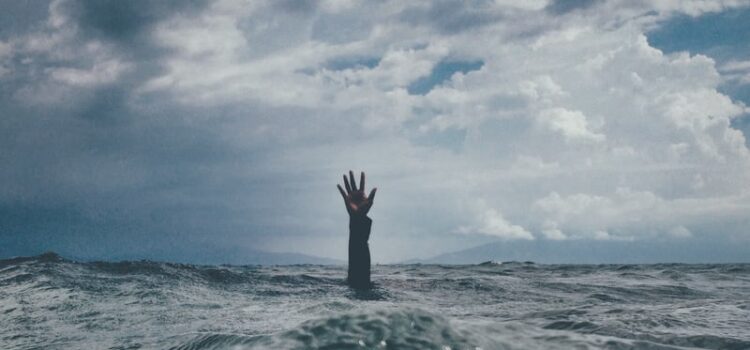
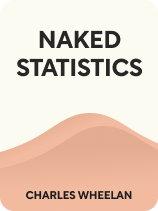
This article is an excerpt from the Shortform book guide to "Naked Statistics" by Charles Wheelan. Shortform has the world's best summaries and analyses of books you should be reading.
Like this article? Sign up for a free trial here .
What is survivorship bias in statistics? How does survivorship bias skew our interpretation of the research findings?
Any time a portion of a study sample is able to “leave” the study, we should be wary of survivorship bias. Survivorship bias occurs when we draw conclusions based on the “survivors” of a certain treatment or intervention.
Keep reading to learn about survivorship bias and how it affects research findings.
Survivorship Bias
In statistics, survivorship bias happens when our sample consists of only those who remain at the end of a “treatment” or over a significant period of time. For example, if you were an aerobics instructor, you might be interested in improving your instruction by collecting data on what people think of your class. If you collected data at the end of a class of regular clients, all of whom have been coming to class for a long time, your sample would be biased toward a positive response. Those who did not enjoy the class would have dropped out, and therefore would not be part of the sample. By sampling a class of regulars you’d be left with “survivors” who enjoy your instruction and likely have positive things to say.
Survivorship Bias and the Likelihood of Success In Fooled by Randomness, Nassim Nicholas Taleb introduces a different usage of the term “survivorship bias” with a similar consequence to the more traditional definition offered by Wheelan. As Wheelan notes, survivorship bias can make groups of people or interventions appear more “successful” than they were. For example, if you collected data on the percent of D1 collegiate soccer players who go pro, you would have a skewed perception of how many soccer players actually make it to this level, since you haven’t included all of the people who dropped out of the sport between Pee Wee soccer camp and college. Taleb offers a similar definition of the term outside the formal research context. He explains that it’s often the stories of success that “survive” their way to public attention. For example, an aspiring actress who moves to Hollywood and survives on a shoestring budget only to eventually win an Oscar would be a memorable story that people would retell. Examples of wild success like this one make their way into the mainstream and skew people’s perceived likelihood of success, making outcomes like this seem more plausible than they really are. In both cases, perceptions of the statistical likelihood of an outcome are skewed. |
Healthy-User Bias: Samples can also be biased because of the types of people who engage in the treatment we’re interested in studying. As Wheelan explains, the people who choose to engage in whichever activity or habit that we’re collecting data on are likely to be different in significant ways from people who don’t engage in the “treatment.” Therefore, isolating whether a treatment actually accounts for differences between individuals becomes challenging.
For example, say we were interested in studying the relationship between swimming and health in senior citizens. We would need to be cognizant of the fact that seniors who are swimming are likely different from their average peers outside of the pool as well. Seniors who are taking the time to swim likely take care of their health in other aspects of their life, such as their diet. Additionally, those seniors who are able to swim into old age are likely in better physical condition than their peers to begin with, hence their continued participation in sports.
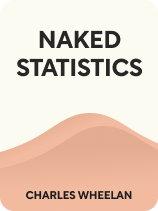
———End of Preview———
Like what you just read? Read the rest of the world's best book summary and analysis of Charles Wheelan's "Naked Statistics" at Shortform .
Here's what you'll find in our full Naked Statistics summary :
- An explanation and breakdown of statistics into digestible terms
- How statistics can inform collective decision-making
- Why learning statistics is an exercise in self-empowerment