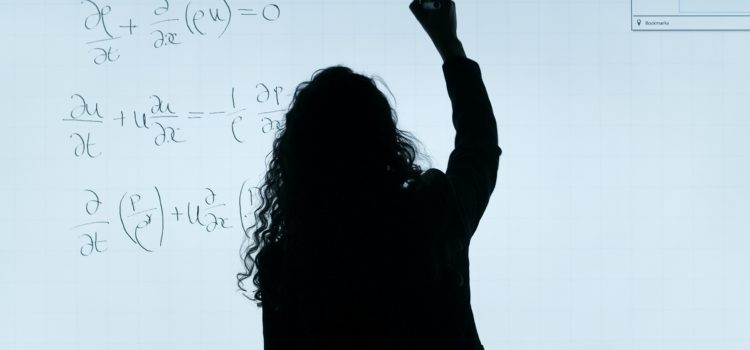
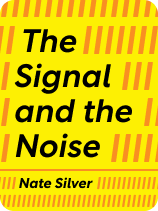
This article is an excerpt from the Shortform book guide to "The Signal and the Noise" by Nate Silver. Shortform has the world's best summaries and analyses of books you should be reading.
Like this article? Sign up for a free trial here.
Why is it important to consider all possibilities when making predictions? How has narrow-minded thinking caused catastrophic events in the past?
Nate Silver’s book The Signal and the Noise argues that, when mathematically forecasting the future, it’s important to consider the possibilities that you don’t even think would happen. Doing so at least takes these events into account, and it could prevent bad outcomes.
Here’s why every possibility should be accounted for.
Consider All Possibilities
Building on the principle of considering prior probabilities, Silver argues that it’s important to consider the possibilities that seem unlikely to happen, especially when you’re dealing with noisy data. Otherwise, you might develop blind spots that hinder your ability to predict accurately.
To illustrate this point, Silver argues that the US military’s failure to predict the Japanese attack on Pearl Harbor in 1941 shows that it’s dangerous to commit too strongly to a specific theory when there’s scant evidence for any particular theory. He explains that in the weeks before the attack, the US military noticed a sudden dropoff in intercepted radio traffic from the Japanese fleet. According to Silver, most analysts concluded that the sudden radio silence was because the fleet was out of range of US military installations—they didn’t consider the possibility of an impending attack because they believed the main threat to the US Navy was from domestic sabotage by Japanese Americans.
(Shortform note: More recent historical analysis challenges Silver’s account of US intelligence ahead of the Pearl Harbor attack. According to the National Archives, the US suspected a Japanese attack was imminent, but they misidentified the likely target. That’s because Japanese radio traffic in October and November suggested a concentration of forces in the Marshall Islands, which the US assumed meant that Japan would target the Philippines, not Hawaii. They even ordered a reconnaissance mission to gather more information about the suspected attack. As it happened, the mission was to leave from Pearl Harbor, and the aircraft chosen for the mission was still awaiting preparations when it was destroyed in the Japanese attack.)
Silver explains that one reason we sometimes fail to see all the options is that it’s common to mistake a lack of precedence for a lack of possibility. In other words, when an event is extremely uncommon or unlikely, we might conclude that it will never happen, even when logic and evidence dictate that given enough time, it will. For example, Silver points out that before the attack on Pearl Harbor, the previous foreign attack on US territory came in the early 19th century—a fact that made it easy to forget that such an attack was even possible.
How to Estimate Prior Probabilities More Accurately Silver’s Pearl Harbor example also points out the importance of generating the best possible prior estimate: Even though Bayesian logic will in theory lead you to the correct answer eventually, on a practical level, you might not find the evidence you need to get there until it’s too late. Therefore, the closer your prior estimate is to the truth, the better off you’ll be. In Algorithms to Live By, Brian Christian and Tom Griffiths offer one way to come up with better prior estimates: base your predictions on the likely distribution of the event in question. For example, if the event typically falls within a bell curve distribution (in which most values cluster around a central average), you should start with the average and then adjust. So if you’re trying to predict a student’s grade in a class, you should start with the average grade (C) then adjust based on available evidence such as the student’s study habits and prior grades. Basing your prior estimates on distributions also gives you a better chance of avoiding the precedence-possibility conflation Silver warns against. For example, if you’re trying to guess a stranger’s net worth, knowing that wealth follows a power law distribution (in which most values are clustered at one extreme with a few values at the opposite extreme) will help you remember that even if you’ve never met a billionaire before, it’s possible (though unlikely) that the stranger is one. In fact, as Christian and Griffiths explain, you’ll need to rely more heavily than usual on the available evidence when dealing with power law distributions—so if the stranger drives up in a million-dollar supercar, revise your initial estimates quickly. |
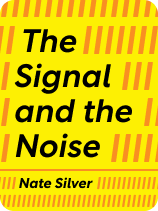
———End of Preview———
Like what you just read? Read the rest of the world's best book summary and analysis of Nate Silver's "The Signal and the Noise" at Shortform.
Here's what you'll find in our full The Signal and the Noise summary:
- Why humans are bad at making predictions
- How to overcome the mental mistakes that lead to incorrect assumptions
- How to use the Bayesian inference method to improve forecasts