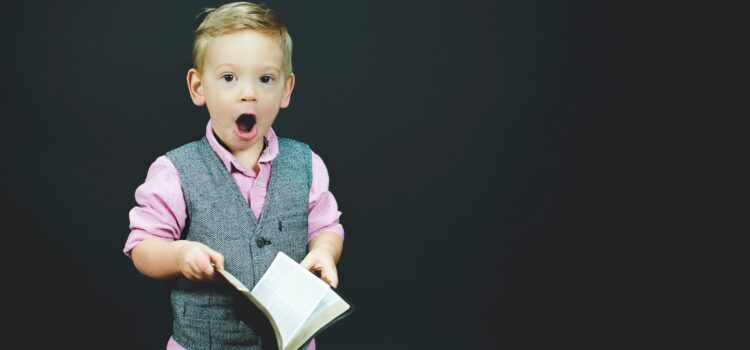
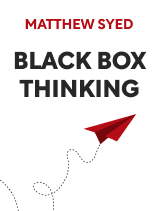
This article is an excerpt from the Shortform book guide to "Black Box Thinking" by Matthew Syed. Shortform has the world's best summaries and analyses of books you should be reading.
Like this article? Sign up for a free trial here .
What is a learning organization and why do you need one? How do you successfully build one?
A learning organization uses failures to improve its operations and innovate solutions to tough problems. According to the book Black Box Thinking, clarifying feedback, breaking down the problem, and finding opportunities to innovate are the most effective ways for you to build a learning organization.
Read on to learn about the top three techniques you can use to build a learning organization.
Technique #1: Clarify Feedback
First, clarify feedback to build a learning organization. While iteration à la the MVP is often effective, feedback is sometimes misleading. For example, if you try to test the effect of coffee on college students but give it to every test subject, you’ll have no basis on which to contextualize the results.
To combat this, Syed argues, you need to run a randomized control trial (RCT). By comparing a control group to the test group, you clarify the effect of what you’re testing.
Here’s how an RCT works: Experimenters randomly divide participants into two groups, subjecting one to the experimental variable and giving the other a placebo (or nothing). The difference between the groups’ results reveals the actual effect of the experimental variable.
For example, a learning organization developing a nootropic (brain-boosting) supplement would give the real pill to one group and a placebo pill to the other. Then they’d compare the groups’ results to determine the pill’s actual efficacy.
(Shortform note: In addition to using a placebo, researchers should conduct the trial as a double-blind. This means that neither the researchers nor the test subjects know whether they receive the treatment or the placebo. This prevents bias from skewing results: If researchers don’t know who has the treatment, their beliefs about its effectiveness can’t influence how they interpret the proceedings of the experiment.)
After testing, experimenters take the feedback and adjust their theory or product accordingly. Then they’ll repeat, keeping a tight loop between the feedback and the next iteration, until they reach a well-tested result.
Continuing the example, the nootropic company could use the data from testing to improve their product or decide whether it’s ready for the market.
(Shortform note: RCTs aren’t always the right solution, for a few reasons. If your sample size is too small (20 or fewer), the test can’t guarantee a strong effect because outliers can skew the data. Sometimes, an RCT isn’t ethical—for example, a test to determine the efficacy of an individualized tutoring program might favor some students while leaving others behind. Because it’s random, there’s no way to ensure that students with the greatest need receive help. Finally, RCTs only reveal simple causal effects: They can tell you that A → B. But real-world causation is often complex and nonlinear—for example, a pill may reduce your cholesterol while also throwing off your sleep patterns and causing hair loss.)
Technique #2: Break Down the Problem
Iteration and randomized control trials often work wonders, but some problems are too immense, too complex, or lack a viable control group. If you’re the US president, for example, you can’t run tests on the whole country—nor do you have a second country to act as a control group.
For a learning organization to handle this, Syed explains, you need to break the problem down into smaller challenges and accumulate small wins. For example, to fix a struggling economy, you’d first identify the smaller issues: runaway inflation, irresponsible financial speculators, a population crushed with debt, and so on.
Continue to chunk down the problems until you have something small enough to take action on. Then, start testing ideas. Maybe you test a free financial literacy course in one town, with a similar town as a control, instead of testing on the whole country. If it works, you can try to scale it; if not, you can adjust and try again.
Over time, many little successes accumulate into big results. Maybe the course doesn’t work until the 15th iteration, but that little success can scale and help solve the larger problem. Then move on to the next small problem, and the next, and keep building toward that larger success.
The Origin of the Black Box In 1949, a British airline called De Havilland began flying its new jet airliner, the Comet. This was the first modern passenger jet—but just a few years later, Comets began crashing. By 1954, seven Comets had disintegrated or crashed. In investigating the crashes, Australia’s Department of Civil Aviation faced a lack of meaningful data. They had no recordings of flight data—speed, positioning, fuel levels—nor any recordings of cockpit conversations, so they couldn’t deduce the cause of the crashes. One Australian researcher, David Warren, realized that if they had such recordings, they could better understand what had happened. Over the next four years, he developed a prototype black box to record flight data and the interactions between crew members in the cockpit. His invention caught the eye of British aviation authorities in 1958, and it sparked a movement to integrate black boxes into all civilian airliners. By 1962, Warren had developed a standard model, and his technology soon spread around the world. This step—from having no data on crashes to over two dozen measures of flight data and in-cockpit happenings—enabled significant improvements in airline safety. |
Technique #3: Find Opportunities to Innovate
Up to a point, you can iterate, test, and break down the problem. But eventually, your efforts will outweigh the gains from each marginal improvement. This is because surface-level iteration improves on an existing paradigm, but it doesn’t fundamentally change it.
To move further as a learning organization, Syed explains that you need to go beyond the “local maximum,” the highest point of improvement for a given paradigm. For example, you can only shoot an arrow so far with a shortbow, so you’d need to develop a new tool to surpass that distance.
(Shortform note: In Blue Ocean Strategy, W. Chan Kim argues that success comes from finding a “Blue Ocean,” or uncontested market. Syed’s “local maximum” corresponds to Kim’s “Red Ocean,” a heavily contested market. To move beyond that obvious, crowded arena, think beneath the surface-level function of products to discover the underlying function. For example, home gym equipment serves the same purpose as fitness classes or a gym membership: To help the user get fit and feel good. Knowing that, you could ask “Is there some untapped approach to home fitness that we could create?”, thus going beyond current conventional options.)
To go beyond the local maximum, you need to find and confront some unexamined problems in the current paradigm:
- To find an opportunity to innovate, consider the recurring problems with the technologies you use. For example, a medieval archer might’ve noticed that human-powered shortbows couldn’t reach distant targets.
- Then confront that problem, asking how things could be better. Maybe the archer asked, “What could propel these arrows more forcefully than human muscle?” Now he’s begun to invent the crossbow, and he did it by looking for an opportunity to change things at a deeper level.
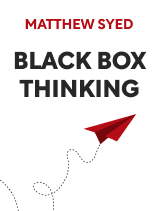
———End of Preview———
Like what you just read? Read the rest of the world's best book summary and analysis of Matthew Syed's "Black Box Thinking" at Shortform .
Here's what you'll find in our full Black Box Thinking summary :
- How an organization’s culture and systems either promote or prevent learning
- The steps for learning from failure in our complex world
- How to shift mindsets around failure to promote a learning-oriented institution