O’Neil. By publicizing the data and methods they use to make their judgments, they open themselves to critique—if a method is obviously flawed or unfair, transparency allows outsiders to point out the flaw so it can be corrected.
Transparency is especially important in models that judge individuals, such as credit scores. Transparency allows consumers to understand the criteria their score is based on and the steps they can take to improve their score.
Dangerous Models Don’t Incorporate Feedback
The next defining characteristic of dangerous mathematical models is a failure to incorporate feedback. According to O’Neil, good mathematical models are constantly incorporating feedback based on the accuracy of their predictions. If their predictions are accurate, the model remains unchanged, but when errors are detected, the model is adjusted. By incorporating feedback, good models slowly become more accurate over time, as their equations and algorithms are tweaked to avoid replicating old errors.
By contrast, dangerous models don’t collect or incorporate feedback. Models that don’t collect feedback remain oblivious to their own errors. If the original model includes bias, such models will continue to replicate that bias, without their designers ever realizing what’s happening.
For example, suppose a news channel in Stockholm debuts a new weather forecasting model that boldly predicts 90-degree highs in the middle of winter. If it’s a good model, it’ll be tweaked over the next week as each of its predictions misses the mark. However, a dangerous model would simply be allowed to continue incorrectly predicting unseasonable highs and lows, without incorporating the results of those predictions into its algorithms.
Dangerous Models Operate on a Large Scale
The final characteristic of dangerous mathematical models is excessive scale. Good mathematical models are used at a reasonable scale, only in the contexts they were designed to simulate. When used within their designated contexts, good mathematical models often make accurate predictions, and when they fail, the harm they do is limited.
For example, imagine a mathematical model designed to predict your favorite color based on your shopping habits. Used within a reasonable context, this model might be used to present you with ads for clothing in your favorite color—such a model would be helpful to both you and the advertiser. And, if the model got your favorite color wrong, the only consequence would be presenting you with a blue dress instead of a red one.
By contrast, dangerous mathematical models are deployed at massive scale, often far beyond the contexts they were originally designed for. O’Neil says that when used in such broad contexts, even low rates of inaccuracy cause harm to many people, as even small fractions of massive numbers often represent sizable groups of people.
For example, imagine that researchers discover a slight correlation between favorite color and credit—people who prefer blue are found to be slightly more likely to pay their bills on time than those who prefer red. In response, banks start using the favorite color model to set interest rates for mortgages. Nationwide, people start paying different amounts for the same services, all because their favorite color supposedly corresponds to their reliability. As a result of being deployed at scale, far beyond its intended context, the favorite color model becomes dangerous.
Dangerous Models Produce Dangerous Effects
Now that you understand the differences between good mathematical models and dangerous ones, let’s consider the impact dangerous models have on society. According to O’Neil, dangerous models disproportionately harm poor people, reproduce social bias, and make harmful self-fulfilling prophecies.
Dangerous Models Disproportionately Inflict Harm on the Poor
O’Neil argues that dangerous mathematical models tend to harm poor people while offering preferential treatment to rich people. It’s more efficient and cost-effective for institutions to automate the bulk of their interactions, so they use mathematical models to determine college admissions, sort through job applications, evaluate job performance, and calculate credit scores—all processes that favor wealthy people’s backgrounds and circumstances.
The result is that poor people often get poorer from their interactions with dangerous mathematical models, while rich people largely benefit from their interactions with the same systems. Over time, this leads to increased income disparity and social inequality.
For example, algorithms that calculate credit scores are designed to predict a borrower’s ability to repay loans based on factors like loan repayment history, current debt levels, and income. Poor people, often with low scores due to unstable incomes, little credit history, or small financial missteps (such as late bill payments, which are common among those struggling financially), face higher loan interest rates or denial of credit, amplifying their financial struggles. Conversely, wealthier individuals often have long credit histories and enough resources for on-time payments, leading to high credit scores.
Dangerous Models Reproduce Social Bias by Lumping People Into Groups
According to O’Neil, dangerous mathematical models tend to mete out identical judgments to large groups of individuals who share characteristics such as location, income level, race, and gender. This often results in the replication of human biases, as harsh judgments are meted out to people from a certain background or geographical area.
Dangerous Models Make Self-Fulfilling Prophecies
O’Neil asserts that the negative judgments made by dangerous mathematical models often turn into self-fulfilling prophecies. This is especially true of mathematical models that are used to predict your financial behavior. If mathematical models deem you to be financially unreliable, you may be unable to get a mortgage, a credit card, a car, or an apartment. These financial consequences make it difficult for you to improve your financial situation, leading to a vicious cycle, in which it’s impossible to overcome the negative judgment of a dangerous model.
How to Defuse Dangerous Models
In response to these negative effects, O’Neil proposes strategies industries and governments can take to limit the harm caused by dangerous mathematical models. O’Neil recommends monitoring and measuring the impact of mathematical models, regulating industry use of mathematical models, and setting more positive goals for mathematical models as opposed to targeting profitability above all else.
Measure Impact
O’Neil argues that you need to measure the effects of dangerous mathematical models before you can mitigate them. As we’ve learned, good mathematical models incorporate feedback, sharpening their algorithms based on previous results. Measuring the impact of dangerous models creates feedback that can be used to improve those models.
When measuring a mathematical model’s impact, consider whether the model’s judgments are fair. Ask whether the model treats all individuals equitably or whether it produces judgments that either favor or punish some groups or individuals unfairly.
For example, if your company uses a mathematical model to sort through job applications, evaluate whether it’s favoring men or those with typically white names. Then consider refining the model so that it ignores names altogether and instead zeroes in on qualifications.
Regulate Industry
O’Neil’s second recommendation for limiting the damage done by dangerous models is to impose regulations on industry use of mathematical models. Since data science is a relatively new field, there are few government strictures on how companies are allowed to use customer data. O’Neil argues that increased regulatory oversight will help prevent companies from misusing mathematical models.
Specifically, O’Neil supports mandatory transparency for companies that use algorithms. Transparency allows consumers to understand the criteria that they’ll be judged on. This increases fairness, as those customers will better understand what measures they can take to improve their standing.
Transparency also helps bring to light patently unfair processes, such as models that discriminate based on race or gender.
O’Neil also proposes increased regulation around which data organizations are allowed to collect and sell. She notes that in Europe, many countries have adopted an opt-in model regarding data collection. Under such a model, none of your personal data can be collected and sold unless you opt-in to a given program.
Set Different Goals
O’Neil writes that in addition to measuring impact and increasing regulation, organizations should optimize their models for fairness and social good and incorporate feedback to increase fairness over time. She says companies should build mathematical models with values and ethical standards included in their decision-making processes. Many of the problems produced by dangerous models occur because companies optimize models for profit alone.
For example, people who are strapped for cash and search online about food stamps might be served ads for high-interest payday loans. While these ads generate profits for the advertiser and the search engine, they do this at the expense of vulnerable consumers. In this case, regulation should look beyond the bottom line and factor in the ethics of such predatory practices.
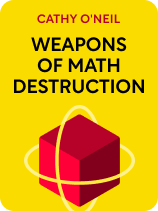
———End of Preview———
Like what you just read? Read the rest of the world's best book summary and analysis of Cathy O'Neil's "Weapons of Math Destruction" at Shortform.
Here's what you'll find in our full Weapons of Math Destruction summary:
- The insidious ways mathematical models are being used in society
- How mathematical models are being used to reinforce bias and harm the poor
- Recommendations for how to rein in dangerous mathematical models