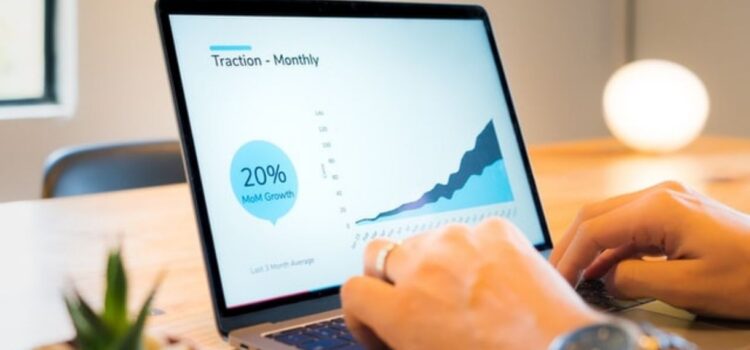
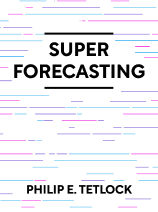
This article is an excerpt from the Shortform book guide to "Superforecasting" by Philip E. Tetlock. Shortform has the world's best summaries and analyses of books you should be reading.
Like this article? Sign up for a free trial here .
What makes a superforecaster? Are extraordinary prediction abilities a result of inborn intelligence or are they acquired through practice?
A superforecaster is a person who makes highly accurate predictions of future events, as compared to the general public or experts. According to Tetlock, superforecasters are not necessarily off-the-charts geniuses. Rather, their forecasting success comes to methodology.
Keep reading to learn about what makes a superforecaster.
What Makes a Superforecaster?
It’s reasonable to assume that superforecasters are just a group of geniuses gifted at birth with the power to see the future. Reasonable, but wrong. Superforecasters do have some unique cognitive and personality traits—like constantly seeking intellectual stimulation, or what psychologists call a “need for cognition”—but these only explain why they pursue forecasting as a hobby in the first place, not why they’re so good at it. The rest of their success comes down to methodology.
Measuring Superforecaster Intelligence
To determine the role of intelligence in superforecasters’ success, researchers needed a baseline to compare them to. Random assignment, or the random distribution of participants to different groups, is an important component of scientifically solid research. But comparing forecasters of any kind to the general population is hardly random assignment—not only are they universally intelligent and well-educated, but they also willingly volunteered to participate in the tournament for no reward other than internal fulfillment (and an Amazon gift card). To get an accurate picture, researchers needed to compare superforecasters to the general population and other forecasters.
“Intelligence” is a hard thing to measure since it takes so many forms. For this study, researchers tested forecasters on both fluid intelligence and crystallized intelligence. Fluid intelligence describes problem-solving ability, or how well you can recognize patterns in information and use them to draw conclusions. Crystallized intelligence is knowledge of facts, like “Montevideo is the capital of Uruguay” or “nine Justices sit on the United States Supreme Court.”
Overall, forecasters are a brainy group, outperforming roughly 70% of the general population on both fluid and crystallized intelligence. Superforecasters had a slight edge, outperforming 80% of the general population. In other words, superforecasters are smart (but far from genius), and one in five people in the general population can beat their scores on raw intelligence. So what makes them “super”?
Supertechnique: Fermi Estimation
In forecasting, measures of intelligence are mainly a measure of potential. What sets superforecasters apart from other forecasters is not how smart they are, but how they use that intelligence. For example, superforecasters are able to break down a complex problem, even without having all the information.
One form of this technique was popularized by Enrico Fermi, a Nobel Prize-winning Italian American physicist who also worked on the Manhattan Project. Fermi’s approach was to break down seemingly impossible questions into smaller and smaller questions. The idea is that eventually, you’ll be able to separate questions that are truly unknown from questions for which you can at least make an educated guess. Added together, a handful of educated guesses can get you remarkably close to the correct answer to the original question.
Example Fermi Problem
It’s much easier to understand this process with an example. Let’s use a classic Fermi problem: “How many piano tuners are there in the city of Chicago?” Unless you happen to be a piano tuner in Chicago, you probably have no idea how to even begin to answer this question. At best, you might take a wild guess. But Fermi’s approach is more methodical.
To answer this question, we need to determine what information we’re missing. What would we need to know to figure this out? Piano tuning is a profession, and like any profession, the number of people doing the job depends on the amount of available work divided by the amount of that work that can be done by a single person. So to figure out the number of piano tuners in Chicago, we need to know four things:
- The number of pianos in Chicago
- How frequently pianos need to be tuned
- How much time it takes to tune a single piano
- How many hours a single piano tuner needs to work to make a living
Breaking the question down like this helps us separate what we know from what we don’t. For some of these questions, we have at least enough background information to make an educated guess. For others, we may still have to take a wild guess with no context to rely on. But because those wild guesses are only for smaller parts of the overall question, we’re still likely to be more accurate than if we’d just thrown out an answer to the original question.
If we happened to know the answers to any of those four questions, great! But if we don’t, we can break them down even further into questions that we can answer. Let’s do that now.
Breaking the Piano Question Down
- The first thing we need to know is the number of pianos in Chicago. This is a difficult one, so let’s break it down further.
- The number of pianos probably depends on the number of piano players. So to start, let’s estimate the population of Chicago as a whole. We know Chicago is a big city, but it’s certainly smaller than New York and Los Angeles. If we have a rough estimate of the population of one of those cities—say, four million people—then we know Chicago has fewer than four million people (and more than zero).
- That’s still a big range, so let’s narrow it down to a range that we’re 90% sure contains the answer (this is called a confidence interval). Chicago is a major U.S. city, so let’s guess that its population is greater than 1.5 million. It’s also significantly smaller than Los Angeles, so we’ll guess there are fewer than 3.5 million people. We’ve narrowed the range from four million to two million, but that’s still too broad, so let’s use the midpoint of our confidence interval as our guess: 2.5 million people.
- So, how many of those people own a piano? Pianos are expensive, so only a small portion of the population can probably afford them. Of those who can afford them, an even smaller portion will know how to play the piano and want one in their home, so we know that only a tiny fraction of the population owns a piano. Let’s guess 1% for now.
- But it’s not just private homes that have pianos—we also find them in schools, bars, and performance spaces. In a big city, there must be at least enough of these to double our current piano ownership rate to 2%.
- So, by calculating 2% of 2.5 million people, we get an estimate of fifty thousand pianos in Chicago.
- Next, we need to know how often pianos need tuning. We don’t often hear people talking about getting their piano tuned, so it must be pretty rare—let’s say once a year.
- Now, how long does it take to tune one piano? Without more information, we can only guess. Let’s say two hours.
- Finally, how many hours does one piano tuner work in a year? Assuming piano tuning is a full-time job, the average tuner would work 40 hours per week and take two weeks of vacation per year. Working 40 hours a week, 50 weeks a year, gives us 2000 hours per year.
- But that number doesn’t account for travel time between clients. Chicago is a big city, so let’s guess that 20% of those hours are spent traveling. That gives us a total of 1600 hours a year.
- But that number doesn’t account for travel time between clients. Chicago is a big city, so let’s guess that 20% of those hours are spent traveling. That gives us a total of 1600 hours a year.
- After all that, we can finally make an educated guess at the original question. Fifty thousand pianos tuned once a year, at two hours per piano, is a total of one hundred thousand hours of piano tuning work per year. If we divide that by 1600 (the number of hours one piano tuner works per year), then there is enough work in Chicago for 62.5 piano tuners. Since we’re talking about people, let’s round that up to a final answer of 63 piano tuners in Chicago.
What’s the real answer? There are about 83 business listings for piano tuners in Chicago, but many of these are duplicates. Breaking down the question and making some careful guesses gave us an answer that is shockingly close.
Outside-In Thinking
Solving the piano tuning problem above required answering some much broader questions (like the population of Chicago). Daniel Kahneman calls that wider perspective the “outside view.” The “inside view” describes the particular details of a situation.
- For example, imagine you are given a description of a particular family and asked the likelihood of that family having a pet. If you take the inside view, you’ll focus on the unique details of that family—the number of children and parents, their financial situation, ethnicity, personalities, and so on. You’ll really be answering the question, “Are these the sort of people who are likely to have a pet?”
- By contrast, starting with the outside view means ignoring all those details and focusing on the wider context of the question. Instead of thinking about whether the family seems like the type to have a pet, you’d be answering the question “What percentage of families have a pet in general?”
By nature, our storytelling minds gravitate toward the inside view. Statistics are dry and abstract—digging into the nitty-gritty details of family relationships is much more exciting. But that natural tendency can quickly lead us astray. If we’re told that the family has three children, lives on a farm, and loves dogs, we might say it’s 90% likely they have a pet. On the other hand, if we’re told they live in a cramped city apartment and work long hours, we might swing to the other extreme and guess 10%.
The problem is that we have no way of knowing how extreme those answers are. For that, we need a base rate to give us an idea of how common it is to own a pet in general. In reality, about 62% of American households have a pet. So a guess of 90% is much closer to the mean than a guess of 10%, which means that a 10% guess is much more likely to be wrong.
Often, there are multiple outside views to consider. For example, if we know the family lives in Rhode Island (where only 45% of households have a pet), we might need to lower our base rate significantly. Choosing the most fitting outside view of the situation is important.
But why does it matter which view we start with? If we’re going to adjust our initial outside-view guess based on information from the inside view, wouldn’t the reverse give the same answer? If we were computers, maybe. But human minds are vulnerable to a psychological concept called anchoring. The number we start with has a powerful hold on us, and we tend to under-adjust in the face of new information.
For example, in the pet ownership question, if we start with an inside view guess of 10%, then move to an outside view of 45%, we’d bump our original number up, but only slightly—maybe to 15%. In this case, our anchor came out of thin air and significantly skewed the final results. But if we start with an anchor of 45% (outside view), then move to an inside view and see that the family doesn’t seem like the type to have pets, we might adjust that number down to 30%. Since most families fall relatively close to the mean, our outside-in guess is far more likely to be accurate.
Forecasting questions are rarely as simple as whether a certain family has a pet, and the inside view is often a huge, messy collection of details. In that case, looking at the whole inside view isn’t practical. We need to decide what unique aspects of the situation are important and then focus on understanding those in detail.
Putting It All Together
A superforecaster confronting a new forecasting question will take the steps above—breaking down the problem into smaller questions in Fermi style, finding the outside view and using that as an anchor, identifying what details of the situation are important, then diving into the inside view to find those details. Let’s look at an example of this process in action.
Example: Predicting Terrorism
In January of 2015, a superforecaster named David Rogg was asked to predict whether there would be a terrorist attack carried out by Islamist militants in France, the UK, Germany, the Netherlands, Denmark, Spain, Portugal, or Italy before the end of March. Terrorism is already a loaded subject, and this question was asked just days after the terrorist attack at the Charlie Hebdo office in Paris killed 12 people. In other words, resisting the impulse to start with the inside view was even more difficult than usual.
But taking an analytical approach even for emotionally charged questions is the hallmark of a superforecaster. In this case, Rogg started with the outside view by researching the context of terrorist attacks. He discovered there had been six terrorist attacks in the listed countries in the past five years, giving a base rate of 1.2 attacks per year. Notice how Rogg only counted attacks in the relevant countries, rather than researching the overall frequency of terrorist attacks worldwide. That’s an example of choosing the most relevant outside view.
Next, Rogg looked at the inside view to get a sense of how the details of this question might affect his answer. He learned that the Islamic State of Iraq and Syria (ISIS) movement had grown significantly in the previous few years and made frequent threats of terror attacks. The rise of ISIS had created a completely new political background in the previous four years, so Rogg decided to only use data from that time period, raising his base rate to 1.5 attacks per year.
But there are more inside views to consider. Europe was on high alert in the wake of the Charlie Hebdo attack, so security measures would be heightened, reducing the likelihood of another attack. On the other hand, ISIS recruitment was still on the rise. Rogg weighed these opposing factors and raised his estimate to 1.8 attacks per year. Dividing that rate into the number of days until the question expired on March 31st gave an overall prediction of a 34% chance of another terrorist attack.
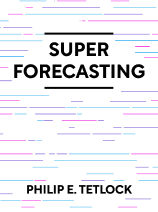
———End of Preview———
Like what you just read? Read the rest of the world's best book summary and analysis of Philip E. Tetlock's "Superforecasting" at Shortform .
Here's what you'll find in our full Superforecasting summary :
- How to make predictions with greater accuracy
- The 7 traits of superforecasters
- How Black Swan events can challenge even the best forecasters