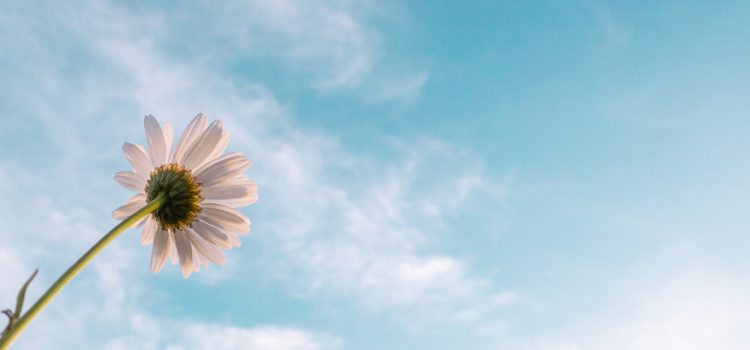
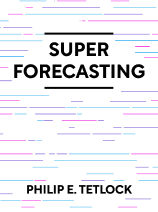
This article is an excerpt from the Shortform book guide to "Superforecasting" by Philip E. Tetlock. Shortform has the world's best summaries and analyses of books you should be reading.
Like this article? Sign up for a free trial here .
How do you measure forecast accuracy? What are some challenges in evaluating whether a forecast is correct and to what degree?
Given all the ways our brains can work against us, forecasting accurately is incredibly difficult. But evaluating an existing forecast’s accuracy in the first place presents difficulties of its own.
Read about the difficulties of measuring forecast accuracy.
Measuring Forecast Accuracy
Predictions about everything from global politics to the weather are not hard to come by. You find them on news channels, in bestselling books, and among friends and family. But most of these predictions have one thing in common: After the event, no one thinks to formally measure how accurate they were. This lack of measurement means that you have no sense of how accurate any particular source usually is. Without that baseline, how do you know who to listen to the next time you need to make a decision?
Given how important accurate predictions are, it’s surprising that we have no standard way of measuring forecast accuracy. Instead, forecasters in popular media deliver their predictions with so much confidence that we take them at their word, and by the time the events they predict happen (or don’t), the news cycle has moved on. The loudest voice is often the most convincing one, regardless of how accurate they are.
(Meteorologists are an exception to this rule. They use data to continually update weather forecasts, and they compare the actual weather to their predictions after the fact to measure forecast accuracy and get insight into what they may have missed.)
Evaluating Forecast Accuracy
Given all the ways our brains can work against us, forecasting accurately is incredibly difficult. But determining whether a forecast is accurate in the first place presents difficulties of its own. A forecast judged by different standards than the forecaster intended will be deemed a failure, even if it’s not. This is the case for one of the most famous forecasting flops of all time: the 2007 claim by Steve Ballmer, then-CEO of Microsoft, that there was “no chance” that Apple’s iPhone would get “any significant market share.”
In hindsight, this prediction looks spectacularly wrong, and it often tops lists of “Worst Tech Predictions Of All Time.” But judging Ballmer’s prediction is more difficult than it seems. What did he mean by “significant”? And was he referring to the US market or the global market? The smartphone market or the mobile phone market as a whole?
These questions matter, because the answers lead us to very different conclusions. Judged against the US smartphone market (where the iPhone commands 42% of the market share), Ballmer is laughably wrong. But in the global mobile phone market (not just smartphones), that number falls to 6%—far from significant.
Some Forecasts Are Too Vague to Judge
Although Ballmer’s infamous iPhone forecast seems clear at first, it’s actually ambiguous. The nature of language means that certain words can be interpreted differently by different people, and forecasts tend to be full of these words (like “significant,” “likely,” and “slight”). Think about a forecast that claims a particular result “may happen.” Like the doomsday prediction, there’s technically no way to discredit this forecast—if something “may” happen, it’s also implied that it may not. Either way, the forecaster is correct. But the forecast itself is useless for making decisions.
“Likely” is another word that often pops up in forecasts and presents similar problems. If a forecaster claims an event is “likely” to happen and then it doesn’t—was the forecaster wrong? Our gut reaction is to say yes, but that’s incorrect. Think of it this way: If you reach into a bag that you know contains twenty red balls and one blue ball, you could correctly claim that it’s “most likely” you’ll draw a red ball. If you happen to draw the lone blue ball, your claim is still correct—you just happened to get an unlikely result.
Lack of timelines is another common problem in popular forecasts. If someone says “the world will end tomorrow,” that has a clear end date—tomorrow, if the world has not ended, we can safely say they were wrong. But if someone says “the world will end,” any arguments to the contrary can be met with “just wait and see.” The lack of a time frame means that no matter how much time passes, they can’t be proven wrong.
Probabilities Are Useful Estimates, Not Facts
If the example of the red and blue balls brought back memories of math textbooks, there’s a reason for that. Probability is one of the biggest obstacles to judging forecast accuracy. Calculating the probability of pulling a blue ball out of a bag is fairly easy—even if you don’t know any probability formulas, you can just keep blindly pulling a ball out of the bag, recording its color, then putting it back and repeating the process. After enough trials, it would be easy to say which color ball you’re most likely to draw and about how much more likely you are to draw that color than the other.
However, attaching an accurate number to the probability of a real-world event is almost impossible. To do so, we’d need to be able to rerun history over and over again, accounting for all the different possible outcomes of a given scenario. This means that for most events that forecasters are concerned with, it is impossible to know for sure that there is a specific probability of the event happening. Therefore, any probability attached to an event in a forecast is only the forecaster’s best guess, not an objective fact. This can be misleading, but it doesn’t mean that estimated probabilities are useless.
In fact, using numerical probability estimates in forecasts is critical. In the 1950s, the CIA forecasting team discovered this after delivering a report forecasting the likelihood of the Soviet Union invading Yugoslavia. The report concluded that an attack was a “serious possibility.” When a State Department official later asked the director of the forecasting team what they meant by “serious possibility” in terms of odds, he estimated the odds at 65 to 35, much higher than how the State Department had interpreted it.
This miscommunication was understandably alarming. The director of the forecasting team, Sherman Kent, took the problem back to his team and asked them each to put a number on “serious possibility.” Though they had all collectively approved of the phrasing in the official report, every single team member assigned a different numerical value to those words. Kent was horrified: Not only were the forecasters not on the same page, but their forecasts were being used to inform foreign policy. If their reports were misunderstood, there could be global consequences.
That claim may sound dramatic, but it’s exactly what happened in 1961 when President Kennedy commissioned the Joint Chiefs of Staff to report on his plan to invade Cuba. The final report predicted a “fair chance” of success, and the government went ahead with what became the Bay of Pigs disaster. After the fact, it was clarified that “fair chance” meant three to one odds against success, but President Kennedy interpreted the phrase more positively and acted accordingly.
In the aftermath of the failed Bay of Pigs invasion, Sherman Kent proposed a universal standard for official forecasts that would eliminate ambiguity by assigning numerical probabilities to particular words. He created the chart below:
Certainty | Word |
100% | Certain |
87-99% | Almost certain |
63-86% | Probable |
40-63% | Chances about even |
20-39% | Probably not |
1-19% | Almost certainly not |
0% | Impossible |
The table above would make it difficult to misinterpret a forecast but was rejected outright by the intelligence community, who felt that expressing probabilities numerically was crude and misleading. They feared readers would fall into the common trap of interpreting numbers to mean something is X percentage likely to happen, not that the forecaster believes that to be the likelihood.
That distinction matters, since it affects not just what we do with a prediction but how we judge the person who made it. What probability percentages mean and what people think they mean are entirely different. For example, if a meteorologist correctly predicts a 70% chance of rain, it means that if we were able to replay that day hundreds of times, it would rain in 70% of those replays.
But that’s not how we typically read weather forecasts. Instead, we fall for what the authors call the “wrong-side-of-maybe fallacy,” where we interpret any prediction higher than 50% to mean something will happen and anything lower than 50% to mean it won’t. So if the meteorologist predicts a 70% chance of rain on a day where it does not rain, we think she was wrong, and consequently, that she must not be very good at her job.
In spite of those risks, meteorology has embraced the clarity of numbers, and most of us are now accustomed to seeing weather forecasts in terms of percentages. But avoiding baseless negative judgment is a major reason forecasters in other fields prefer vague language like “serious possibility.”
Using Numbers to Evaluate Forecast Accuracy
How can we measure the overall accuracy of a particular forecaster? For singular events, there really isn’t an accurate way—even with modern technology, replaying history to see every possible outcome is a power still reserved for fictional heroes like Dr. Strange. Instead, we rely on aggregates.
Let’s imagine that the meteorologist in the above example predicts the weather every day for several years, racking up hundreds of total predictions. While we still can’t say how accurate her forecast is for any specific day, we can figure out how accurate she is in general through a process called calibration.
Let’s say the meteorologist predicted a 70% chance of rain in 100 of her daily forecasts. If it actually did rain on 70 of those days, her forecasting is perfectly calibrated. In other words, a given event happens 70% of the time that she says there is a 70% chance of that event happening.
Visually, you can represent calibration with a line graph, with “forecasted percentage” on the X-axis and “percentage correct” on the Y-axis. Perfect calibration is an exact diagonal line, like on the graph below:
Obviously, most forecasters aren’t spot-on every time. The same graph setup can help us judge an individual forecaster’s accuracy by plotting each of her predictions on the graph, calculating the curved trend line for all those points, and comparing that line to the perfect diagonal line. If the forecaster is under-confident (and chronically underestimates probabilities), her curve will be far over the line; If she’s overconfident, the curve will be far under the line.
Calibration is helpful, but it’s not the only important measure for evaluating forecasts. A forecaster who always predicts probabilities near the level of chance (50%) will be fairly well-calibrated, but the information isn’t helpful—it’s the mathematical equivalent of a shrug. Stronger forecasters are accurate outside the range of chance—they’re willing to assign much higher or lower odds to a particular event, despite the increased risk of being wrong. We can measure this using resolution. Forecasters with higher resolution are more impressive than cautious forecasters who are equally well-calibrated. You can see this on the graphs below.
Interpreting Brier Scores
Combining measures of calibration and resolution gives us a concrete way to evaluate forecaster accuracy. These measures are combined into a single number, called a Brier score. Brier scores express the difference between a forecast and what really happened. Scores range between 0 and 2, where zero is an absolutely perfect forecast and two is a forecast that is wrong in every possible way. Random guessing, over time, produces a score of .5.
A forecaster’s Brier score is only meaningful in the context of the types of forecasts they make. For example, if a forecaster predicts the weather in Phoenix, Arizona to be “hot and sunny” every day for the month of June, their Brier score is likely to be almost zero, since Phoenix summers are notoriously hot and sunny. This is an impressive score but says very little about the forecaster’s skill because it took very little thoughtful consideration.
Brier scores also give us a way to compare one forecaster to another—we can say that a forecaster with an overall Brier score of .2 is a more accurate forecaster than someone with a score of .4. But context is important here, too, because Brier scores don’t account for the difficulty of each prediction. Comparing weather forecasters using their Brier scores is helpful, but it’s not fair to compare the Phoenix forecaster to a forecaster in a less stable climate like Missouri. Even if the Missouri forecaster’s score is slightly higher (and thus less accurate), earning that score in unpredictable circumstances is still much more impressive than a better score in Phoenix.
Skill vs. Luck
Brier scores measure forecast accuracy against what really happened. They’re a great way to measure the performance of individual forecasters, but there’s a caveat—they don’t rule out the possibility that someone with a stellar Brier score is just an incredibly lucky guesser. To do that, we need a way to compare forecasters’ performance to each other over time—If someone outperforms other forecasters year after year, we can confidently say their success comes down to skill; If they score above average one year and below average the next, it’s possible that initial success was just beginner’s luck.
Tracking each forecaster’s performance compared to the group reveals how much of the superforecasters’ success comes down to luck and how much is real skill. To understand the role of luck (or chance) in forecasting, we need to understand randomness. Skills and traits that are normally distributed in a population can be plotted on a classic bell curve.
- To simplify this, imagine a coin-tossing game. If 100 people were asked to predict the outcome of 100 coin tosses, the results would be normally distributed. The vast majority of guessers would be clustered in the middle of the curve, around 50%. A small group would have terrible luck and fall on the far left of the curve. Another small group would have fantastic luck and fall on the far right.
- Remember, there is no skill involved in guessing heads or tails, so those on the far right extreme of the graph are not “better guessers.” This sounds obvious when it’s spelled out, but randomness is not an intuitive concept, and studies have shown that we’re all too quick to interpret success as being a result of skill, not luck. In one study, even Yale students fell into this trap—those who had a string of correct guesses early in the coin toss game predicted they would do better than chance if the experiment were repeated. In reality, they were no more likely to beat chance than they were the first time.
Regression to the Mean
To fully understand randomness and the role of luck, we need to understand regression to the mean. With enough trials of a task, outliers will shift toward the mean. In the coin toss example, we would most likely see quick regression to the mean if the experiment were repeated multiple times. Over time, each person’s data would average out to roughly 50% correct guesses. In each repetition, there would be guessers who did extremely well or extremely poorly—but without any skill involved, the people on either extreme would be different people every time.
Regression to the mean is an invaluable tool for interpreting the IARPA tournament results. To understand this, imagine two hypothetical forecasters, Person A and Person B. In year one, Person A was a standout with 99% accuracy (and a Brier score near 0). Person B did terribly, at 1% accuracy for forecasts (giving them a Brier score close to 2.0). Their scores are plotted on the graph below.
Now let’s look at their scores in year two. If their year one performance was pure skill, we’d expect no regression at all. If their scores were half luck, half skill, we’d expect each person to regress halfway to the mean, so Person A would be at roughly 25% and Person B would be around 75%. If there is no skill involved at all (like the coin toss game), both people would likely regress back to the mean (50%) in year two. These outcomes are shown in the graphs below.
(Remember, these scores are just for year two. If each of these forecasters kept at it for decades, we would expect to see some regression—poor Person B would hopefully improve a bit with practice, and Person A’s scores would likely slip a bit over time. The key here is the rate at which this happens: Skill-based scores regress slowly, but luck-based scores regress quickly.)
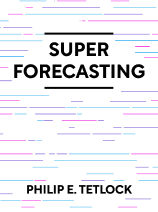
———End of Preview———
Like what you just read? Read the rest of the world's best book summary and analysis of Philip E. Tetlock's "Superforecasting" at Shortform .
Here's what you'll find in our full Superforecasting summary :
- How to make predictions with greater accuracy
- The 7 traits of superforecasters
- How Black Swan events can challenge even the best forecasters