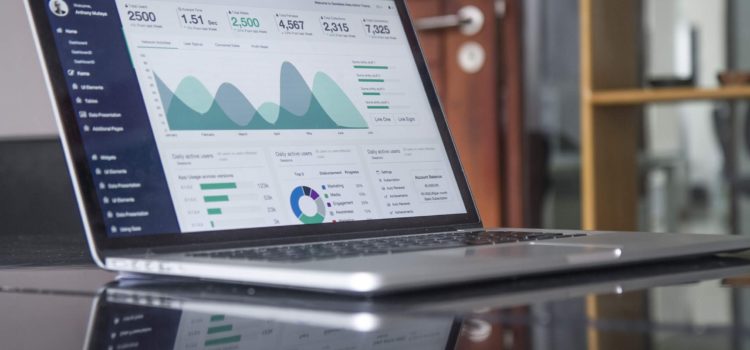
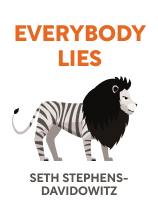
This article is an excerpt from the Shortform book guide to "Everybody Lies" by Seth Stephens-Davidowitz. Shortform has the world's best summaries and analyses of books you should be reading.
Like this article? Sign up for a free trial here .
What is the importance of big data analytics? How powerful is big data?
In Everybody Lies, Seth Stephens-Davidowitz argues that one of the powers of big data is that it allows you to zoom in on specific subsets of data. This allows new insights and new types of studies.
Learn more about how important big data is to various studies.
High-Definition Information
The ability to receive high-definition information is an example of the importance of big data analytics. Big data allows us to zoom in because providing so many data points gives our information better resolution in the same way that a high-definition display improves resolution by including more pixels.
For instance, Stephens-Davidowitz describes using Wikipedia’s database to figure out what geographical factors give you the best chance of succeeding in life. He used the database to figure out the birth county of every American notable enough to warrant a Wikipedia entry, then he cross-referenced that information with census and other data to find that the most important factors for success are proximity to a big city, proximity to a major university, and proximity to an immigrant population. His point is that a study like this is only possible because he had enough information to zoom in on individual counties and compare them across numerous factors.
(Shortform note: A study like this points to another power of big data that Stephens-Davidowitz doesn’t explicitly discuss: the ease of cross-referencing different types of information. Much of Stephens-Davidowitz’s work involves combining and comparing data from different sources to draw out new insights—even from “old” information like census data.)
The Power of Doppelgangers
Another example of big data analytics’ importance is the doppelganger method. It’s a technique where researchers make predictions about one person by studying another person who’s statistically similar to the first person.
He explains that this method was first developed by statistician and political forecaster Nate Silver, who used it to predict baseball players’ future performances. Silver realized that instead of trying to map a player’s performance onto a generic career trajectory curve, it would be better to find the past players who were statistically most similar to the player in question. These similar players are what Stephens-Davidowitz calls doppelgangers, and finding them lets you use them as a reference for your predictions. For example, if you’re trying to decide whether to keep or trade your star hitter as he nears 30 years old, you can look at his doppelgangers to see whether they kept performing or declined in their 30s.
Stephens-Davidowitz suggests that the doppelganger method could be used to improve other fields such as medicine. He argues that if we gathered and compiled enough medical data, we could find doppelgangers for each patient, and doctors could use these doppelgangers to inform their medical decisions. For example, by comparing a patient to other similar patients, a computer could flag the early symptoms of disease before they’re obvious to the doctor. He argues that a doppelganger system would also let patients find others similar to themselves to find out what treatments helped their doppelgangers.
Similar to zooming in, finding doppelgangers requires a high volume of information—you need enough people in your database to have a high likelihood of finding matches, and you need enough different data points on those people to be able to compare them meaningfully. Stephens-Davidowitz points out that the doppelganger technique—like many statistical and data science developments—started in baseball because baseball has far more comprehensive data (in terms of breadth, depth, and historical longevity) than most fields.
(Shortform note: Coincidentally, baseball also offers another example of the type of new data we saw earlier. Baseball analytics traditionally relied on players’ statistics (batting average, home runs, and so on) for insights. But recently, ballparks installed video-based tracking systems like PITCHf/x to record information like pitch velocity and spin rate, batted ball speed and trajectory, players’ running speed and ground covered, and so on. These new data types have opened up a whole new realm of performance analysis, showing that even in one of the most data-heavy industries imaginable, there are brand new types of data yet to be unearthed and studied.)
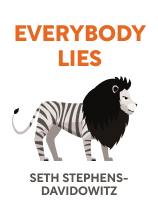
———End of Preview———
Like what you just read? Read the rest of the world's best book summary and analysis of Seth Stephens-Davidowitz's "Everybody Lies" at Shortform .
Here's what you'll find in our full Everybody Lies summary :
- How people confess their darkest secrets to Google search
- How this "big data" can be used in lieu of voluntary surveys
- The unethical uses and limitations of big data