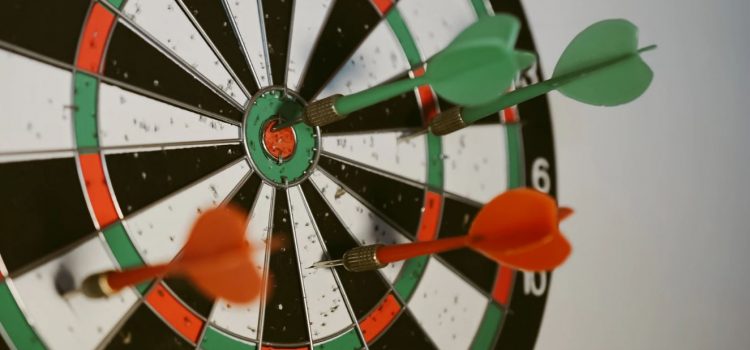
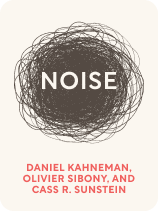
This article is an excerpt from the Shortform book guide to "Noise" by Daniel Kahneman, Olivier Sibony, and Cass Sunstein. Shortform has the world's best summaries and analyses of books you should be reading.
Like this article? Sign up for a free trial here .
What’s the difference between noise vs. bias? Which one is a worse issue when it comes to making decisions?
Noise and bias are the two main errors that occur when humans make a judgment. They are often confused, but the authors of the book Noise make a clear distinction between the two ideas.
Here’s how to understand the difference between noise and bias.
What Noise Is
The authors of Noise define noise as one of the two main errors in human judgment (the other being bias). To understand noise, we must first define judgment.
Judgment
A judgment is an attempt to mentally assign a value to something in order to choose a course of action. The authors break judgments down into predictions and evaluations.
Predictions aim to come as close as possible to some correct value or answer. The authors point out that insurance underwriters make predictions when they prepare quotes, and they’re aiming at a theoretical goldilocks number (just right). If the premium is too low, the company loses money. If the premium is too high, the company loses customers.
Likewise, doctors make predictions when they diagnose patients; they are trying to find the correct cause(s) of the patients’ ailments. The authors point out that you can measure the accuracy of a predictive judgment by comparing the prediction to the correct answer once it’s known.
Other judgments are evaluations; they have no correct answer, but instead require the decision-maker to balance pros and cons as best as possible. The authors point out that judges make evaluations when they decide how to sentence criminals, or whether to grant asylum. Similarly, teachers make evaluations when they grade essays. The authors contrast evaluative judgments with predictive judgments by pointing out that since there is no “correct” answer to an evaluative judgment, you can’t measure the accuracy of an evaluation in the same way you can with a prediction.
As a general rule, judgments are neither purely factual nor purely opinion-based. A doctor reading the results of your blood panel isn’t making a judgment (though if she sees an anomaly, she might make a judgment about its cause). Likewise, your preference for one band over another isn’t a judgment either.
Given how important professional judgments are in so many areas of our lives, we would certainly hope and expect that these judgments are accurate, consistent, and error-free. We expect a certain amount of deviation from one judger to the next and from one case to the next, because judgments take place in situations where we expect some room for disagreement among qualified, well-informed, reasonable people.
Noise vs. Bias
To improve judgments, the authors argue that we need to reduce error as much as possible by correcting for noise and bias. The authors use the following metaphor to explain noise and bias: Think of a judgment as a target at a shooting range, where inconsistency = noise and inaccuracy = bias.
- If you see a target with all the shots grouped tightly around the bullseye, you know that the shooters were both accurate and consistent (no bias, no noise).
- If you see a target with all the shots grouped tightly some distance away from the bullseye, you know the shooters were consistent but not accurate (no noise, but biased).
- If the shots are generally centered on the bullseye, but not tightly grouped, you know the shooters were generally accurate but inconsistent (not biased, but noisy).
- If you see a target with the shots spread widely and not centered on the bullseye, you know the shooters were both noisy and biased.
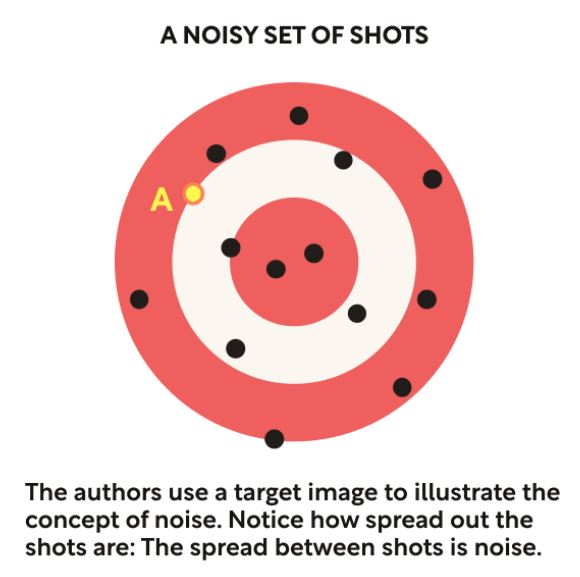
The authors make the further point that if you flip over the targets so that you can see the shots but not the bullseye, you can no longer detect bias or accuracy, but you can still easily see noise, because noise refers to the spread of the shots. That’s the difference between noise vs. bias. It means that you can detect and correct for noise without knowing the correct answer to a prediction. It also means that you can detect noise in evaluative judgments, which, as we’ve seen, are situations where there is no correct answer by which to measure the quality of the judgment. In both cases, you can also address noise without needing to know whether the judgments were biased or not. That’s because noise is the degree of inconsistency between one judgment and the next.
Don’t Mistake Low Noise for Accuracy It’s important to keep in mind that reducing noise is not a matter of improving accuracy per se—it simply means reducing variation. This is one way in which the target shooting metaphor could be misleading. We might be tempted to think that reducing noise would get us closer to the bullseye, when in fact, reducing noise only means bringing our shots closer to each other. They might still be off the mark. For example, in the image above, it’s possible that after reducing noise, all of the shots might converge around the shot marked “A” in the graphic, rather than around the bullseye as we would hope. In that case, we’d still need to improve our overall aim by reducing bias or finding other ways to be more accurate. In The Signal and the Noise, Nate Silver provides further caution against conflating noise reduction with accuracy. Silver uses a graphic almost identical to the one in Noise, but in his case, the spread of the shots represents precision—the tighter the grouping, the more precise the forecast. The problem, he says, is that when we look at forecasts, we tend to mistake precision for accuracy with sometimes devastating results—as in the 2008 financial crisis. High accuracy (in other words, low noise) creates the illusion that forecasters are on target and thereby masks both uncertainty and bias. |
While noise and bias contribute equally to overall error, the authors stress that noise is a more pertinent problem than bias because it’s less recognized and less understood. They argue that as a society, we realize that bias is an issue and try to prevent or correct for it. The same isn’t true of noise. They also suggest that noise is harder to understand than bias because it occurs on a statistical level (you need a certain number of shots before you can see how spread apart they are) which is different from how we think. We’ll explore this idea at length later.
We might be tempted to think that noise averages out over time. The authors argue that it doesn’t. They point out that if the target being aimed at is a just sentence, an accurate diagnosis, or a prudent business decision, then every miss is costly, and these costs don’t cancel each other out—they compound each other.
Is “Noise” a New Idea? The authors argue that noise, as they define it, is a new and unexplored idea. Some of Noise’s critics suggest that the book’s arguments are just a repackaged version of previous work by other authors or of common-sense ideas. To be sure, the ideas in Noise do draw on similar work from other sources. While the definition of noise as variance specific to human judgment does seem to be novel, the broader idea of noise as statistical variance isn’t. For example, Fischer Black identifies noise (which he contrasts with information) as a fundamental component of several economic models. Similarly, Nate Silver defines noise as junk data (as opposed to signal, by which he means useful information or meaningful patterns) in his treatise on how to improve our predictions. Moreover, Noise builds extensively on ideas that Kahneman previously explored in Thinking, Fast and Slow. As we’ll see, many of the underlying sources of noise trace back to errors and biases outlined in that book. In fact, we could even think of Noise as an exploration of what happens when the thinking errors from Thinking, Fast and Slow manifest on a larger scale in systems and organizations. |
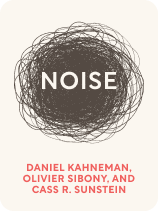
———End of Preview———
Like what you just read? Read the rest of the world's best book summary and analysis of Daniel Kahneman, Olivier Sibony, and Cass Sunstein's "Noise" at Shortform .
Here's what you'll find in our full Noise summary :
- A deep dive into the unexpected and unwanted variance in human judgments
- How to reduce or eliminate noise from your decision-making
- How to practice good decision hygiene