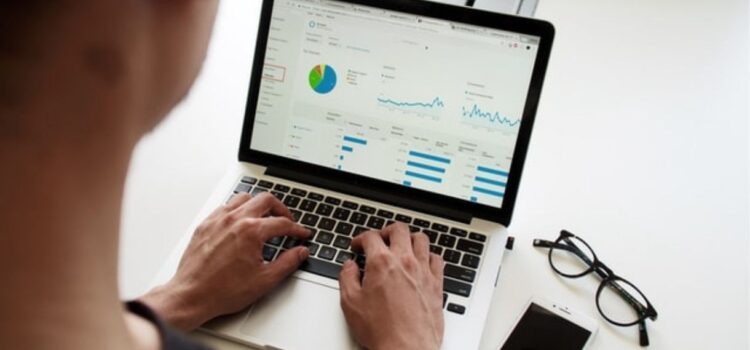
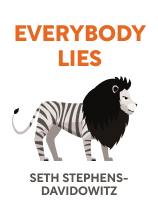
This article is an excerpt from the Shortform book guide to "Everybody Lies" by Seth Stephens-Davidowitz. Shortform has the world's best summaries and analyses of books you should be reading.
Like this article? Sign up for a free trial here .
What happens when you use data for unethical reasons? What are the drawbacks and dangers of big data?
Even though Seth Stephens-Davidowitz is openly enthusiastic about data studies, he’s aware that data has drawbacks and limitations and can lead to great harm if used unethically. In Everybody Lies, he explores some cases where these dangers have come to pass.
Read below for unethical use of data examples.
When Data Gets in the Way
Stephens-Davidowitz warns that good data science isn’t just a matter of amassing a giant data set. When working with data, he says it’s important to keep data’s shortcomings in mind and not lose sight of the bigger picture. To illustrate this, he gives unethical use of data examples.
Drawback #1: False Correlations
Stephens-Davidowitz says that when a dataset is too detailed, it can lead to predictive errors. The problem, he says, is the curse of dimensionality—a phenomenon whereby the more details a dataset contains, the more likely it is to suggest false positives when you look for predictive correlations.
Stephens-Davidowitz gives the example of flipping coins to try to predict the stock market. Say you flip a coin every day, record whether it was heads or tails, and then record whether the stock market went up or down that day. Stephens-Davidowitz says that if you perform this test using 1,000 coins for two years, it’s likely that by pure chance, at least one coin’s results will appear to correlate with market performance. Obviously this correlation is false. But Stephens-Davidowitz says this problem happens any time you test a lot of variables against a small number of outcomes—such as when trying to predict the stock market or link gene variations to disease likelihood.
(Shortform note: In addition to the risk of drawing conclusions based on random noise as Stephens-Davidowitz describes, the curse of dimensionality can make it hard to draw any meaningful conclusions at all. That happens when you classify data into so many parameters that all data points appear equidistant from each other and there are fewer “clusters” of data to draw your attention—in other words, you can no longer see useful similarities between items.)
Drawback #2: Data for Data’s Sake
Stephens-Davidowitz points out that it’s easy to fall in love with data for its own sake. When that happens, we’re likely to lose sight of what the data was supposed to be doing for us in the first place. He gives the example of standardized testing in education, which aims to make teaching and learning measurable by generating data on student outcomes.
But in many cases, schools end up focusing on improving their test scores (which are tied to schools’ reputation and funding) by any means necessary—means that include limiting the curriculum in order to focus on test prep and, in extreme cases, cheating on the tests. Stephens-Davidowitz says that studies suggest the best way to use data to measure teacher quality is to combine test scores with other factors like student evaluations and classroom observation. He says that many fields are finding that this combination of big data and traditional, small-scale information works better than focusing on big data alone.
Dangers: Exploitation and Privacy Invasion
Stephens-Davidowitz warns that an unethical use of big data can easily lend itself to exploitative practices by businesses and by governments. Check out these examples below.
Businesses Exploit Customers
While A/B testing can help businesses optimize their products and services by identifying the most effective design choices, it can also help them make their offerings more addictive. He points out that from a business perspective, a site like Facebook is ultimately designed to get you to spend more time on Facebook. The more addictive they make the site and its services, the better, and A/B testing helps them find the best ways to keep users hooked.
Similarly, Stephens-Davidowitz argues that businesses can use the doppelganger method to extract the maximum profit from their customers. He gives the example of casinos, which can use data about customers like you to predict your pain point—the point at which you lose enough money that you won’t come back to the casino for a while, if at all. Once they know your pain point, they can let you lose money until you’re approaching that point, then intervene to offer you a free dinner or other perks. They come across as generous when in reality they’re manipulating you by stopping you from gambling now so that you’ll come back sooner.
Minority Report
Finally, in the last unethical use of data example, Stephens-Davidowitz warns of the temptation to use data to make inappropriate predictions about individuals. He points to studies of loan applications that identify which words are most correlated with future defaults and which are most associated with paying back the loan. He points out that it would be unfair for a lender to use this information to deny a loan in any one particular case, based only on the words an applicant used. That’s because data can only identify statistical likelihoods, which tell us, for example, that many people who “swear to God I’ll pay back this loan” default; this insight says nothing about any specific loan applicant’s likelihood of default (whether they “swear to God” or not).
Likewise, Stephens-Davidowitz says, it may be possible to correlate, for instance, a rise in searches for racist terminology in a specific neighborhood with the likelihood of racially motivated violence in that neighborhood. He argues that it may even be prudent to use this information to allocate police resources. But he rejects the idea of acting against individuals—just because somebody’s Google search suggests an interest in committing a hate crime doesn’t mean that person will actually commit any crime.
Stephens-Davidowitz acknowledges that these lines can be fuzzy: If police know that someone has been Googling where to buy guns and ammunition, how to modify those guns to make them fully automatic, and for information on a nearby school, should they intervene directly against that person? Should they inform the school? The answers aren’t clear.
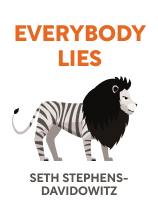
———End of Preview———
Like what you just read? Read the rest of the world's best book summary and analysis of Seth Stephens-Davidowitz's "Everybody Lies" at Shortform .
Here's what you'll find in our full Everybody Lies summary :
- How people confess their darkest secrets to Google search
- How this "big data" can be used in lieu of voluntary surveys
- The unethical uses and limitations of big data