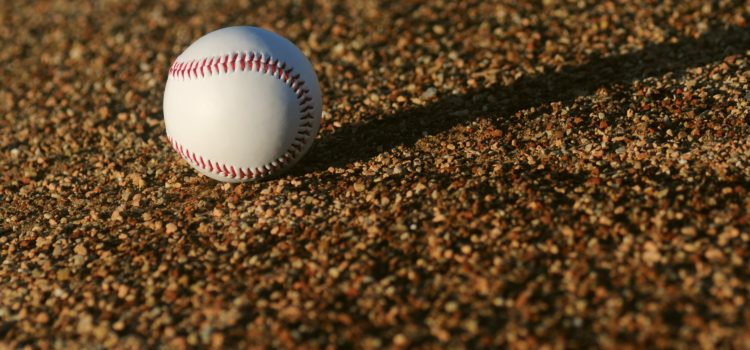
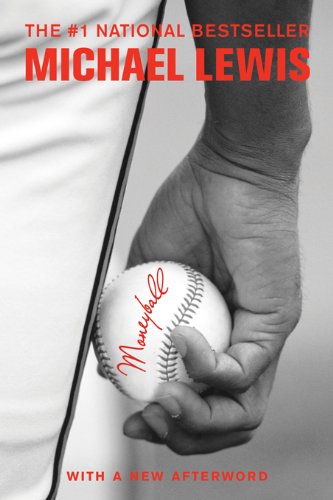
This article is an excerpt from the Shortform summary of "Moneyball" by Michael Lewis. Shortform has the world's best summaries of books you should be reading.
Like this article? Sign up for a free trial here .
Are there data analytics in baseball? What kind of baseball data do teams use to evaluate players and competition?
Data analytics in baseball is a long-standing practice. However, the type of analytics in baseball is changing rapidly as baseball adjusts to the changing business. Here’s how data analytics in baseball were changed by Moneyball and sabermetrics.
Data Analytics in Baseball: A Value-Driven Philosophy
As a cash-strapped club, the Oakland A’s adopt a practice of developing players on their own through the draft and then trading them a few years later when they become free agents, after which such players will be too expensive to retain. After the 2001 season, they lose some major stars—through trades and free agency—whose contracts they are no longer able to afford.
Despite the team’s lack of financial resources, the A’s are remarkably successful on the field under Billy’s leadership. The 2001 A’s finish 102-60. The 2002 iteration of the team goes on to a 103-59 record, good for first place in the American League West Division and second overall in the league, despite having what appears to be an inferior roster.
They achieve this through shrewd, value-driven management of their most important asset: the players. No one on the team is treated as irreplaceable. DePodesta is able to quantify the net runs each player contributes to the team (by adding runs through offense and preventing them through defense). Every action taken by a player has an expected run value.
Taking this a step further, DePodesta calculates that the team will likely need to win 95 games to make the playoffs. To win 95 games, they will need to have a net run differential of approximately +135. And this makes the team’s task a lot clearer. They need to find a combination of players whose net run production will offset the loss of Isringhausen, Damon, and Giambi, plugging in those holes to get the run differential they need. This exemplifies data analytics in baseball.
Success From Baseball Data
The team’s success is a testament to their value investment strategy in building the team and a stunning rebuke to decades of conventional wisdom about what makes a winning ballclub. Still, many baseball traditionalists, particularly former player-turned-TV-commentator Joe Morgan, appear to be actively rooting against the A’s in the playoffs. They seem to not so much disagree with Billy’s ideas about how to win baseball games as to be outright offended by them. Winning games without flashy home run sluggers or lighting-speed pitchers, to these keepers of baseball’s conventional wisdom, is a violation of the natural order of things, a perversion of the noble tradition and spirit of America’s national pastime. Morgan is part of an insider’s club of pundits, writers, scouts, and ex-players, which never seems to face accountability for its failures. They are a social club that values loyalty over competence.
Data Analytics in Baseball Examples
Billy Beane and his team used several new types of data analytics in baseball to make decisions. Here are two examples of baseball data that helped Beane find high-value players where no one else was looking.
Scott Hatteberg
Hatteberg’s style blends perfectly with Beane’s philosophy and he thrives. By the end of the 2002 season, Hatteberg is one of the stingiest swingers in the league, choosing not to swing at 64.5 percent of pitches thrown to him—the third-highest rate in the American League. DePodesta calculates that if Scott Hatteberg had taken every at bat for the A’s in 2002, the team would have scored between 940 and 950 runs, more than the entire roster of the powerhouse Yankees. Put another way, nine Scott Hattebergs are worth more than the entire Yankee team.
Chad Bradford
Chad Bradford is a pitcher who excels in the new system of data analytics in baseball, since he’s a pitcher who excels in the DIPS categories. Despite his unusual throwing style, he is a remarkably talented pitcher, one of the best on the Oakland A’s staff and one of the best relief pitchers in the major leagues. And at a meager $237,000, he is a steal. Like nearly all the other A’s pitchers, he has been overlooked or written off by the other teams, as someone who could never succeed in the major leagues. Yet he is a key part of the A’s pitching staff—the best in baseball. They’re seeing something that the rest of the league isn’t—and at a fraction of the price.
Data analytics in baseball have always been used to evaluate the game. But new baseball data changed the most fundamental aspect of the game: the players. Looking at players as value investments opens up a whole new world of data analytics in baseball.
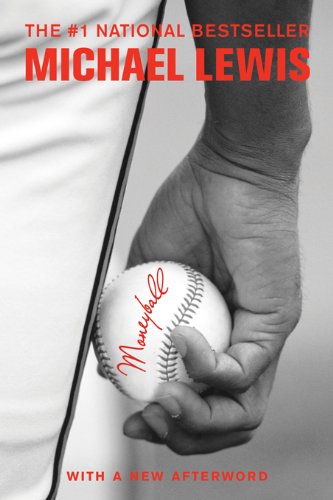
———End of Preview———
Like what you just read? Read the rest of the world's best summary of Michael Lewis's "Moneyball" at Shortform .
Here's what you'll find in our full Moneyball summary :
- How Billy Beane first flamed out as a baseball player before becoming a general manager
- The unconventional methods the Athletics used to recruit undervalued players
- How Sabermetrics influences American baseball today