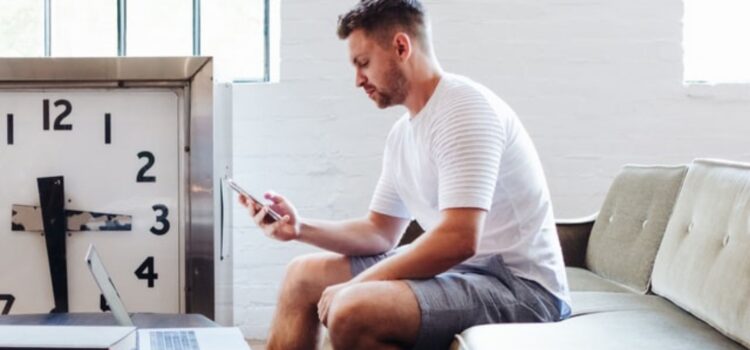
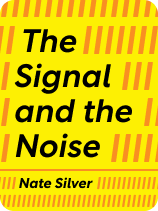
This article is an excerpt from the Shortform book guide to "The Signal and the Noise" by Nate Silver. Shortform has the world's best summaries and analyses of books you should be reading.
Like this article? Sign up for a free trial here.
Is society’s reliance on technology really all that great? How do data and technology fail to make accurate predictions?
It’s assumed that, with technological advancements, machines can help us do anything. This includes predicting the future. But, in actuality, they’re more wrong than ever.
Continue reading to learn how our reliance on technology prevents us from making correct predictions.
We Trust Too Much in Data and Technology
One of the challenges of making good predictions is a lack of information. By extension, the more information we have, our predictions should be better, at least in theory. By that reasoning, today’s technology should be a boon to predictors—we have more data than ever before, and thanks to computers, we can process that data in ways that would have been impractical or impossible in the past. However, The Signal and the Noise by Nate Silver argues that data and computers both present their own unique problems that can hinder predictions as much as they help them, and it’s all because of society’s heavy reliance on technology.
For one thing, Silver says, having more data doesn’t inherently improve our predictions. He argues that as the total amount of available data has increased, the amount of signal (the useful data) hasn’t—in other words, the proliferation of data means that there’s more noise (irrelevant or misleading data) to wade through to find the signal. At worst, this proportional increase in noise can lead to convincingly precise yet faulty predictions when forecasters inadvertently build theories that fit the noise rather than the signal.
(Shortform note: In Everybody Lies, data scientist Seth Stephens-Davidowitz goes into more detail about why too much data can actually be a bad thing. He explains that the more data points you have, the greater your chances of detecting a false signal—an apparent correlation that occurs due to chance. For example, if you flip 1,000 coins every day for two years, record the results for each coin, and record whether the stock market went up or down each day, it’s possible that through pure luck, one of the coins will appear to “predict” the stock market. And the more coins you flip, the likelier you are to find one of these “lucky” coins because more coins means more chances to generate randomness that seems predictive.)
Likewise, Silver warns that computers can lead us to baseless conclusions when we overestimate their capabilities or forget their limitations. Silver gives the example of the 1997 chess series between grandmaster Garry Kasparov and IBM’s supercomputer Deep Blue. Late in one of the matches, Deep Blue made a move that seemed to have little purpose, and afterward, Kasparov became convinced that the computer must have had far more processing and creative power than he’d thought—after all, he assumed, it must have had a good reason for the move. But in fact, Silver says, IBM eventually revealed that the move resulted from a bug: Deep Blue got stuck, so it picked a move at random.
According to Silver, Kasparov’s overestimation of Deep Blue was costly: He lost his composure and, as a result, the series. To avoid making similar errors, Silver says you should always keep in mind the respective strengths and weaknesses of humans and machines: Computers are good at brute-force calculations, which they perform consistently and accurately, and they excel at solving finite, well-defined, short- to mid-range problems (like a chess game). On the other hand, Silver says, computers aren’t flexible or creative, and they struggle with large, open-ended problems. Conversely, humans can do several things computers can’t: We can think abstractly, form high-level strategies, and see the bigger picture.
Machine Intelligence Revisited
With the proliferation of large language model (LLM) AIs such as ChatGPT and Bing Chat, computing has changed radically since The Signal and the Noise’s publication—and as a result, it’s easier than ever to forget the limitations of computer intelligence. LLMs are capable of complex and flexible behaviors such as holding long conversations, conducting research, and generating text and computer code, all in response to simple prompts given in ordinary language.
In fact, these AIs can seem so lifelike that in 2022, a Google engineer became convinced that an AI he was developing was sentient. Other experts dismissed these claims—though the engineer’s interpretation was perhaps understandable in light of interactions in which AIs have declared their humanity, professed their love for users, threatened users, and fantasized about world domination.
Given these surprisingly human-like behaviors, we might be left wondering, like Kasparov with Deep Blue, whether these programs can actually think. According to AI experts, they can’t: Though LLMs can effectively emulate human language, they do so not through a deep understanding of meaning but through an elaborate matching operation. In essence, when you enter a query, the program consults a massive database to find statistically likely words and phrases to respond with. (Some observers have likened this to the Chinese Room thought experiment, in which a person with no understanding of Chinese conducts a written exchange in that language by copying the appropriate symbols according to instructions.)
That’s why, despite AI’s impressive advances, many experts recommend that the best way forward isn’t to replace people with AI—it’s to combine the two, much as Silver recommends, in order to maximize the strengths of both. Interestingly, Kasparov himself promotes this approach, arguing that the teamwork between specific humans and specific machines is more important than the individual capabilities of each.
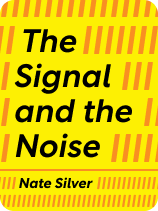
———End of Preview———
Like what you just read? Read the rest of the world's best book summary and analysis of Nate Silver's "The Signal and the Noise" at Shortform.
Here's what you'll find in our full The Signal and the Noise summary:
- Why humans are bad at making predictions
- How to overcome the mental mistakes that lead to incorrect assumptions
- How to use the Bayesian inference method to improve forecasts