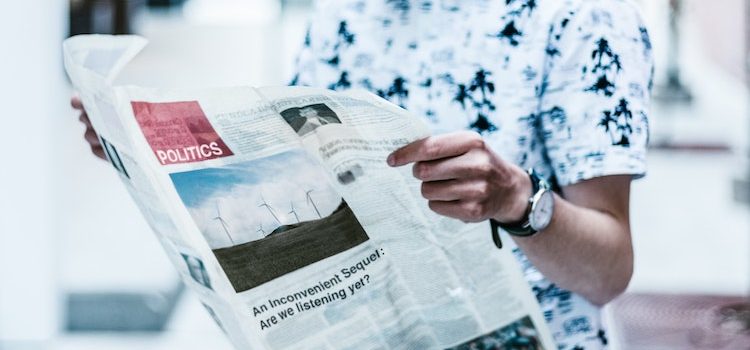
Is the institution of science immune from misinformation? How can there be lies in science when practitioners are committed to the scientific method?
In Calling Bullshit, Carl T. Bergstrom and Jevin D. West argue that science’s focus on statistical significance gives rise to bullshit for two reasons: we can easily misinterpret what statistical significance means, and we’re exposed to statistically significant findings only because of publication bias.
Let’s take a close look at both of these problematic dynamics in science.
Lies in Science
We don’t like to think that lies in science exist. But, Bergstrom and West discuss how this can happen when scientists mishandle statistical significance. They explain that a statistically significant finding is one with a certain p-value—a statistical measure of how likely it is that a study’s result happened by pure chance.
For example, imagine you wanted to see if there was a relationship between smoking cigarettes daily and getting lung cancer. You could perform a statistical analysis comparing the rates of people with lung cancer who did, and did not, smoke cigarettes daily. If you found a positive correlation between smoking and cancer and the resulting p-value of that correlation was less than 0.05, scientists would normally consider that a statistically significant result—one that is unlikely to occur from chance alone. If your analysis yielded a p-value of 0.01, that would mean there would be a 1% chance of it occurring if there weren’t a correlation between smoking and cancer.
(Shortform note: Without going too deep into the underlying mathematics, p-values are normally calculated via integral calculus on a probability distribution of all possible values, such that the p-value is identical to the percentage of the probability distribution’s area that is equal to, or more significant than, the value that you’re testing. For example, if your study between smoking and cancer established a positive correlation of 0.8, and only, say, 1% of correlations on a random distribution are 0.8 or greater, then your p-value would be equal to 0.01.)
However, Bergstrom and West point out that many people misinterpret the p-value, taking it to be the likelihood that there’s no correlation between the variables tested, which leads to bullshit when they overstate the results of statistical analyses. For example, just by sheer chance, it’s possible that if we flipped two coins 100 times, they would land on the same side 60 times, yielding a p-value of around 0.03 (in other words, there’s about a 3% chance of getting this result by pure luck). But we would be mistaken to conclude that the likelihood the two coins are connected is 0.97 because we know that barring any funny business, two simultaneous coin flips are independent events. So, we would instead be justified in concluding that the low p-value was a statistical anomaly.
The Consequences of Misrepresented P-Values The misinterpretation of p-values can have dire consequences in the courtroom, as seen in the 1998 trial of Sally Clark, a mother accused of double homicide after her first child died with no apparent cause at 11 weeks of age and her second died without cause at eight weeks. The prosecution argued that, statistically, the prior probability of two infants dying an unexplained death was one in 73 million and thus concluded that Clark had likely murdered her children. However, they failed to consider that the prior probability of a double homicide of two infants was even lower than one in 73 million, leading statisticians to later point out that it was actually more likely that the infants had suffered unexplained deaths. Although Clark was initially convicted of double homicide, she was exonerated in 2003. |
Further, Bergstrom and West contend that publication bias can promote bullshit by creating a distorted view of scientific studies. Publication bias refers to scientific journals’s tendency to only publish statistically significant results since such results are considered more interesting than non-significant results. In practice, this means published scientific studies often report statistically significant results even when these results don’t necessarily indicate a meaningful connection.
For example, even though there isn’t a connection between astrological signs and political views, if 100 studies attempted to test this relationship, we should expect about five to have a p-value below 0.05. Because these five studies would likely get published while the other 95 wouldn’t, scientific journals would inadvertently promote the bullshit view that there’s a connection between astrology and politics because of publication bias.
(Shortform note: Experts point out that, although publication bias is partially due to journals preferring to publish statistically significant results, it’s also partially due to authors often refusing to submit statistically insignificant results in the first place. For many authors, this decision is practical: Because experiments with statistically insignificant results are much more common than those with statistically significant results, submitting insignificant results could overwhelm journals with submissions.)