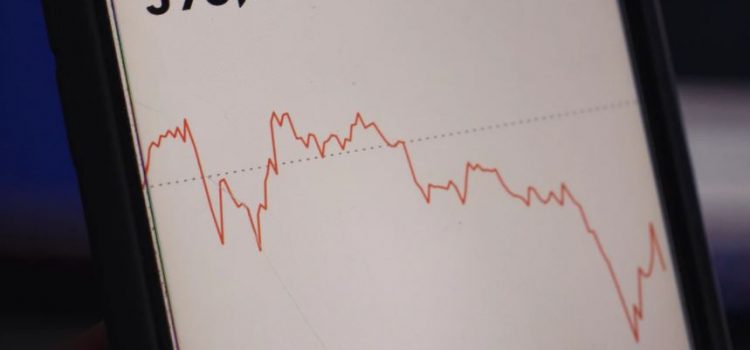
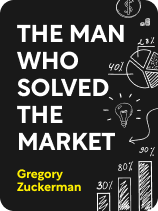
This article is an excerpt from the Shortform book guide to "The Man Who Solved the Market" by Gregory Zuckerman. Shortform has the world's best summaries and analyses of books you should be reading.
Like this article? Sign up for a free trial here.
How did Jim Simons first identify stock trend patterns that changed the market forever? What did Simons do as a codebreaker?
Jim Simons is known for his work as a mathematician who identified recognizable and consistent patterns in the financial market. But what some people might not know is that he started out decoding Soviet messages and signals during the Cold War.
Discover more about how a codebreaker used his work to identify stock trend patterns.
Identifying Stock Trends by Breaking Codes
After completing his doctoral thesis at Berkeley in 1962 and publishing his first mathematical paper that year, Simons took a job as a codebreaker with the Institute for Defense Analyses (IDA), a nonprofit research organization with close ties to the United States government. This job would be beneficial for his future work in finding stock trend patterns in the financial market.
Working there from 1964 through 1968, at the height of the Cold War between the United States and the Soviet Union, Simons’s job was to sift through reams of coded Soviet messages and signals to identify patterns. Once Simons and his intelligence analyst colleagues could identify the Soviet patterns of communication, they could unlock the meaning of those communications and gain crucial intelligence on Soviet actions—such as where they might be moving troops, diplomatic personnel, or military hardware.
(Shortform note: Coded or enciphered messages like the ones Simons and his colleagues were cracking at the IDA have existed since the earliest human civilizations. The Greek scholar Polybius devised one of history’s earliest known encryption patterns with the Polybius Square, in which each letter is represented as two numbers. This made the encrypted messages difficult to decipher for those unfamiliar with the pattern. The Roman general and statesman Julius Caesar invented another early encryption pattern by shifting the order of alphabetical characters by a set number of places, known as the Caesar Shift. Thus, if you were shifting each letter down five spaces, “F” (the sixth letter) would be written as “A” (the first letter).)
Learning to Apply Advanced Mathematics
In The Man Who Solved the Market, Gregory Zuckerman writes that the IDA was Simons’s first professional opportunity to practically apply his passion and aptitude for mathematics—an experience that was critical to his later success as an investor.
As the work was highly mathematical in nature, it played to Simons’s existing strengths and passions. But it also honed those strengths and taught him to harness those passions for practical purposes. At the IDA, he and his team applied mathematical models to construct algorithms—step-by-step processes to solve problems and perform complex tasks on a repeated basis—and perform statistical analyses to identify patterns in the coded Soviet messages.
As Zuckerman recounts, learning to recognize and identify these patterns would prove valuable later in his career, when his task was to identify minute patterns in financial and economic data. Simons and his colleagues were already exploring the possibilities of applying their intelligence codebreaking models to the world of investing. They began experimenting with purely mathematics-based market predictions, ignoring the core economic data that investors traditionally relied on—like earnings reports, company balance sheets, and published economic forecasts. Instead, they did what they already knew how to do as mathematicians and decoders: They looked at large historical data sets of financial markets and applied mathematical models to identify patterns.
Fundamental vs. Technical Analysis Simons’s approach of eschewing analysis of individual companies and stocks and instead looking at large data sets from a quantitative perspective speaks to the differences between two schools of investing thought: fundamental analysis and technical analysis. Fundamental analysis is the more traditional approach, which Simons and his IDA colleagues rejected. This school of thought attempts to measure the intrinsic value of a stock. Adherents of this philosophy seek to answer why a particular stock goes up or down in value. They do this, as the name suggests, by delving into the “fundamentals” of the company behind the stock. They’ll look at sector-wide trends and the company’s earnings, expenses, assets, and liabilities to make predictions about what the stock will do. Technical analysis, on the other hand, identifies trends and correlations—how a particular stock goes up or down in value. It’s much closer to what Simons and his colleagues were doing at the IDA and what they later did at Renaissance. Technical analysts assume that the “fundamentals” are already factored into the stock price, so it’s a waste of time and effort to analyze them. Instead, they use mathematical analysis to identify patterns and trends within the market and across different types of financial instruments (like stocks, bonds, commodities, and currencies), and these trends then signal what a stock will do in the future. |
(Shortform note: The close connections between the financial sector and the intelligence community have continued well beyond Simons’s days at the IDA in the 1960s. The CIA even allows its agents to consult for private companies on the side—a valuable policy for Wall Street firms for whom these agents’ analytical skill is enormously valuable. Defenders of this policy argue that allowing talented agents to moonlight in the private sector enables the CIA to retain top-flight talent that would otherwise jump to the corporate world—where they can earn double or triple their government salaries.)
Finding Signals in the Market Data
As Zuckerman writes, Simons and his colleagues performed their mathematics-based market predictions by analyzing historical data sets to see how prices of different financial instruments have moved. Based on the historical patterns they found in that data, they could look at the current market and gauge which condition or “state” it was in. Once they identified the state, they could identify which price movements were correlated with other price movements to make reasonable predictions about short-term price movements. In other words, in the maze of data, they could find market “signals” that would indicate what the market would do next.
For example, their model might show that under a certain condition of the market, a .25% increase in the price of wheat is historically correlated with a 3% surge in the price of sesame seeds three months later. In this case, the market signal (the increase in wheat prices) is an indication to take a certain action (buying up sesame seed futures in anticipation of the price surge in three months).
The Opportunities and Risks of Correlation Identifying patterns and correlations has become a major strategy in modern investing, as investors seek to learn how assets move in relation to each other. Correlations are measured on a scale: A perfect positive correlation between two assets is written as +1 (the two assets always move in the same direction); a perfect negative correlation is written as -1 (the two assets always move in opposite directions). Portfolio managers often use correlation to assess how diversified their assets are. As a general rule, including assets that have a low correlation to each other reduces portfolio risk: If one asset goes down in value, the uncorrelated asset is likely to go up and offset some of those losses. However, a risk of this approach is its reliance on historical data, which cannot perfectly predict future outcomes. Two previously correlated assets can become uncorrelated; likewise, two negatively correlated assets can become positively correlated, upsetting the risk balance in the portfolio. For example, under conditions of high inflation, stocks and bonds tend to have a positive correlation—their prices move in the same direction (typically downward). However, under conditions of low inflation stocks and bonds tend to have a negative correlation—when stocks rise in price, bonds fall in price, and vice versa. |
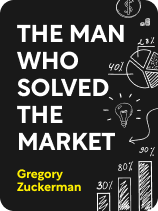
———End of Preview———
Like what you just read? Read the rest of the world's best book summary and analysis of Gregory Zuckerman's "The Man Who Solved the Market" at Shortform.
Here's what you'll find in our full The Man Who Solved the Market summary:
- The story of Jim Simons, one of the most successful hedge fund managers ever
- How Simons' experience as a codebreaker helped him succeed
- How Simons set the stage for today's large-scale, automated trading