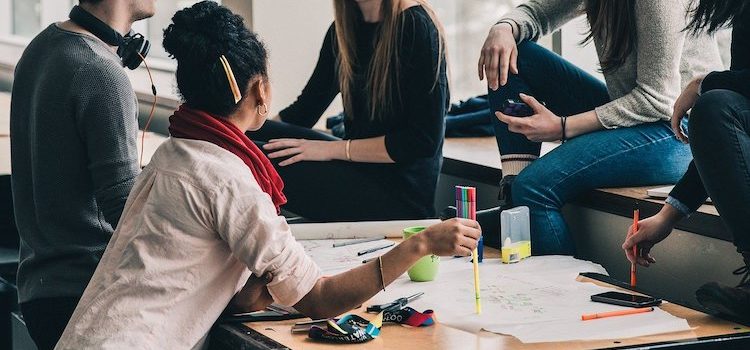
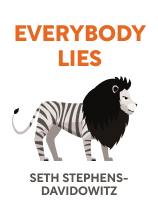
This article is an excerpt from the Shortform book guide to "Everybody Lies" by Seth Stephens-Davidowitz. Shortform has the world's best summaries and analyses of books you should be reading.
Like this article? Sign up for a free trial here .
How do cause and effect studies benefit from big data? How does big data eliminate causal research problems?
To get the most out of big data, Seth Stephens-Davidowitz says you should focus on its four benefits. In Everybody Lies, he dives into one of those benefits: easy cause-effect analysis.
Let’s look at the two ways big data makes cause-and-effect studies easier.
Easy Cause and Effect Studies
One benefit Stephens-Davidowitz says big data has is that it makes it easy to perform cause and effect studies. Scientific studies typically try to find cause-effect relationships by performing experiments that determine what impact a given variable has in a specific situation. In the social sciences, this research traditionally involves recruiting volunteers, dividing them into two or more groups, exposing some of the groups to the variable, and comparing those experimental groups to the control group.
Stephens-Davidowitz points out that this traditional experimental process requires a lot of funding, time, and other resources—and these factors limit the number of experiments researchers can do as well as the scope of those experiments. He says that big data research eliminates these problems, thereby vastly expanding the research we can do.
A/B Testing
One way big data makes causal research easier is by enabling simple A/B testing. Stephens-Davidowitz explains that an A/B test entails randomly selecting groups of users and showing each group a different version of a product or feature. For example, if a business is developing a new webpage, they might code their site so that half of their visitors see a red background and half see a blue background. They could then track each group to see how long people stayed on the page, how many links they clicked on, and whether they bought anything. By comparing the red group to the blue group, the company could determine which background color is more effective.
(Shortform note: Stephens-Davidowitz frames A/B testing as a way for data science to expand social science research, but the scientific benefits are not so clear cut. For one thing, most of the real-world applications of A/B testing seem to be limited to the corporate and political worlds—which might explain why Stephens-Davidowitz’s examples are limited to experiments with marketing and interface design. Moreover, when sites like Facebook have used A/B techniques to explore sociological questions, critics have questioned the ethics of manipulating users’ emotions or influencing their voting behavior in the name of research.)
Natural Experiments
Big data also makes cause and effect studies easier by allowing researchers to study pre-existing data (rather than running experiments to generate new data). Stephens-Davidowitz calls these kinds of studies natural experiments—a technique common in fields like economics and epidemiology where controlled experiments would be impossible or unethical. A natural experiment entails studying the results of natural processes such as disease outbreaks or market changes as though they were the results of randomized controlled experiments.
For example, Stephens-Davidowitz cites studies that examined whether attending an elite high school or college results in better outcomes than attending an ostensibly lesser school. It wouldn’t be ethical to ask schools to alter their admissions for the sake of an experiment, so the researchers instead turned to pre-existing data. To account for the fact that elite schools attract elite applicants, researchers studied two groups—students who just made it in and those who just missed the admissions cutoff or who were admitted but went to school elsewhere. The studies examined future salaries as a measure of success, and found similar results from both groups, suggesting that the schools themselves have little impact on their students’ future success.
As with zooming in and doppelganger studies, this kind of research is only possible thanks to big data’s high volume. Researchers needed to know a lot about the students in question: their application qualifications like test scores, their backgrounds, which colleges they applied to, which colleges they attended, and their career earnings.
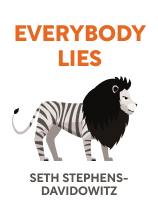
———End of Preview———
Like what you just read? Read the rest of the world's best book summary and analysis of Seth Stephens-Davidowitz's "Everybody Lies" at Shortform .
Here's what you'll find in our full Everybody Lies summary :
- How people confess their darkest secrets to Google search
- How this "big data" can be used in lieu of voluntary surveys
- The unethical uses and limitations of big data