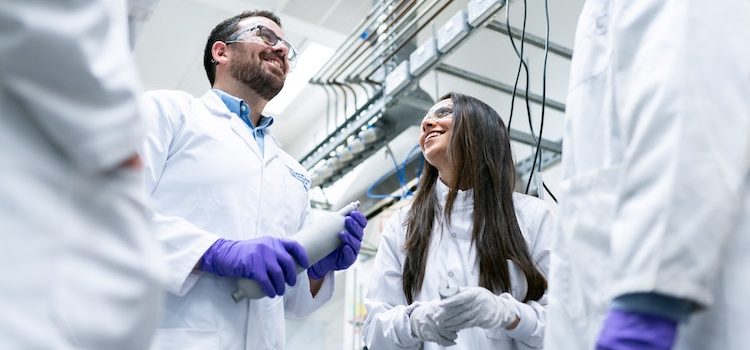
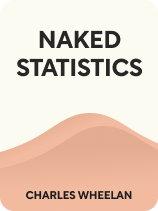
This article is an excerpt from the Shortform book guide to "Naked Statistics" by Charles Wheelan. Shortform has the world's best summaries and analyses of books you should be reading.
Like this article? Sign up for a free trial here .
What is sampling in research methodology? Why is having a representative sample important?
Many research and survey projects rely on sampling as a way to learn about a larger population. Researchers aim to select samples that reflect the target population as closely as possible.
Keep reading for the definition of a representative sample, why it’s important, and how to collect samples that are representative of the population in question.
The Importance of a Representative Sample
The definition of a representative sample is in the name: A sample that accurately represents the population in question. If the data collected in our sample doesn’t accurately represent our population, then our resulting statistics will be unreliable. There are two main ways to ensure a representative sample:
Random Sampling: A truly random sample is ideal for data collection. Random sampling allows us to be reasonably confident that we’re capturing the diversity of the underlying population because any individual has as much chance as any other of being selected. Therefore, the diversity of the sample should be close to the diversity of the population. When a sample accurately reflects the composition of its population, it’s referred to as a “representative sample.”
Large Sample Sizes: The larger our sample size, the greater the likelihood that it will represent the underlying population. This is in part because a larger sample size means more chances for the inclusion of diversity and in part because a larger sample reduces the influence of outliers. As a rule, the larger the sample, the more reliable the statistics. However, Wheelan reminds us that this rule only applies to a truly random sample. A biased sample, big or small, will produce biased statistics.
Effect Size as a Source of Bias Analysis of close to 50,000 research studies across 22 fields of science has shown that much of the bias in published research stems from the misinterpretation or misrepresentation of a study’s effect size. The effect size of a study is a measure of the difference in outcomes between the treatment and experimental groups. Statisticians argue that the effect size is just as, if not more important than the p-value (which tells us if a study is statistically significant) because a study can show very small differences between outcomes for treatment and control groups and still report statistically significant findings. As Wheelan explains, while the differences in outcomes may be mathematically significant, they may be negligible in the real world. Therefore, in addition to studying a large and random sample, one way to reduce bias in published research is to publish the effect size alongside measures of statistical significance. A famous example of this phenomenon is the five-year study of 22,000 people that resulted in the recommendation that people take aspirin to prevent heart attacks. The p-value in the study was .00001, meaning that there was a .001% chance that the observed reduction in heart attack rates while taking aspirin was due to random chance. However, the generalized recommendation that people take aspirin to prevent heart attacks has since been modified because the effect size of the study was only 0.77%, with an R2 value of .001. Since this effect size was so small, the possible side effects of taking aspirin likely outweigh the benefits for many people. |
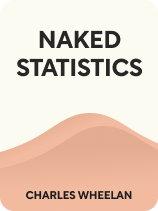
———End of Preview———
Like what you just read? Read the rest of the world's best book summary and analysis of Charles Wheelan's "Naked Statistics" at Shortform .
Here's what you'll find in our full Naked Statistics summary :
- An explanation and breakdown of statistics into digestible terms
- How statistics can inform collective decision-making
- Why learning statistics is an exercise in self-empowerment