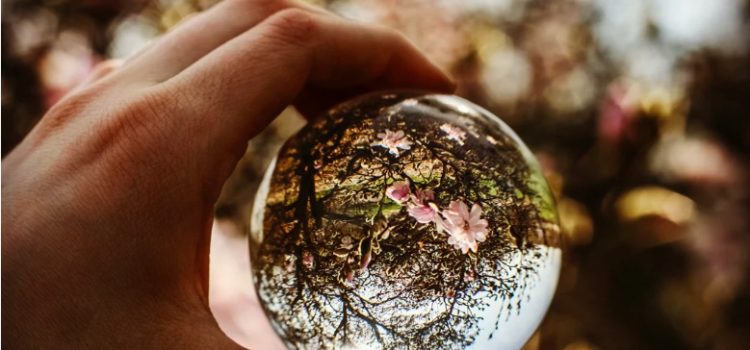
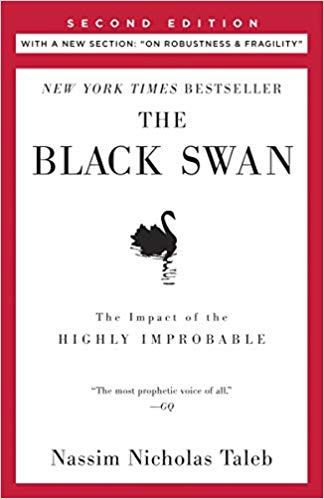
This article is an excerpt from the Shortform summary of "The Black Swan" by Nassim Taleb. Shortform has the world's best summaries of books you should be reading.
Like this article? Sign up for a free trial here .
Why do you make bad predictions? Is there any way to make better predictions?
According to Nassim Nicholas Taleb in The Black Swan, some of the most world-altering events are unpredictable. Although many day-to-day events can be predicted, humans are bad at making accurate predictions about many major events. We’ll look at 12 fallacies and cognitive biases that encourage your bad predictions.
Are Bad Predictions Inevitable?
The Black Swan is named after a classic error of induction wherein an observer assumes that because all the swans he’s seen are white, all swans must be white. Black Swans have three salient features:
- They are rare (statistical outliers);
- They are disproportionately impactful; and, because of that outsize impact,
- They compel human beings to explain why they happened—to show, after the fact, that they were indeed predictable.
Taleb’s thesis, however, is that Black Swans, by their very nature, are always unpredictable—they are the “unknown unknowns” for which even our most comprehensive models can’t account. The fall of the Berlin Wall, the 1987 stock market crash, the creation of the Internet, 9/11, the 2008 financial crisis—all are Black Swans.
Bad Prediction Reason #1: The Illusion of Understanding
We all tend to think we have a grasp of what’s going on in the world when, in fact, the world is far more complex than we know.
For example, all the adults around Taleb predicted the civil crisis would last a matter of days (it ended up lasting around 17 years). Despite the fact that events kept contradicting people’s forecasts, people acted each day as though nothing exceptional had occurred.
Bad Prediction Reason #2: The Retrospective Distortion
History always appears predictable (or, at least, explainable) in our retrospective accounts of events.
In the case of the Lebanese Civil War, the adults whose forecasts were continually proved incorrect were always able to explain the surprising events after the fact. In other words, the events always were indeed predictable, but one could only predict them after they’d already happened.
Bad Prediction Reason #3: The Overvaluation of Facts and the Flaw of Expertise
We accumulate information and listen to expert analysis of that information, but these elements never measure up to real events.
Taleb cites the example of his grandfather, who eventually rose to deputy prime minister of Lebanon. Although his grandfather was an educated man with years of experience in politics, his forecasts were proven wrong as routinely as those of his uneducated driver. Neither knew more than the other about the twists and turns of the war.
Newspapers, too, did nothing to help the Lebanese understand the war. They communicated information, but they didn’t make anyone’s predictions any more accurate. The reporters of the war also tended to “cluster”—emphasizing the same details and using the same categories as each other. Clustering reduces the complexity of the world—and leaves us vulnerable to Black Swans.
Bad Prediction Reason #4: The Error of Confirmation
All too often we draw universal conclusions from a particular set of facts. For example, if we were presented with evidence that showed a turkey had been fed and housed for 1,000 straight days, we would likely predict the same for day 1,001 and for day 1,100.
Taleb calls this prediction the “round-trip fallacy.” When we commit the round-trip fallacy, we assume that “no evidence of x”—where x is any event or phenomenon—is the same as “evidence of no x.”
For example, in the turkey illustration, we might assume that “no evidence of the possibility of slaughter” equals “evidence of the impossibility of slaughter.” To take a medical example, if a cancer screening comes back negative, there is “no evidence of cancer,” not “evidence of no cancer” (because the scan isn’t perfect and could have missed something).
In addition to drawing broad conclusions from narrow observations, we also have a tendency to select evidence on the basis of preconceived frameworks, biases, or hypotheses. For example, a scientist conducting an experiment may, often unconsciously, discount evidence that disconfirms her hypothesis in favor of the evidence that confirms it. Taleb calls this habit “naive empiricism,” but it’s more commonly known as “confirmation bias.”
Taleb’s solution to naive empiricism/confirmation bias is negative empiricism—the rigorous search for disconfirming, rather than corroborating, evidence. This technique was pioneered by a philosopher of science named Karl Popper, who called it “falsification.” The reason negative empiricism/falsification is so effective is that we can be far more sure of wrong answers than right ones.
Bad Prediction Reason #5: The Narrative Fallacy
Because humans are naturally inclined to stories, with distinct causes and effects, we are perennially in danger of committing the “narrative fallacy”—the ascription of meaning or cause to random events.
Our tendency to narrativize is part and parcel of our compulsion to interpret. Humans are evolutionarily conditioned—by the development of the left hemisphere of our brains—to reduce the complexity of the world’s information (we’ll discuss why in a moment); and the most efficient way of simplifying that complexity is through interpretation.
Neurotransmitters in the brain, too, encourage interpretation. When patients are administered dopamine supplements, they become more likely to see patterns where there are none.
Why are humans predisposed to interpretation? For a very practical reason: It makes information easier for our brains to store. Whereas retaining 100 randomly ordered numbers would be near impossible, retaining 100 numbers that were ordered according to a specific rule would be much easier. When we interpret—or narrativize—we’re attempting to impose our own organizing rule on the random facts of the world.
Bad Prediction Reason #6: The Distortion of Silent Evidence
History—which Taleb defines as “any succession of events seen with the effect of posterity”—is inevitably, necessarily distorted. That is, no matter how “factual” or “objective,” the historical record is always a product of our tendency to narrate and thus always biased.
What the narratives are biased against is randomness—the inexplicability and importance of Black Swans.
Take most CEOs’ and entrepreneurs’ (auto)biographies. These books attempt to draw a causal link between the CEO/entrepreneur’s (a) character traits, education, and business acumen and (b) later success. The “silent evidence” (which Taleb also calls “the cemetery”) in these narratives is that there are many more people with the same attributes as the triumphant CEOs/entrepreneurs who failed. The fact is, in business, like in so many other fields, the deciding factor is nothing other than luck (i.e., randomness).
Once we become attuned to the existence of “silent evidence”—which we can think of as the “flipside” or contrary to any story we’re told—we can see it everywhere.
Bad Prediction Reason #7: Our Tendency to “Tunnel”
A repercussion of the Distortion of Silent Evidence, “tunneling” describes the natural human tendency to favor knowns and known unknowns rather than unknown unknowns. In other words, our understanding of uncertainty is based almost exclusively on what has happened in the past rather than what could have happened.
The primary practitioners of tunneling are those Taleb calls “nerds”—academics, mathematicians, engineers, statisticians, and the like. Nerds are those who think entirely “inside the box”; they Platonify the world and can’t perceive possibilities that lie outside their scientific models and academic training.
Nerds suffer from the “ludic fallacy.” (“Ludic” comes from the Latin word ludus, which means “game.”) That is, they treat uncertainty in real life like uncertainty in games of chance, for example roulette or blackjack. The problem with this approach is that, unlike games of chance, real life has no rules.
Nerds aren’t the only ones guilty of the ludic fallacy, however; average people indulge it as well. For example, most people think casino games represent the height of risk and uncertainty. In truth, casino games hail from Mediocristan—there are clear and definite rules that govern play, and the odds of winning or losing are calculable. Unlike real life, the amount of uncertainty in a casino game is highly constrained.
Bad Prediction Reason #8: Epistemic Arrogance
The reason we overestimate our ability to predict is that we’re overconfident in our knowledge.
A classic illustration of the fact comes from a study conducted by a pair of Harvard researchers. In the study, the researchers asked subjects to answer specific questions with numerical ranges. (A sample question might be, “How many redwoods are there in Redwood Park in California?” To which the subject would respond, “I’m 98% sure there are between x and y number of redwoods.) The researchers found that the subjects, though they were 98% sure of their answers, ended up being wrong 45% of the time! (Fun fact: The subjects of the study were Harvard MBAs.) In other words, the subjects picked overly narrow ranges because they overestimated they own ability to estimate. If they had picked wider ranges—and, in so doing, acknowledged their own lack of knowledge—they would have scored much better.
Taleb calls our overconfidence in our knowledge “epistemic arrogance.” On the one hand, we overestimate what we know; on the other, we underestimate what we don’t—uncertainty.
It’s important to recognize that Taleb isn’t talking about how much or how little we actually know, but rather the disparity between what we know and what we think we know. We’re arrogant because we think we know more than we actually do.
This arrogance leads us to draw a distinction between “guessing” and “predicting.” Guessing is when we attempt to fill in a nonrandom variable based on incomplete information, whereas predicting is attempting to fill in a random variable based on incomplete information.
But say that same someone asks you what the U.S. unemployment rate will be in a year. You might look at past figures, GDP growth, and other metrics to try and make a “prediction.” But the fact is, your answer will still be a “guess”—there are just too many factors (unknown unknowns) to venture anything better than a guess.
Bad Prediction Reason #9: The Curse of Information
It stands to reason that the greater our information is about a particular problem, the more likely we are to come upon a solution. And the same goes, it would seem, for predictions: The more information we have to make a prediction, the more accurate our prediction will be.
But an array of studies shows that an increase in information actually has negligible—and even negative—effects on our predictions.
For example, the psychologist Paul Slovic conducted a study on oddsmakers at horse tracks. He had the oddsmakers pick the ten most important variables for making odds, then asked the oddsmakers to create odds for a series of races using only those variables.
In the second part of the experiment, Slovic gave the oddsmakers ten more variables and asked them to predict again. The accuracy of their predictions was the same (though their confidence in their predictions increased significantly).
The negative outcome of an increase in information is that we become increasingly sure of our predictions even as their accuracy remains constant.
Other Problems with Projections
Like other predictions, projections—of incomes, costs, price fluctuations, construction time, and the like—are notoriously inaccurate.
This is because the authors of projections, like the authors of other kinds of predictions, “tunnel”—that is, they exclude from their calculations events external to whatever method they’re using.
Taleb cites the example of oil prices. In 1970, U.S. officials projected that the price of a barrel of oil would remain static or decline over the next ten years. In fact, due to crises like the Yom Kippur War and the Iranian Revolution, crude oil prices rose tenfold by 1980. (Shortform note: One can imagine those U.S. officials employing the “Different Game” and “Outlier” defenses to explain why their projection proved incorrect.)
A major problem with financial and governmental projections is that they don’t incorporate a margin of error. That is, the authors of these projections don’t take into account (nor do they publicize) how significantly their projections might be off-target.
The disregard for and omission of margins of error reveal three fallacies of projection:
Bad Prediction Reason #10: The “Final Number” Fallacy
Because corporate projections omit a margin of error, we tend to fixate on the final number of the projection, taking it as gospel—when, in fact, it obscures a (wide) range of possibilities. For example, there’s a big difference between a projected ocean temperature rise of 1ºC with a margin of error of 0.05ºC and a projection of 1ºC with a margin of error of 5ºC.
Bad Prediction Reason #11: The “Far Future” Fallacy
The further into the future one projects, the wider is that projection’s margin for error (because of the greater possibility for random occurrences), yet we treat these projections similarly to shorter-term projections. Classic examples come from literature: George Orwell’s 1984 (published in 1949), for example, was far off in terms of the state of the world in the mid-Eighties.
Bad Prediction Reason #12: The “Black Swan” Fallacy
We underestimate the randomness of the variables used in the projection—that is, we fail to understand that any part of the method used to determine the projection is susceptible to Black Swans. For an example, see the oil-price-projection example just above.
Predictors = Liars
When leveling his critiques at financial, political, and security analysts—people who make their living from forecasting—Taleb often gets asked (snippily) to propose his own predictions. In these situations, Taleb freely admits that he cannot forecast and that it would be irresponsible to attempt to.
In fact, he goes a step further: He encourages those who forecast uncritically—the “incompetent arrogants”—to get new jobs. To him, bad forecasters are either fools or liars and do more damage to society than criminals.
———End of Preview———
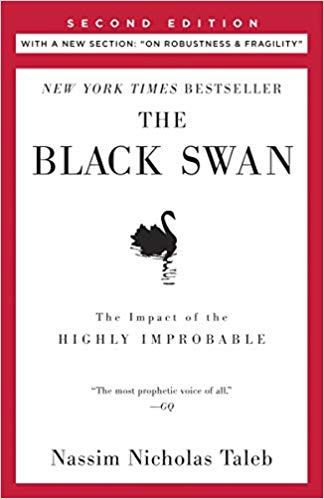
Like what you just read? Read the rest of the world's best summary of "Black Swan" at Shortform . Learn the book's critical concepts in 20 minutes or less .
Here's what you'll find in our full Black Swan summary :
- Why world-changing events are unpredictable, and how to deal with them
- Why you can't trust experts, especially the confident ones
- The best investment strategy to take advantage of black swants