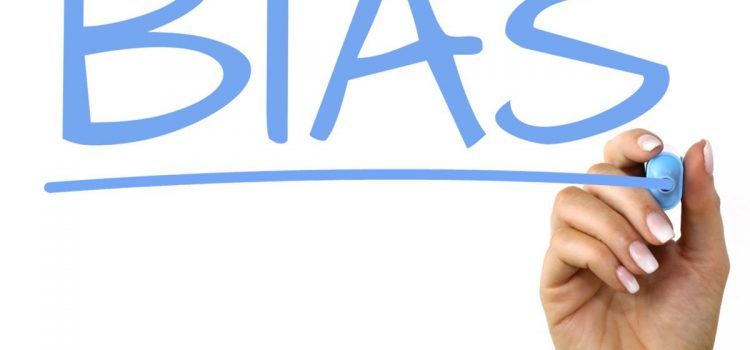
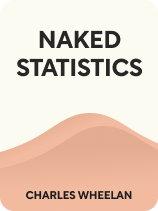
This article is an excerpt from the Shortform book guide to "Naked Statistics" by Charles Wheelan. Shortform has the world's best summaries and analyses of books you should be reading.
Like this article? Sign up for a free trial here .
What are the different types of bias in statistics? What are some ways bias can creep into a research project?
As individuals and as a society, we rely on scientific research to make informed decisions and to understand the world around us. Therefore, researchers have an ethical obligation to identify and address sources of bias in their research. Statistical bias can make its way into a research project anywhere along the way, from the study’s conception to the research question, the data collection, the statistical analysis, the reporting of findings, and the study’s publication.
Keep reading to learn about the most common sources of bias in statistics.
Sources of Bias
Biased data can sabotage otherwise sound research methods and statistical calculations. Sources of bias in data may be glaringly obvious or so subtle as to go unnoticed. If we want our data to be reliable, we should be aware of and take steps to mitigate potential sources of bias.
Reducing Bias Is a Researcher’s Responsibility As individuals and as a society, we rely on scientific research to make informed decisions and understand the world around us. Therefore, researchers have a practical and ethical obligation to identify and address sources of bias in their research. Bias can make its way into a research project anywhere along the way, from the study’s conception to the framing of the research question, the data collection, the statistical analysis, the reporting of findings, and the study’s publication. Therefore, keeping bias out of research takes effort and attention, beginning with an awareness of the myriad sources of bias, both glaringly obvious and inadvertent. |
Wheelan highlights the following types of bias in statistics:
Selection Bias
Selection bias happens when our sample is not random, and certain subsets of the population are over- or underrepresented. Selection bias can be subtle. If researchers are not cognizant of selection bias when developing data collection methods, the fact that a sample is not truly random might go unnoticed.
For example: Say you wanted to collect data on people’s political leanings before an election, and you decided to collect your data at an art show outside of town. You might think that your sample was random because the art show was a public event, the crowd was a mix of people from different parts of town, and people of all ages were represented. However, it’s likely that your data would be biased towards the opinions of wealthier residents because the people at the art show can afford the cars they used to drive out of town and the art for sale.
Selection bias can also happen when people are able to self-select into (or out of) a study. When we allow the people who feel strongly enough about a study to become the sample, our data is automatically skewed. For example, if you were to stand on the sidewalk with a banner promoting a local dog park and asked “random” people to take your survey, chances are that dog lovers strongly in favor of a dog park would be over-represented in your results, since they would take the time to come over.
Recall Bias
Recall bias happens when we ask people to give us data on the effect of a treatment or event retroactively. The challenge with obtaining reliable data from the past is that memory is not static. Wheelan explains that when we try to recall data from the past, our memory will be influenced by the meaning and emphasis our mind has placed on the event. For example, a person who fails calculus in college might be more likely to report that they “have always hated math,” even if they enjoyed math classes in high school, because their negative experience in college calculus is affecting their memory of prior classes.
Survivorship Bias
Any time a portion of a study sample is able to “leave” the study, we should be wary of survivorship bias. Wheelan explains that survivorship bias happens when our sample consists of only those who remain at the end of a “treatment” or over a significant period of time.
For example, if you were an aerobics instructor, you might be interested in improving your instruction by collecting data on what people think of your class. If you collected data at the end of a class of regular clients, all of whom have been coming to class for a long time, your sample would be biased toward a positive response. Those who did not enjoy the class would have dropped out, and therefore would not be part of the sample. By sampling a class of regulars you’d be left with “survivors” who enjoy your instruction and likely have positive things to say.
Healthy-User Bias
Samples can be biased because of the types of people who engage in the treatment we’re interested in studying. As Wheelan explains, the people who choose to engage in whichever activity or habit that we’re collecting data on are likely to be different in significant ways from people who don’t engage in the “treatment.” Therefore, isolating whether a treatment actually accounts for differences between individuals becomes challenging.
For example, say we were interested in studying the relationship between swimming and health in senior citizens. We would need to be cognizant of the fact that seniors who are swimming are likely different from their average peers outside of the pool as well. Seniors who are taking the time to swim likely take care of their health in other aspects of their life, such as their diet. Additionally, those seniors who are able to swim into old age are likely in better physical condition than their peers to begin with, hence their continued participation in sports.
Publication Bias
Publication bias has less to do with data in an individual study and more to do with the overall representation of data or an idea in public awareness. Wheelan explains that a large portion of statistics-based research is never published. This is in part, he explains, because “negative findings” don’t make for attention-grabbing headlines. For example, people might not go out of their way to read a paper entitled “Wearing Skinny Jeans Has No Impact On Your Health.”
People are more interested in attention-grabbing headlines, such as a hypothetical: “Are Your Skinny Jeans Killing You? Research Finds Link Between Skinny Jeans and Risk of Heart Attack!”
Wheelan explains that publication bias can lead to misconceptions and inflated confidence in research findings because people never get to see corresponding studies that might negate or temper published research results. For example, suppose a hypothetical study found a small positive correlation between owning a dog and lower rates of a certain type of cancer. In that case, people might rush to adopt a dog without ever knowing that five other studies found no such link.
To combat publication bias, Wheelan explains that medical journals may enforce a policy that all studies on a particular research question be reported, not just the positive ones.
Eliminating all sources of bias in a sample may not be feasible. But the more aware we are of bias in our data and the challenges of data collection, the better we’ll be able to produce reliable statistics and add context to the statistics produced by others.
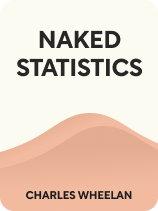
———End of Preview———
Like what you just read? Read the rest of the world's best book summary and analysis of Charles Wheelan's "Naked Statistics" at Shortform .
Here's what you'll find in our full Naked Statistics summary :
- An explanation and breakdown of statistics into digestible terms
- How statistics can inform collective decision-making
- Why learning statistics is an exercise in self-empowerment